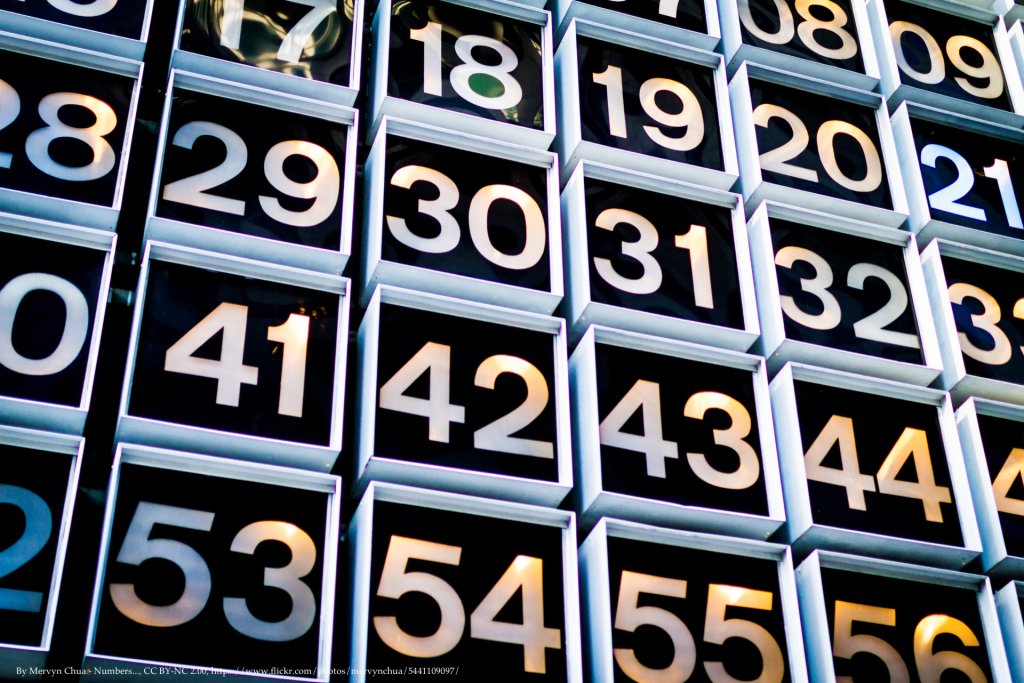
The Application of Composite Leading Indicators on the Single Economic Space Economies
This brief is based on a CEFIR research project aimed at the short-term forecasting of socio-economic development of the member-countries of the Single Economic Space (SES), conducted for the Eurasian Economic Commission in 2013. This project focused on compiling composite leading indicators that could allow policymakers to identify phases of a business cycle and to forecast its turning points. We suggest a methodology for the selection of components of the Composite Leading Indicators (CLIs) for industrial production, and apply this methodology to predict industrial production in SES member states. Our methodology performs well for Russia and Kazakhstan, and slightly less so for Belarus.
Leading economic indicators are measures of economic performance, which change before the economy is changing, i.e. before the underlying economic cycle starts to move in a particular direction. These indicators are intended to forecast where an economy is heading. Policymakers use them to implement programs and measures to avoid negative consequences of economic events to the extent possible. Most policymakers do not believe that any single indicator is reliable enough to be used as the sole guide for policy formation and responses. As a result, authorities are always looking for a variety of valuable tools for analyzing economic performance, and, in particular, short-run indicators of whether the economy is going off track. Analysts often follow composite indexes of leading indicators since trends emerging in a group of leading indicators tend to provide more reliable signals than movements of individual series.
Using leading indicators is straightforward. Investors may use leading economic indicators in order to predict both the evolution of entire economies and particular sectors within these economies, relying on established economic relationships. Furthermore, dynamics of the Composite Leading Indicators (CLIs) may reflect economic performance to the extent that they may influence expectations of likely monetary policy responses. For example, if CLIs point towards an economic slowdown, central banks may lower interest rates or implement easing measures. Thus, investors may use these indicators to help predict monetary policy.
There is a broad discussion on the use of leading economic indicators in economic forecasting, but it is mostly related to the developed economies. Morgardini and Saadi-Sedik (2003) cite three factors which account for the unpopularity of constructing leading indicators for emerging markets: the predominance of agriculture, which makes business cycle more dependent on weather changes than cyclical fluctuations in non-agricultural production processes; poor quality and frequency limitations of the data; as well as the predisposition to sudden crises and volatility in macroeconomic variables, which makes it more difficult to identify any type of economic regularity. Further, Rand and Tarp (2001) found that business cycles in emerging markets are different from those of industrial countries. In particular, an average duration of a cycle in these countries is shorter, and the speed from one stage of a cycle to another is faster. In this brief, we describe a methodology for selecting component series and compiling composite indicators that do not place any restrictions on the length of business cycle phases or a relationship between components of CLI and economic activity (usually, measured by GDP or industrial production)[1]. We will also provide some results of its application.
Methodology for Compiling CLIs
Construction of CLIs starts with the choice of the target variable, namely, the variable the indicators are supposed to lead. The most common choices of target variables indicating economic activity are gross domestic product (GDP) and industrial production. Unfortunately, GDP data in member-countries of the Single Economic Space – Belarus, Kazakhstan and Russia – is available only on a quarterly basis (except for Belarus) and with a substantial lag. Thereby, we use monthly series for industrial production, the most volatile component of economic activity, to represent «the state of the economy» in our modeling.
