Tag: Labor Market
Active Labor Market Policy in the Baltic-Black Sea Region
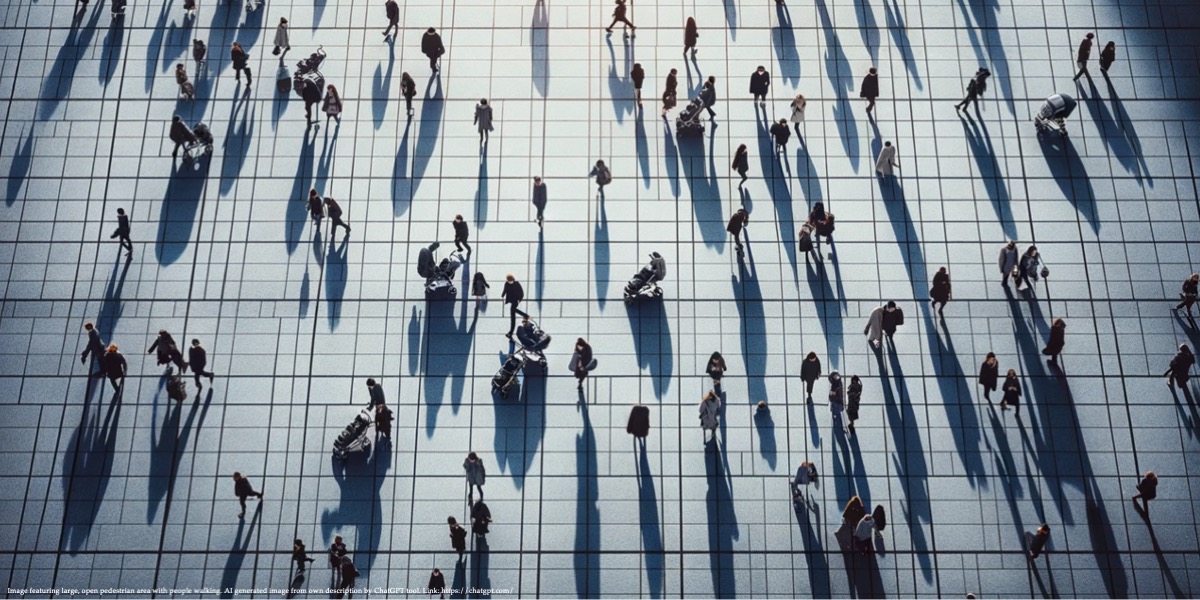
This brief outlines the characteristics of active labor market policy (ALMP) in four countries in the Baltic-Black Sea region: Belarus, Lithuania, Poland, and Ukraine. An analysis of the financing expenditure structure within this framework reveals significant differences between the countries, even for Poland and Lithuania, where the policies are to be set within a common EU framework. Countries also differed in terms of their ALMP reaction to the economic challenges brought about by the Covid-19 pandemic, as Poland and Lithuania increased their ALMP spending, while Ukraine, and, especially, Belarus, lagged behind. Despite these differences, all four countries are likely to benefit from a range of common recommendations regarding the improvement of ALMP. These include implementing evidence-informed policymaking and conducting counterfactual impact evaluations, facilitated by social partnership. Establishing quantitative benchmarks for active labor market policy expenditures and labor force coverage by active labor market measures is also advised.
Introduction
This policy brief builds on a study aimed at conducting a comparative analysis of labor market regulation policies in Belarus, Ukraine, Lithuania, and Poland. In comparing the structure of labor market policy expenditures, the aim was to identify common features between Poland and Lithuania, both of which are part of the EU and employ advanced labor market regulation approaches. We also assessed Ukraine’s policies, currently being reformed to align with EU standards, contrasting them with Belarus, where economic reforms are hindered by the post-Soviet authoritarian regime.
The analysis of the labor market policies for the considered countries is based on an evaluation of the structure of pertinent measures between 2017 and 2020 (Mazol, 2022). We used the 2015 OECD systematization of measures of active labor market policy, as presented in the first column of Table 1.
Our study reveals substantial differences in active labor market policies within the four considered countries. Still, motivated by OECD’s approach to ALMP, we provide a range of common policy recommendations that are relevant for each country included in the study. Arguably, aligning with the OECD approach would have more value for current EU and OECD members, Poland and Lithuania, and the aspiring member, Ukraine. However, these recommendations also hold value when considering a reformation of the Belarusian labor market policy.
ALMP Expenditures in Belarus, Lithuania, Poland and Ukraine
Labor market policy comprises of active and passive components. Active labor market policy involves funding employment services and providing various forms of assistance to both unemployed individuals and employers. Its primary objective is to enhance qualifications and intensify job search efforts to improve the employment prospects of the unemployed (Bredgaard, 2015). Passive labor market policy (PLMP) encompasses measures to support the incomes of involuntarily unemployed individuals, and financing for early retirement.
Poland and Lithuania are both EU and OECD members, so one would expect their labor market policies to be driven by the EU framework, and, thus, mostly aligned. However, our analysis showed that the structure of their expenditures on active labor market policies in 2017-2019 differed (Mazol, 2022). In Lithuania, the majority of the funding was allocated to employment incentives for recruitment, job maintenance, and job sharing. From 2017 to 2019, the share for these measures was between 18 and 28 percent of all expenditures for state labor market regulation. In Poland, the majority of funding was allocated to measures supporting protected employment and rehabilitation. The spending on these measures fluctuated between 23 and 34 percent of all expenditures for state labor market regulation between 2017 and 2019.
The response to the labor market challenges during the Covid-19 pandemic in Poland and Lithuania resulted in a notable surge in state labor market policy spendings in 2020, amounting to 1.78 percent of GDP and 2.83 percent of GDP, respectively. Both countries sharply increased the total spending on employment incentives (see Table 1 which summarizes the expenditure allocation for 2020). Poland experienced a nine-fold increase in costs for financing these measures (29.4 percent of total expenditures on state labor market regulation). Meanwhile, in Lithuania, financing for employment incentives increased more than tenfold, amounting to 42.5 percent of all expenditures for state labor market regulation. In both countries it became the largest active labor market policy spending area.
