Tag: Latvia
Baltic Shadow Economies
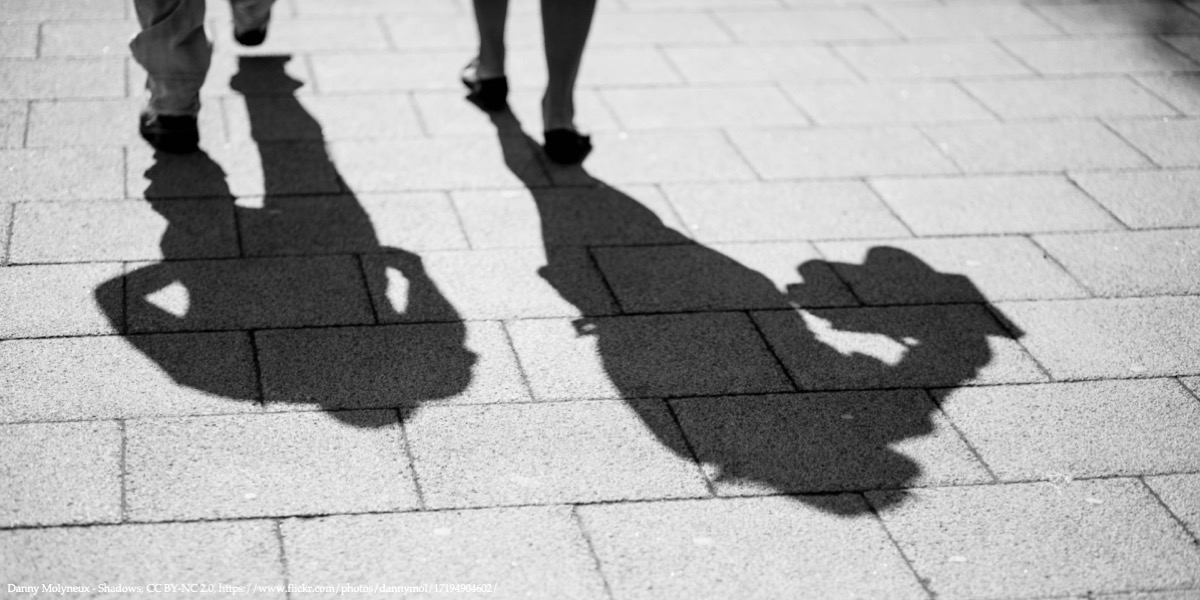
This policy brief summarises the results and implications of a recent study of the size and determinants of the shadow economies in Estonia, Latvia, and Lithuania. The results suggest that the shadow economy in Latvia in 2010 is considerably larger than in neighboring Estonia and Lithuania. While the shadow economy as a percentage of GDP in Estonia contracted from 2009 to 2010, it expanded in Latvia and Lithuania. An important driver of shadow activity in the Baltic countries is the entrepreneurs’ dissatisfaction and distrust in the government and the tax system. Involvement in the shadow economy is more pervasive among younger firms and firms in the construction sector. These findings have a number of policy implications, which are discussed at the end of this brief.
Background and Aims
Anecdotal evidence suggests that the shadow economies in the Baltic countries and other emerging Central and Eastern European countries are substantial in size relative to GDP. This is an important issue for these countries because informal production has a number of negative consequences.
First, countries can spiral into a ‘bad equilibrium’: individuals go underground to escape taxes and social welfare contributions, eroding the tax and social security bases, causing increases in tax rates and/or budget deficits, pushing more production underground and ultimately weakening the economic and social basis for collective arrangements. Second, tax evasion can also hamper economic growth by diverting resources from productive uses (producing useful goods and services) to unproductive ones (mechanisms and schemes to conceal income, monitoring of tax compliance, issuance and collection of penalties for non-compliance). Third, informal production can constrain entrepreneurs’ ability to obtain debt or equity financing for productive investment because potential creditors/investors cannot verify the true (concealed) cash flows of the entrepreneur. This can further impede growth. Finally, shadow activities distort official statistics such as GDP, which are important signals to policy makers.
The aim of our study is to measure the size of the shadow economies in Estonia, Latvia, and Lithuania, and to analyse the factors that influence participation in the shadow sector. We use the term ‘shadow economy’ to refer to all legal production of goods and services that is deliberately concealed from public authorities. The study also makes a methodological contribution by developing an index of the size of the shadow economies as a percentage of GDP. It is foreseen that the index will be published regularly.