Once the target variable is identified, the leading indicators to predict it have to be selected. A large set of economic series available on a monthly basis was used to construct the composite leading indicators. The data sets for Russia, Belarus and Kazakhstan included series for different economic variables, such as exchange rates, monetary aggregates, price indices, budget deficit, trade flows, retail trade turnover, employment, etc. Most data for SES countries are available for only a limited historical period, which can affect the quality of the composite indices. On the other hand, one can expect improvement in the quality of CLIs as new data become available.
The selection of the components of CLIs was based on a comparison of turning points of potential component variables from the abovementioned dataset and target variable. A dating rule that identifies turning points in the series – the peaks and troughs – was based on a formal procedure defining expansion and recession terminating sequences (Harding, Pagan, 2002). Series, selected according to this procedure, i.e. components of composite index, were aggregated similarly to OECD CLIs, which aims at providing early signals of turning points in economic activity relative to its long term potential level (where long-term trend is set to be equal to 100). The OECD has developed CLIs for the Russian Federation, however, since our analysis followed a different econometric technique and was applied to different data, the component series in our composite indicator for the Russian Federation differ from those in use by the OECD.
Results: Reliability and Predictive Efficiency of CLIs
Figures 1-3 illustrate each country’s dynamics of industrial production and the composite leading indicator, which attempts to indicate turning points in economic activity three months in advance. Typically, three consecutive same-direction changes in monthly CLI suggest a turning point in the economy. Growth cycle phases are defined similarly to the OECD CLI: expansion (increase above 100 – long-term trend), downturn (decrease above 100), slowdown (decrease below 100) and recovery (increase below 100). It is worth noting that according to the OECD methodology, CLIs have the property of moving in the same direction as the business cycle, but one cannot judge the speed or strength of recovery or downturn; values of the CLI should not be interpreted as forecasts of the business cycle.
Generally, our indicators seem sufficiently reliable for policymakers to use these instruments for predicting turning points in the economic activity and the identification of phases of the economic cycle. CLIs neither miss any actual turning points in the economic activity, nor predict any extra points on top of those taking place in reality.
In Russia, the CLI continues to indicate that industrial production is pretty close to its long-term level but with some negative tendencies since the index is below the long-term level of 100. Another interesting aspect is to check the component series that were identified by the suggested methodology to enter the composite leading index to see what economic factors are important in predicting the development of Russian industrial production.
Figure 1. Composite leading indicator for Russian Industrial production, (3 month lead)
In Belarus, the CLI currently points to a positive change in momentum since mid-2012. In comparison with the Russian CLI, the indicator for Belarus is less reliable: while it successfully indicated the last trough in industrial production relative to its long-term potential level in the end of 2012, it lagged in identification of the downturn in 2008-2009.
Eight series were identified by our methodology as the component series of the Belarus CLI: the Consumer Price Index, the Producer Price Index, USD, freight turnover, the PPI for mining, the PPI for manufacturing, the Price Index for nonfood products and pipeline freight turnover. Thus, the results of the methodology for the selection of components of the CLI point to a strong dependence of Belarus production on inflation factors.
Figure 2. Composite leading indicator for Belarus Industrial production, (3 month lead)
As for Kazakhstan, the CLI is reasonably reliable in indicating the downturn followed by a recovery period.
Figure 3. Composite leading indicator for Kazakh Industrial production, (3 month lead)According to the applied methodology, the composite indicator includes seven series: the Producer Price Index, manufacturing, retail trade turnover, the actual and expected dynamics of prices of finished industrial products and the actual and expected dynamics of average prices of the main product groups in the trade sectors. Thus, the CLI points to a predominant role of manufacturing and an increase of customer demand in Kazakhstan.
Conclusion
Whether the leading indicators truly predict economic cycles has been debated. The CLIs do not measure how strongly an economy is contracting or growing; they are used instead to distinguish between phases of a business cycle. Clearly, the predictability of a leading indicator is conditioned on the quality of the economic data reported by countries, which, as is well known, does not always give the full picture but might still follow a business cycle. Our results show that, even with limitations induced by frequency of data and its quality, it is possible to establish meaningful economical and statistically significant relations between indicators from different sectors of the economy and present and future trends in the economic activity in Russia and Kazakhstan. The compiled leading indicators seem to account for established economic relationships in the economies, which can be interpreted as a sign of reasonably high predictive efficiency of our CLIs. However, one should, perhaps, be more cautious when implementing this methodology on Belarus.
▪
References
- Harding, D., Pagan, A.R. (2002) Dissecting the cycle: A methodological investigation. Journal of Monetary Economics 49(2), pp. 1365-381.
- Morgardini, J., Saadi-Sedik, T. (2003) Estimating Indexes of Coincident and Leading Indicators: An Application to Jordan. IMF Working Paper 03/170.
- Rand, J., Tarp, F. (2002) Business Cycles in Developing Countries: Are They Different?, World Development, Volume 30, Issue 12, pp. 2071-2088.
- OECD (2009), Interpreting OECD Composite Leading Indicators, OECD, 2009
- CEFIR project: Development of the principles of formation of preventive response to negative developments in the economy, based on the model complex aimed at forming the short-term (up to 1 year) forecasts of socio-economic development of the countries – members of CES
[1] Business cycle refers to the sequence of all the four stages. Higher speed of transition from one stage to the other does not necessarily means shorter cycle, while the counter usually holds true.