Table 1. Financing of state labor market measures in Baltic-Black Sea region countries in 2020 (in millions of Euro).
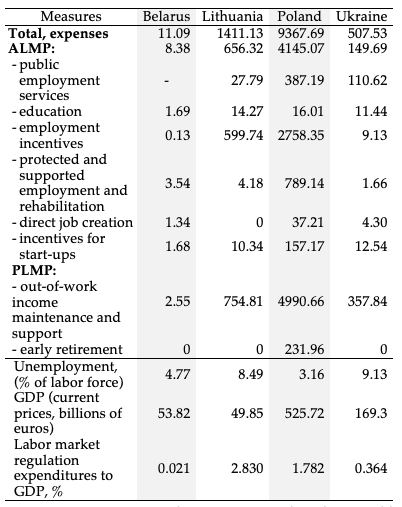
Source: DGESAI, 2023. Author’s estimations based on World Bank data (World Bank, 2023), National Bank of Belarus data, National Bank of Ukraine data.
In Ukraine, the primary focus for active labor market policy expenditures was, from 2017 to 2020, directed towards public employment services, comprising 18 to 24 percent of total labor market policy expenditures. Notably, despite the Covid-19 pandemic, there were no significant changes in either the structure or the volume of active labor market policy expenditures in Ukraine in 2020. Despite Ukraine’s active efforts to align its economic and social policies with EU standards, the government has underinvested in labor market policy, with expenditures accounting for only 0.33-0.37 percent of GDP between 2017 and 2020. This is significantly below the levels observed in Lithuania and Poland.
In Belarus, labor market policy financing is one of the last priorities for the government. In 2020, financing accounted for about 0.02 percent of GDP, amounts clearly insufficient for having a significant impact on the labor market. Moreover, Belarus stood out as the sole country in the reviewed group to have reduced its funding for labor market policies, including both active and income support measures, during the Covid-19 pandemic. The majority of the financing for labor market policy has been directed towards protected and supported employment and rehabilitation, including job creation initiatives for former prisoners, the youth and individuals with disabilities.
ALMP Improvement Recommendations
As illustrated above, the countries under review do not have a common approach to active labor market policy spendings. Further, countries like Poland and Lithuania took a more flexible stance on addressing labor market challenges caused by the Covid-19 pandemic, by implementing additional financial support for active labor market policies. However, Ukraine and Belarus did not adjust their expenditure structures accordingly. Part of these cross-country differences can be attributed to differing legal framework: Poland and Lithuania are OECD and EU member states, and, thus, subject to corresponding regulations. Ukraine is in turn motivated by the prospects of EU accession, while Belarus currently has no such prosperities to take into account.
Another important source of deviation arises from the differences in current labor market and economic conditions in the respective countries, and the governments’ need to accommodate these. While such a market-specific approach is well-justified, aligning expenditure structures with current labor market conditions necessitates obtaining updated and reliable information about the labor market situation and the effectiveness of specific labor market measures or programs. An effective labor market policy thus requires establishing a reliable system for assessing the efficiency of government measures, i.e., deploying evidence-informed policy making (OECD, 2022).
To achieve this, it is crucial to establish a robust system for monitoring and evaluating the implementation of specific measures. This involves leveraging data from various centralized sources, enhancing IT infrastructure to support data management, and utilizing modern methodologies such as counterfactual impact evaluations (OECD, 2022).
Moreover, an effective labor market regulation policy necessitates the ability to swiftly adapt existing active measures and service delivery methods in response to changes in the labor market. This might entail rapid adjustments in the legal framework, underscoring the importance of close cooperation and coordination among key stakeholders, and a well-functioning administrative structure (Lauringson and Lüske, 2021).
To accomplish this objective, it is vital to foster close collaboration between the government and institutions closely intertwined with the labor market, capable of providing essential information to labor market regulators. One of the most useful tools in this regard appears to be so-called social partnerships – a form of a dialogue between employers, employees, trade unions and public authorities, involving active information exchange and interaction (OECD, 2022).
A reliable system to assess labor market policy and in particular to facilitate their targeting, is an essential component of this approach.
Ukraine and Belarus are underfunding their labor market policies, both in comparison to the levels observed in Poland and Lithuania, and in absolute terms. It is therefore advisable to establish quantitative benchmark indicators to act as guidance for these countries, in order to ensure that any labor market policy implemented is adequately funded. Here, a reasonable approach is to align the costs of implementing labor market measures with the average annual levels for OECD countries (which are 0.5 percent of GDP for active measures and 1.63 percent for total labor market policy expenditures (OECD, 2024). Furthermore, it’s essential to ensure a high level of labor force participation in active labor market regulation measures. A target standard could be set, based on the average annual coverage from active labor market measures, at 5.8 percent of the national economy labor force, as observed in OECD countries (OECD, 2024).
Conclusion
The countries under review demonstrate varying structures of active labor market expenditures. Prior to the Covid-19 pandemic, employment incentives received the most financing in Lithuania. In Poland the largest share of expenditures was instead directed to measures to support protected employment and rehabilitation. In Ukraine, the main expenditures were directed towards financing employment services and unemployment benefits while Belarus primarily allocated funds to protected and supported employment and rehabilitation. Notably, Lithuania and Poland responded to the economic challenges following Covid-19 by significantly increasing spending on employment incentives, while Ukraine and Belarus did not undertake such measures.
Part of the diverging patterns may be attributable to the countries varying legal framework and differences in the countries respective labor market and economic conditions.
While some of the differences in labor market policies are thus justified, ensuring funding at the OECD level for labor market measures, alongside adequate tools for monitoring and evaluating labor market policies, are likely to benefit all four Baltic-Black Sea countries.
References
- Bredgaard, T. (2015). Evaluating What Works for Whom in Active Labour Market Policies. European Journal of Social Security, 17 (4), 436-452.