Although an index invites comparisons, and maybe even ‘competitions’ between countries, the purpose here is not to create a ‘Baltic championship’ on shadow economies. The index should primarily be seen as a tool to promote discussion on the size and role of the shadow economy and to provide a metric which can be used to measure the degree of success in fighting the shadow economy.
Method of Measuring the Shadow Economies
Estimates the size of the shadow economies are derived from surveys of a stratified random sample of entrepreneurs in the three countries (591 in Latvia, 536 in Lithuania and 500 in Estonia). The rationale for this approach is that those most likely to know how much production or income goes unreported, are the entrepreneurs who themselves engage in the misreporting and shadow production.
Survey-based approaches face the risk of underestimating the total size of the shadow economy due to non-response and untruthful response given the sensitive nature of the topic. We minimise this risk by employing a number of surveying and data collection techniques shown in previous studies to be effective in eliciting more truthful responses (e.g., Gerxhani, 2007; Kazemier and van Eck, 1992; Hanousek and Palda, 2004).
These approaches include framing the survey as a study of satisfaction with government policy, gradually introducing the most sensitive questions after less sensitive questions, phrasing misreporting questions indirectly, e.g., asking entrepreneurs about the shadow activity among ‘firms in their industry’ rather than ‘their firm’, and, in the analysis, controlling for factors that correlate with potential untruthful response, such as tolerance towards misreporting. We aggregate entrepreneurs’ responses about misreported business income, unregistered or hidden employees, as well as unreported ‘envelope’ wages to obtain estimates of the shadow economies as a proportion of GDP.
There are three common methods of measuring GDP: the output, expenditure and income approaches. Our index is based on the income approach, which calculates GDP as the sum of gross remuneration of employees (gross personal income) and gross operating income of firms (gross corporate income). Computation of the index proceeds in three steps: (i) estimate the extent of underreporting of employee remuneration and underreporting of firms’ operating income using the survey responses; (ii) estimate each firm’s shadow production proportion as a weighted average of the two underreporting estimates with the weights reflecting the proportions of employee remuneration and firms’ operating income in the composition of GDP; and (iii) calculate a production-weighted average of shadow production across firms. Taking weighted averages of the underreporting measures rather than a simple average is important for the shadow economy index to reflect a proportion of GDP.
Size of the Shadow Economies
Table 1 indicates that the shadow economy as a proportion of GDP is considerably larger in Latvia (38.1%) compared to Estonia (19.4%) and Lithuania (18.8%) in 2010. Only Estonia has managed to marginally decrease the proportional size of its shadow economy from 2009 to 2010 – a statistically significant decrease of 0.8 percentage points. In contrast, the proportional size of the shadow economies in Lithuania and Latvia has increased by an estimated 0.8 and 1.5 percentage points, respectively.
Table 1. Shadow economy index for the Baltic countries

Note: This table reports point estimates and 95% confidence intervals for the size of the shadow economies as a proportion of GDP. The third column reports the change in the relative size of the shadow economies from 2009 to 2010.
Form of Shadow Activity
Figure 1 illustrates the average levels of underreporting (business profits, number of employees and salaries) in each of the countries in 2009 and 2010. The average levels of underreporting in all three areas are in the order of two to three times higher in Latvia compared to Lithuania and Estonia. In Latvia and Lithuania, the degree of underreporting of business profits and salaries (‘envelope’ wages) is approximately twice as large as the underreporting of employees. The exception to this trend is the relatively low amount of underreported business profits in Estonia, likely to be a result of low corporate tax rates. Bribery in Latvia and Lithuania constitutes a similar fraction of firms’ revenue, approximately 10%, whereas in Estonia bribery is less pervasive and constitutes around 6% of firms’ revenue.
Figure 1. Simple averages of underreporting and bribery among Estonian (EE), Lithuanian (LT) and Latvian (LV) firms in 2009 and 2010.

Determinants of Involvement in the Shadow Economy
The literature on tax evasion identifies two main groups of factors that affect the decision to evade taxes and thus participate in the shadow economy. The first set emerges from rational choice models of the decision to evade taxes. In such models individuals or firms weigh up the benefits of evasion in the form of tax savings against the probability of being caught and the penalties that they expect to receive if caught. Therefore the decision to underreport income and participate in the shadow economy is affected by the detection rates, the size and type of penalties, firms’ attitudes towards risk-taking and so on. These factors are likely to differ across countries, regions, sectors of the economy, size and age of firm, and entrepreneurial orientation (innovativeness, risk-taking tendencies, and pro-activeness).