- DGESAI. (Directorate-General for Employment, Social Affairs and Inclusion). (2023. Expenditure by LMP intervention – country https://webgate.ec.europa.eu/empl/redisstat/databrowser/explore/all/lmp?lang=en&subtheme=lmp_expend.lmp_expend_me&display=card&sort=category&extractionId=LMP_EXPME
- Lauringson, A. and Lüske M. (2021). Institutional Set-up of Active Labour Market Policy Provision in OECD and EU Countries: Organisational Set-up, Regulation and Capacity. OECD Social, Employment and Migration Working Papers no. 262.
- Mazol, A. (2022). Active Labor Market Policy in the Countries of the Baltic-Black Sea Region. BEROC Policy Paper Series, PP no. 115.
- OECD. (2015). OECD Employment database – Labour market policies and institutions https://www.oecd.org/employment/Coverage-and-classification-of-OECD-data-2015.pdf
- OECD. (2022). Impact Evaluation of Vocational Training and Employment Subsidies for the Unemployed in Lithuania. Connecting people with jobs. Paris: OECD Publishing.
- OECD. (2024). OECDstats: Labor market programs https://stats.oecd.org
- World Bank. (2023). World Development Indicators. https://databank.worldbank.org/source/world-development-indicators
Disclaimer: Opinions expressed in policy briefs and other publications are those of the authors; they do not necessarily reflect those of the FREE Network and its research institutes.
Would a Higher Minimum Wage Meaningfully Affect Poverty Levels Among Women? – A Simulation Case from Georgia
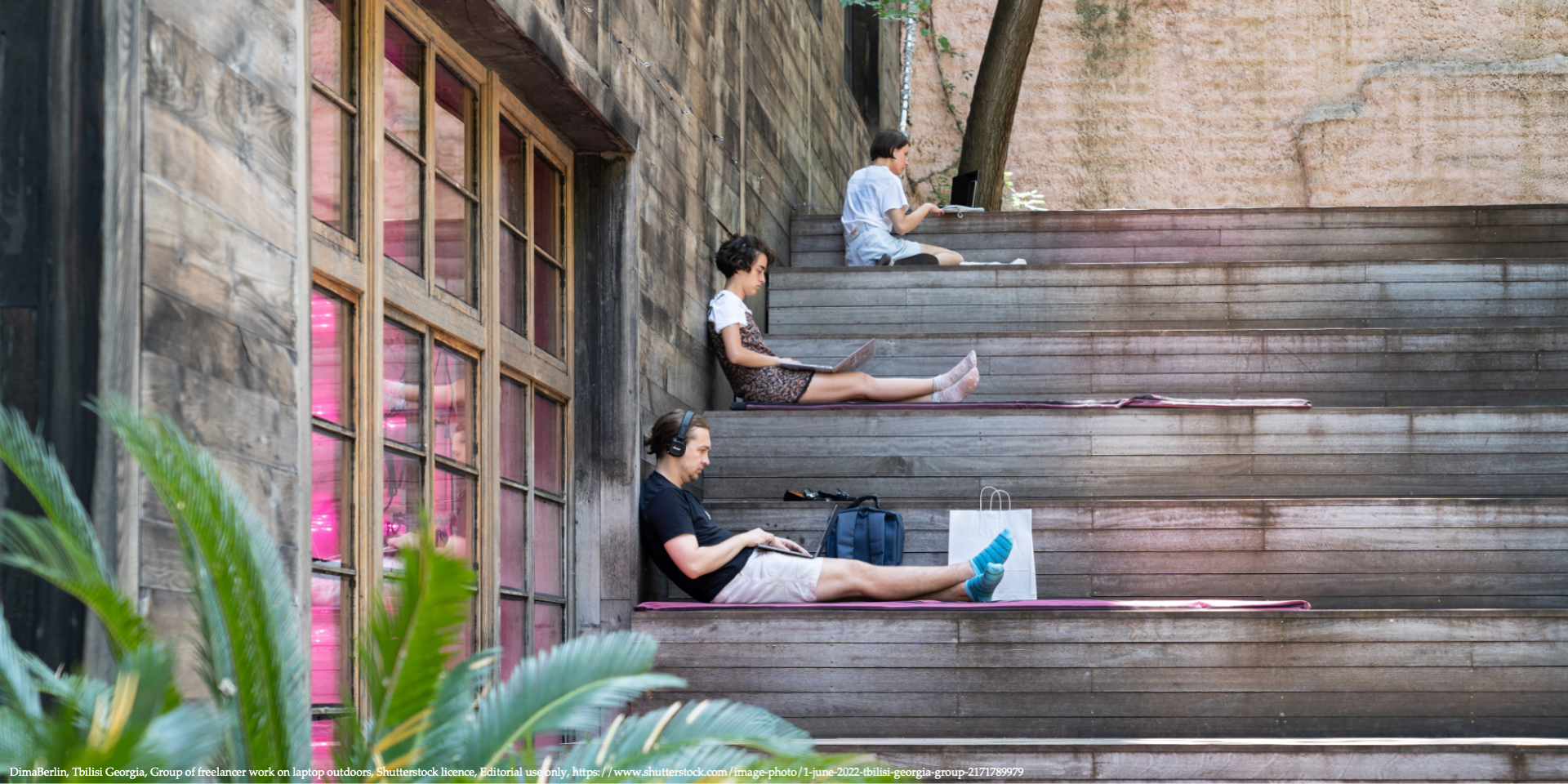
In economic literature the effect of minimum wage on the labour market and its relevance as an anti-poverty, equality-enhancing policy tool, is a matter of vigorous debate. The focus of this policy brief is a hypothetical effect on poverty rates, particularly among women, following an increase in the minimum wage in Georgia. A simulation exercise (Babych et al., 2022) by the ISET-PI research team shows that, in Georgia, a potential increase in the minimum wage is likely to result in an overall positive albeit small reduction in poverty rates in general. At the same time, women are likely to gain more from such minimum wage policy than men. The findings are consistent with the literature claiming that a minimum wage increase alone may not result in meaningful poverty reduction. Any minimum wage increase should thus be enhanced by other policies such as training programs increasing labor force participation among women.
Many countries around the world have enacted minimum wage laws. According to the International Labour Organization (ILO) “Minimum wages can be one element of a policy to overcome poverty and reduce inequality, including those between men and women” (ILO, 2023). In economic literature, the minimum wage debate has been particularly acute, with pros and cons of the minimum wage increases, their effect on the labor market, and their relevance as an anti-poverty and equality-enhancing policy tool fiercely contested in empirical studies and simulation studies. In this policy brief, we focus on the effect of a minimum wage increase in Georgia on poverty rates, and in particular poverty rates among women.
Minimum Wage Effects
According to the European Commission (2020) a number of benefits is associated with the introduction of minimum wage. These benefits include a reduction in in-work poverty, wage inequality and the gender pay gap, among others.
International evidence, however, cautions against considering an increase in minimum wage as the silver bullet to end poverty. A 2019 report by the International Labour Organization (ILO, 2019) shows that the incidence of poverty among the working poor is comparable to the incidence of poverty among individuals outside of the labor market. Therefore, even if an increase in minimum wages would lift all working poor out of poverty, a substantial number of poor would remain.
Moreover, minimum wage can have a potential adverse effect on employment of the most vulnerable by deterring firms from hiring low-wage, low-skilled labor (Neumark, 2018). The adverse employment effect will be stronger if current wages correspond more closely to the real productivity of labor. In such scenario companies would lose by retaining low-productivity workers and, likely respond to the increase in minimum wage by laying off workers, resulting in the loss of wages, rather than in their increase. On the other hand, if salaries are lower than the real productivity of the less productive workers, companies might still be able to profit from employing them and will not be forced to lay them off, resulting in a wage increase for low-wage workers.
Whether – and to what extent – the introduction of a minimum wage reduces poverty and/or assists low-income households then depends on how many individuals are going to lose their jobs, how many workers will maintain their jobs and receive a higher wage, and where these winners and losers are positioned along the distribution of family incomes.
With regard to employment effects, the results are not perfectly homogeneous. On the one hand, a large body of evidence suggests that minimum wages do lower the number of jobs accessible to low-skill employees (Sabia, Burkhauser and Hansen, 2012; Sotomayor, 2021; Neumark, 2018) On the other hand, some scholars argue that once the study design is changed to take into account the non-random distribution of minimum wage policies in different parts of the country in question, the “disemployment effect” of minimum wage policies (considering the example of United States) largely disappear (Allegretto et al., 2013; Dube et al., 2010).
With regards to poverty, a number of studies look at minimum wage as an anti-poverty policy tool for developing countries and consider its effectiveness in reducing poverty and/or inequality. For example, a study by Sotomayor (2021) suggests that poverty and income inequality in Brazil decreased by 2.8 and 2.4 percent respectively within three months of a minimum wage increase. Effects diminished with time, particularly for bottom-sensitive distribution measures, a process that is consistent with resulting job losses being more frequent among poorer households. The fact that the subsequent yearly increase in the minimum wage in Brazil resulted in a renewed drop in poverty and inequality shows that possible unemployment costs might be outweighed by benefits in the form of higher pay among working persons and – potentially – by positive spillover effects such as increased overall consumption.
Minimum Wage and Female Poverty
As in the case of poverty in general, there is some discrepancy in the literature on whether a minimum wage increase would help reduce poverty among women. Single mothers have been the focus of research in this regard since they are typically the most vulnerable low-wage workers, likely to be hurt by the loss of employment following an increase/ introduction of a minimum wage. Burkhauser and Sabia (2007) argue that the minimum wage increases in the U.S. (1988-2003) did not have any effect on the overall poverty rates, on the poverty rates among the working poor, or on poverty among single mothers. They argue that an increase in Earned Income Tax Credit (EITC), which provides a wage subsidy to workers depending on income level, tax filing status, and the number of children, would have a higher impact on poverty, in particular among single mothers.
In the meantime, Neumark and Wascher (2011) find that EITC and minimum wage reinforce each other’s positive effect for single women with children (boosting both employment and earnings), but negatively affects childless single women and minority men. Another study on the U.S. (Sabia, 2008) looked at the effect of minimum wage increases on the welfare of single mothers, finding that most of them were unaffected as they earned above-minimum wage. Single mothers with low-education levels did not see an increase in net incomes due to the negative effect on employment and hours worked: for low-skilled individuals, a 10 percent increase in minimum wage resulted in an 8.8 percent decline in employment and an 11.8 percent reduction in hours worked.
Yet another study (DeFina, 2008) focus on child poverty rates and show that minimum wage increases have a positive (reducing) impact on child poverty in female-headed families. The effect is small but significant (a 10 percent increase in the minimum wage decreases child poverty rates by 1.8 percentage points), controlling for other factors.
Ultimately, the effect of minimum wage on poverty among women or female-headed households is somewhat ambiguous. It depends on the poverty threshold used, other policy instruments (such as the EITC), existing incentives to enter employment and how, in the specific country of interest, labor laws may affect the employer’s cost of hiring (e.g. for France, see Laroque and Salanie, 2002).
The discussion is however relevant for countries like Georgia, where the wage gap between men and women is quite large, and where more women than men tend to work in low-wage and vulnerable jobs. While the overall poverty gap between men and women in Georgia is insignificant (mainly because poverty is measured at the household level), the gap becomes apparent when comparing female-headed households to male-headed ones. The poverty rates in the former case are nearly 2 percentage points higher in Georgia (20 percent vs. 18.3 percent in 2021). The poverty rates are the highest among households with only adult women (39.3 percent for all-female households vs. 20.1 percent overall in 2018).
A Simulation of a Minimum Wage Raise in Georgia
The Georgian minimum wage legislation dates back to 1999. The presidential decree N 351 from June 4, 1999 states that the minimum (monthly) wage that is to be set in Georgia is equal to 20 GEL (with some specific exceptions in the public sector). This is a non-binding threshold. Therefore, one has to think carefully what consequences might arise from raising the minimum wage to a much higher level. In addition to previously discussed aspects, one issue to keep in mind is the different average wages across different regions in Georgia. For example, a national minimum wage increase might have more of an impact in poorer regions, where both wages and incomes are lower, while it may still be non-binding in Tbilisi.