Empirical studies find that the actual amount of tax evasion is considerably lower than predicted by rational choice models based on pure economic self-interest. The difference is often attributed to the second, broader, set of tax evasion determinants – attitudes and social norms. These factors include perceived justice of the tax system, i.e., attitudes about whether the tax burden and administration of the tax system are fair. They also include attitudes about how appropriately taxes are spent and how much firms trust the government. Finally, tax evasion is also influenced by social norms such as ethical values and moral convictions, as well as fear of feelings of guilt and social stigmatisation if caught.
Our study uses regression analysis to identify the factors that are statistically related to firms’ involvement in the shadow economy. The results indicate that the size of the shadow economy is smaller in Estonia and Lithuania relative to Latvia, after controlling for a range of factors.
Tolerance towards tax evasion is positively associated with the firm’s stated level of income/wage underreporting. Satisfaction with the tax system and the government is negatively associated with the firm’s involvement in the shadow economy, i.e. dissatisfied firms engage in more shadow activity, satisfied firms engage in less.
This result is consistent with previous research on tax evasion, and offers an explanation of why the size of the shadow economy is larger in Latvia than in Estonia and Lithuania; namely that Latvian firms engage in more shadow activity because they are more dissatisfied with the tax system and the government as illustrated in Figure 2. Analysing each of the four measures of satisfaction separately we find that shadow activity is most strongly related to dissatisfaction with business legislation, followed by the State Revenue Service, the government’s tax policy, and finally the government’s support for entrepreneurs.
Figure 2. Average satisfaction of firms with the tax system and government in 2010.

Note: These questions use a 5-point scale: 1=“very unsatisfied”; 2=“unsatisfied”; 3=“neither satisfied nor unsatisfied”; 4=“satisfied”; and 5=“very satisfied”. SRS is State Revenue Service.
Another strong determinant of involvement in the shadow economy is firm age, with younger firms engaging in more shadow activity than older firms. This effect dominates relations between firm size and shadow activity. A possible explanation for the relation is that young firms entering a market made up of established competitors use tax evasion as a means of being competitive in their early stages. The regression results also provide some evidence that after controlling for other factors, firms in the construction sector and firms that have a pro-active entrepreneurial orientation tend to engage in more shadow activity.
Policy Implications
First, the relatively large size of the shadow economies in the Baltic countries, and their different expansion/contraction trends, cause significant error in official estimates of GDP and its rates of change, because although statistics bureaus in each of the countries attempt to include some of the shadow production in GDP estimates they do not capture the full extent. Not only is GDP used in key policy ratios such as government deficit to GDP, debt to GDP, but also the rate of change is used as a key indicator of economic performance and therefore guides policy decisions. When the shadow economy is expanding (as in Latvia and Lithuania) official GDP growth rates underestimate true economic growth and when the shadow economy is contracting (as in Estonia) official GDP growth rates overstate true economic growth. At a minimum, policy makers need to be aware of these biases in official statistics, but ideally, statistical bureaus would implement more rigorous methods to estimate and incorporate shadow production in official statistics.
Second, our results suggest that to reduce the size of the shadow economies in the Baltic countries by encouraging voluntary compliance, a key factor that needs to be addressed is the high level of dissatisfaction with the tax system and with the government. Addressing this issue could involve actions such as making tax policy more stable (less frequent changes in procedures and tax rates), and increasing the transparency with which taxes are spent.
Finally, our estimates of the size of the shadow economies suggest that there is significant scope for all three governments to increase their revenues by bringing production ‘out of the shadows’. Investment in programs aimed at reducing the size of the shadow economies could be rather profitable for the Baltic governments, because even a small influence on entrepreneurial behaviour could result in significant revenue increases.
References
- Gerxhani, K. (2007) “‘Did you pay your taxes?’ How (not) to conduct tax evasion surveys in transition countries”, Social Indicators Research 80, pp. 555-581.
- Hanousek, J., and F. Palda (2004) “Quality of government services and the civic duty to pay taxes in the Czech and Slovak Republics, and other transition countries”, Kyklos 57(2), pp.237-252.
- Kazemier, B., and R. van Eck (1992) “Survey investigations of the hidden economy”, Journal of Economic Psychology 13, pp. 569-587.
- Schneider, F., A. Buehn, and C.E. Montenegro (2010) “Shadow economies all over the world: New estimates for 162 countries from 1999 to 2007”, World Bank Policy Research Working Paper 5356.
Disclaimer: Opinions expressed in policy briefs and other publications are those of the authors; they do not necessarily reflect those of the FREE Network and its research institutes.