The ISET-PI research team (Babych et al., 2022) use Georgian micro data from the Labor Force Survey (LFS) and the Household Integrated Expenditure Survey (HIES), to simulate the effect of instituting a nation-wide minimum wage on both employment and poverty rates in different regions of Georgia. One focus area of the study was to analyze the effects of a minimum wage increase on female poverty. As with any exercise using a simulation approach, this study is subject to limitations imposed by the assumptions used, e.g. how much labor demand would respond to changes in the minimum wage, etc. The study considered two hypothetical thresholds of the minimum wage; 250 and 350 GEL respectively.
Figure 1. Share of private sector employees earning below certain thresholds, by gender, 2021.
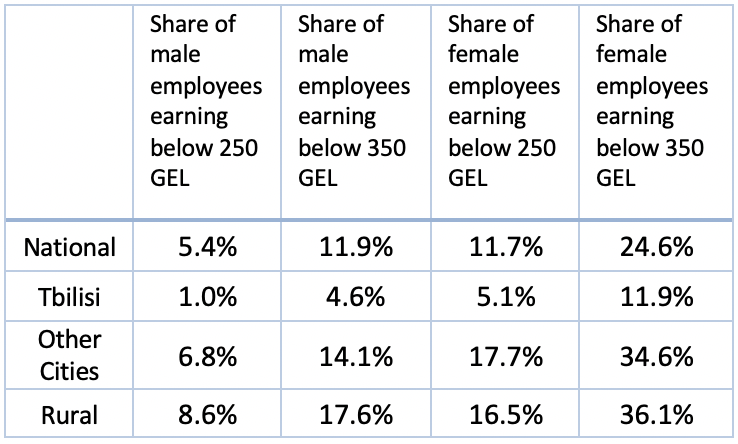
Source: Authors’ calculations based on the Labor Force Survey (Geostat, 2021).
The expected household income after the minimum wage increase was calculated and then compared to the poverty threshold (for each household in a standard way, using the “adult equivalence” scale). According to this methodology, any person who lives in a household which falls below the poverty threshold is considered to be poor. A “working poor” household is defined as a household below the poverty threshold where at least one adult is working.
Figure 1 shows that there is a substantial share of both men and women whose monthly wage income falls below the hypothetical minimum wage thresholds. In addition, women are more than two times as likely to be earning below these thresholds. However, the possible impact from an increased minimum wage on female vs. male poverty is not clear-cut. Since many women are part of larger households which include adult males, their possible income losses/gains may be counterbalanced by income gains/losses of male family members, leaving the overall effect on household income ambiguous.
In addition, poverty rates are not likely to be much affected by a minimum wage increase if most poor households are “non-working poor” (where adult family members are either unemployed or outside of the labor force), a consideration particularly relevant for Georgia. The share of poor individuals who live in “working poor” households (with at least one household member employed) is just 41 percent nationally (and 35 percent in rural areas), meaning that close to 60 percent of poor individuals nationwide (and 65 percent in rural areas) are not likely to be directly affected by minimum wage increases.
Female vs. Male Poverty: Scenarios Following a Minimum Wage Increase
As one can see in Figure 2, increased minimum wages tend to reduce poverty, but the impact is not larger than one percentage point. Not surprisingly, females benefit more than males (0.3 and 0.8 percentage points vs. 0.2 and 0.9 percentage points poverty reduction for men and women respectively, under different threshold scenarios). The maximum positive impact on poverty reduction is observed under a higher minimum wage threshold.
Figure 2. Estimated impact on poverty rates, based on the national subsistence minimum.
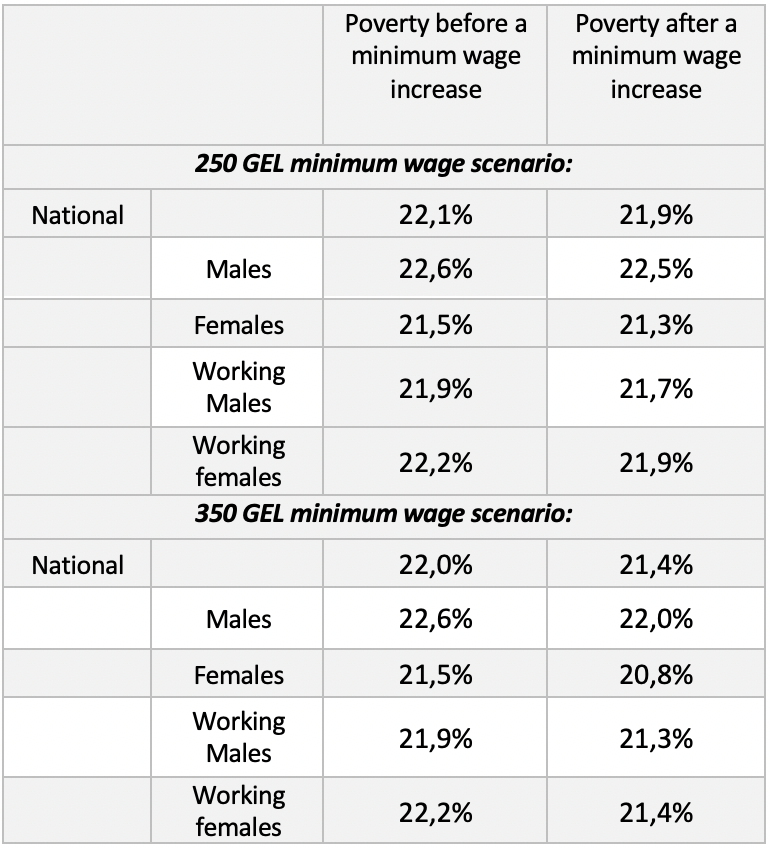
Source: Authors’ calculations based on the Household Integrated Expenditure Survey (Geostat, 2021).
The impact of an increased minimum wage on the expected median consumption of households doesn’t exceed a few percentage points either, as illustrated in Figure 3.
Figure 3. Median monthly consumption per “equivalent adult” in the household under the status quo and minimum wage scenarios, 2021.
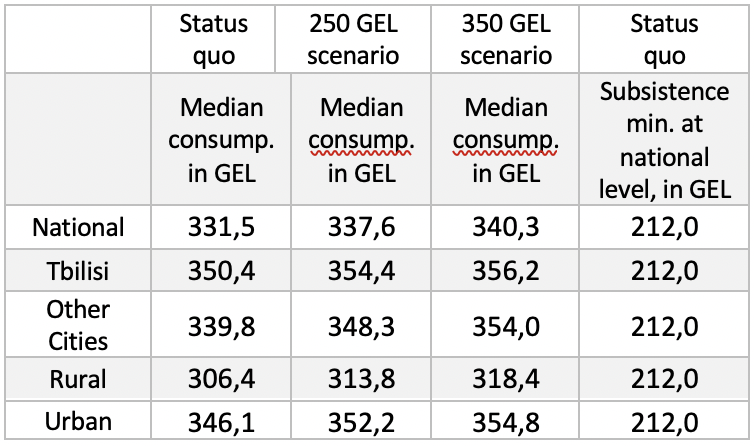
Source: Authors’ calculations based on the Household Integrated Expenditure Survey (Geostat, 2021).
The impact is greatest in urban areas other than Tbilisi (between a 2.5 percent and a 4.2 percent increase in median consumption relative to the status quo). The lower impact in Tbilisi is most likely driven by relatively higher wages, while the low impact in rural areas is likely driven by lower participation in wage employment.
Conclusions
In the hypothetical case of Georgia, an impact of a minimum wage increase on poverty rates is expected to be limited, in line with the literature. In our study this finding is mostly driven by the fact that only a relatively small share of poor individuals live in “working poor” households (about 40 percent, nationally). The remaining 60 percent of poor individuals will be unaffected by the reform.
The quantitative impact on female and male poverty is estimated to be low, although the female poverty rate reduction is somewhat larger than among males.
It is important to note that the analysis doesn’t consider possible differential impacts on different groups of vulnerable families, such as families with small children and single mothers with small children. Some reasons to why groups of households may or may not be affected by the hypothetical minimum wage increase, based on their employment status and other factors, have been discussed above.
Another important point is that our exercise should not be seen as an argument against an increase of the minimum wage in Georgia. Instead, it suggests that such a reform would not have much of an impact if done in isolation. Indeed, the existing literature on minimum wage seems to be in consensus on the fact that minimum wage policies would be more impactful if supplemented by the following measures:
- Maintain and expand targeted social assistance to groups that do not benefit or that are losing jobs/incomes as a result of the minimum wage changes
- Have job re-training programs in place to help laid-off workers
- Have human capital investment programs in place to increase workers’ productivity, in particular for low-productivity sectors
- Consider other support instruments targeted toward the most affected groups of the population such as single working mothers etc.
These recommendations should be incorporated in the policy making regarding minimum wages in Georgia.
Acknowledgement
We are grateful to Expertise France for financially supporting the original report (Babych et al., 2022), which features some of the results and points raised in this policy brief.
References
- Allegretto, S., Dube, A., Reich, M., & Zipperer, B. (2017). Credible Research Designs for Minimum Wage Studies: A Response to Neumark, Salas, and Wascher. ILR Review, 70(3), 559–592. https://doi.org/10.1177/0019793917692788
- Babych, Y., Pignatti, N., Chapichadze, A., Lobzhanidze, G. and Shubitidze, E. (2022). Report on Minimum Wage in Georgia. ISET Policy Institute. Unpublished manuscript.
- Belman, D. and Wolfson, Paul J. (2014). What Does the Minimum Wage Do? Kalamazoo, MI: W.E. Upjohn Institute for Employment Research. https://doi.org/10.17848/9780880994583
- Burkhauser, R. V. and Sabia, J. J. (2007). The effectiveness of minimum‐wage increases in reducing poverty: Past, present, and future. Contemporary Economic Policy, 25(2), 262-281. https://doi.org/10.1111/j.1465-7287.2006.00045.x
- DeFina, R. H. (2008). The impact of state minimum wages on child poverty in female-headed families. Journal of Poverty, 12(2), 155-174. https://doi.org/10.1080/10875540801973542
- Dube, A., T.W. Lester, and M. Reich. 2010. Minimum Wage Effects Across State Borders: Estimates Using Contiguous Counties. The Review of Economics and Statistics, 92(4), 945–964. https://doi.org/10.1162/REST_a_00039
- European Commission. (2020). Proposal for a directive of the European parliament and of the council on adequate minimum wages in the European Union. https://eur-lex.europa.eu/legal-content/EN/TXT/?uri=CELEX%3A52020PC0682GEOSTAT
- International Labour Organization (ILO). (2023). https://www.ilo.org/global/topics/wages/minimum-wages/definition/lang–en/index.htm
- International Labour Organization (ILO). (2019). The working poor or how a job is no guarantee of decent living conditions chrome-extension://efaidnbmnnnibpcajpcglclefindmkaj/https://www.ilo.org/wcmsp5/groups/public/—dgreports/—stat/documents/publication/wcms_696387.pdf
- Geostat. (2021). https://www.geostat.ge/en
- Laroque, G. & Salanié, B. (2002). Labour market institutions and employment in France. Journal of Applied Econometrics, 17(1), 25-48. https://doi.org/10.1002/jae.656
- Neumark, D. & Wascher, W. (2011). Does a higher minimum wage enhance the effectiveness of the Earned Income Tax Credit? ILR Review, 64(4), 712-746. https://doi.org/10.1177/001979391106400405
- Neumark, D. (2018). Employment effects of minimum wages. IZA World of Labor 2018: 6. https://wol.iza.org/articles/employment-effects-of-minimum-wages/long
- Sabia, J. J., Burkhauser, R. V. & Hansen, B. (2012). Are The Effects Of Minimum Wage Increases Always Small? New Evidence From A Case Study Of New York State. Sage Publications, 350-376. https://doi.org/10.1177/001979391206500207
- Sabia, J. J. (2008). Minimum wages and the economic wellbeing of single mothers. Journal of Policy Analysis and Management, 27(4), 848-866. https://doi.org/10.1002/pam.20379
- Sotomayor, O. J. (2021). Can the minimum wage reduce poverty and inequality in the developing world? Evidence from Brazil. World Development 138. https://doi.org/10.1016/j.worlddev.2020.105182.
Disclaimer: Opinions expressed during events and conferences are those of the authors; they do not necessarily reflect those of the FREE Network and its research institutes.
Minimum Wage Spike and Income Underreporting
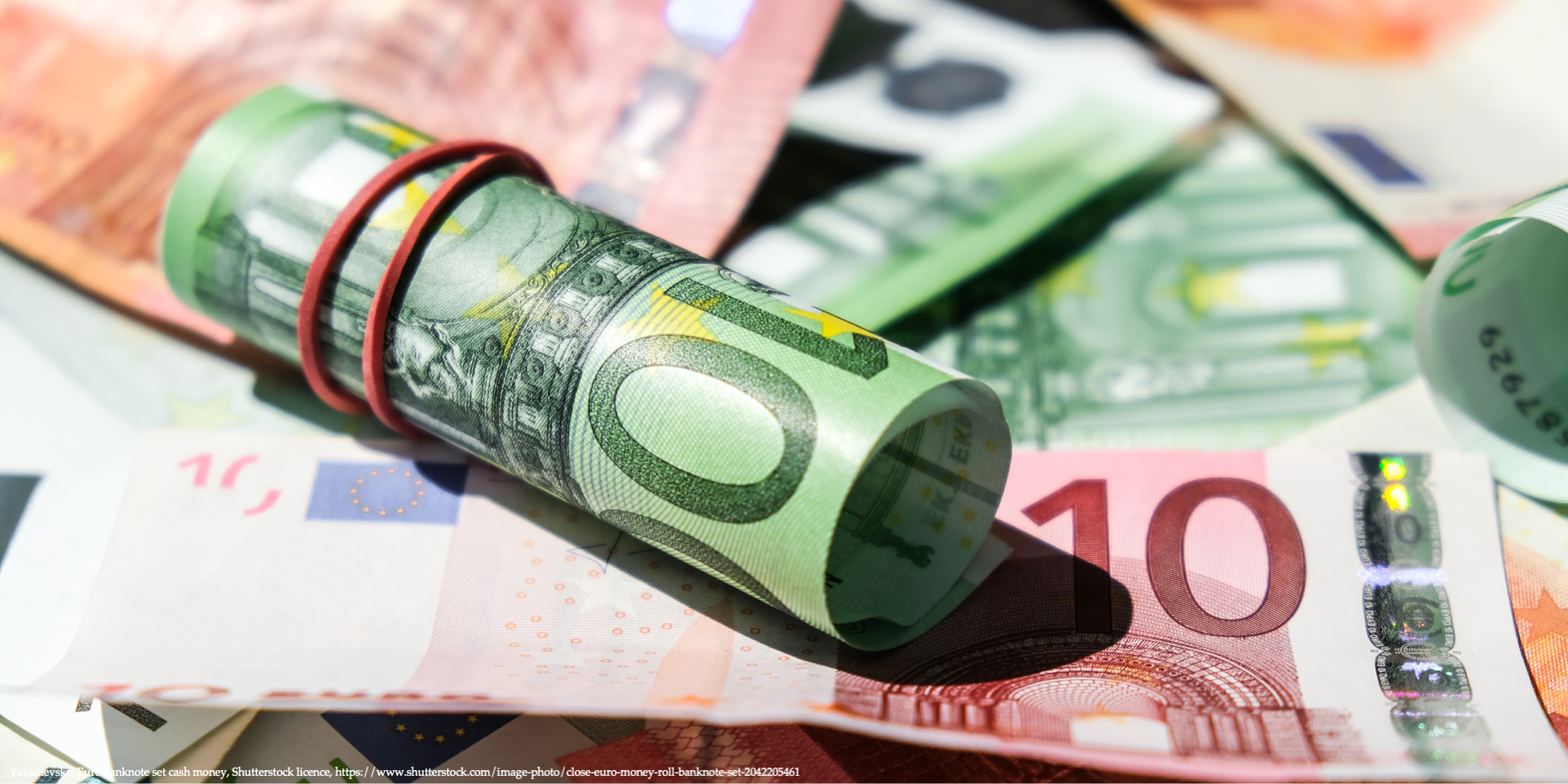
The labor markets of many transition countries are characterized by two features: a spike at the minimum wage level in the wage distribution and widespread use of so-called envelope wages, i.e. non-declared cash payments in addition to the official wage. In this brief, we present a body of suggestive evidence showing that tax evaders are overrepresented among minimum wage earners in Latvia.
Introduction
Labor markets in many transition and post-transition countries are characterized by the prevalence of payroll tax evasion in the form of envelope wages, i.e. non-declared cash in addition to the official wage (see for instance Putnins and Sauka (2015) for Latvia, Paulus (2015) and Kukk and Staehr (2014) for Estonia and Bíró et al. (2022) and Elek et al. (2012) for Hungary).
Another defining characteristic of these transition economies is a very large peak at exactly the minimum wage in the wage distribution. To explain this phenomenon, Tonin (2011) argues that the mass of individuals at the minimum wage level is composed to a large extent of workers receiving envelope wages, where employers and employees collude and agree on reporting only the minimum wage to minimize tax liabilities while remaining under the radar of the tax authorities. In such a setup, the minimum wage policy becomes an enforcement tool for the fiscal administration, as it pushes non-compliant firms to convert part of the envelope wage into an official wage so that it reaches the new minimum wage.
However, only scarce concrete evidence shows that payroll tax evaders are overrepresented among minimum wage earners. Considering the regular minimum wage hikes in the region (e.g., a 95 percent increase in Latvia in 2010-2022 and a planned increase by another 24 percent in 2023), understanding the interaction between minimum wage policy and labor tax evasion is crucial.
In this brief, we present a body of suggestive evidence highlighting the prevalence of wage underreporting at exactly the minimum wage level in Latvia.
Data and Methodology
We use Latvian administrative employer-employee data for 2011 to 2015, covering the full Latvian employed population at a monthly rate. To identify tax evasion, we rely on the comparison between small and large firms. The literature studying tax evasion provides considerable evidence showing that small firms tend to evade more taxes than large firms. Kleven et al. (2016) provide a theoretical foundation for this result, showing that collusive evasion is more difficult to sustain in firms with more employees. Empirically, this effect has been documented in many countries (see for instance Putnins and Sauka (2015), Gavoille and Zasova (2021), and Benkovskis and Fadejeva (2022) for the results on Latvia, Bíró et al. (2022) for Hungary, Paulus (2015) for Estonia, and Kumler et al. (2020) for Mexico).
In this brief, we use a very broad definition for firm size categories and divide firms into firms employing 30 or fewer employees as small and firms with more than 30 employees as large. With such a crude definition, it is inevitable that firms below and above the threshold are highly heterogeneous, implying that some firms below the threshold are tax-compliant, while some firms above the threshold are tax-evading. For our purposes though, it is sufficient to assume that the share of evading employees in small firms is larger than that in the sample of large firms.
Results
We begin by plotting the distribution of wages in the private sector. Figure 1 plots monthly wages in the range of 0–1000 Euros in 2011. The right most dashed vertical line in the figure marks the minimum wage (284.57 Euros per month in 2011) and the left most dashed line marks 50 percent of the minimum wage. There are clear spikes at the minimum wage (and at half of the minimum wage). The minimum level wage spike in small firms (top graph) is much more pronounced than in large firms (bottom graph), which is consistent with the idea that the spike is driven by income underreporting.
Figure 1. Gross wage distribution in the private sector in small (< 30 empl.) and large (> 30 empl.) firms in 2011.
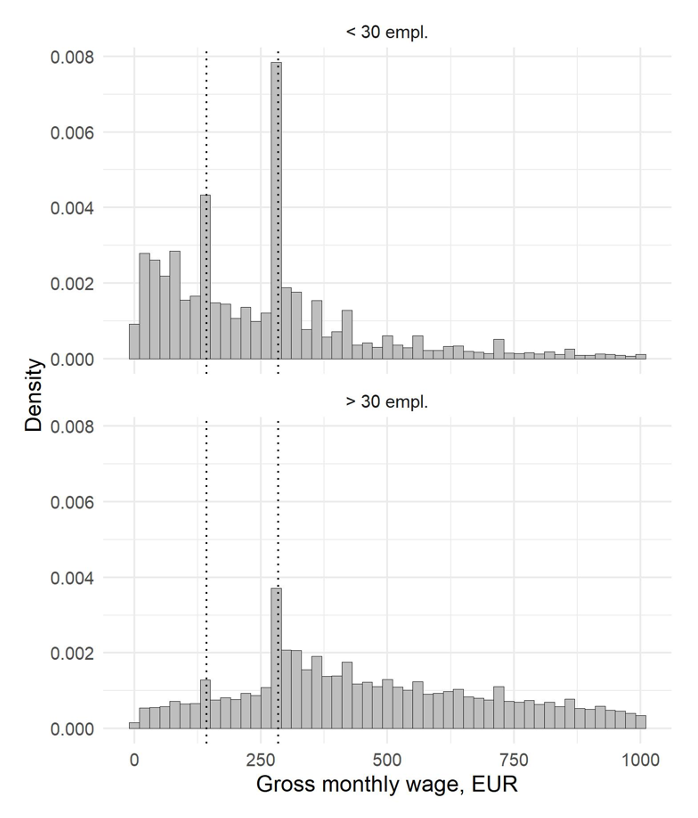
Note: Micro enterprises are excluded. Vertical lines depict the minimum wage (284.57 Euro) and half of the minimum wage (142.29 Euro) in 2011. Source: Authors’ calculations.
This explanation implies that employers and employees choose to declare employment and underdeclare earnings instead of staying completely informal, which is consistent with the available evidence. Staying completely informal involves much higher risks of detection if authorities perform regular inspections of workplaces, and in many Central European countries with prevalent income underreporting, completely informal employment is not very common (OECD, 2008). In Latvia, firms have to register employees in the electronic system of the State Revenue Service before they start to work, hence the probability that an unofficially employed person is detected during a workplace inspection is very high (State Labor Inspectorate, 2010). Existing empirical evidence on Latvia also suggests that income underreporting is much more widespread than completely informal employment, which is estimated at only 2–3.5 percent (European Commission, 2014; Hazans, 2012). Hence, we interpret the spikes as indicative of tax evaders bunching at the minimum wage.
Wage Growth Among Minimum Wage Earners
Wages are expected to grow with tenure, but if minimum wage earners receive part of their income in cash, their reported wage can remain unchanged even after years of employment within a firm (as any increase would arguably go through the non-declared cash). To examine if this is the case, we exploit a period when there were no changes in the Latvian minimum wage (January 2011–December 2013). We select employees who were employed by the same firm in all months of 2011–2013, assign them to wage bins according to their wage in 2011, and in each wage bin calculate the share of workers whose wage in 2013 was the same as in 2011. We assign workers to 10-Euro bins, with the exception of minimum wage earners, whom we assign to a bin of 1 Euro.
As evident from Figure 2 minimum wage earners clearly stand out from other employees. In small firms, almost 45 percent of employees earning the minimum wage in 2011 had the same reported wage in 2013. There is also a spike at the minimum wage in large firms (28 percent), but it is less pronounced than in small firms.
Figure 2. Proportion of continuously employed workers facing no wage growth between 2011 and 2013, by wage bins, in small (< 30 empl.) and large (> 30 empl.) firms.
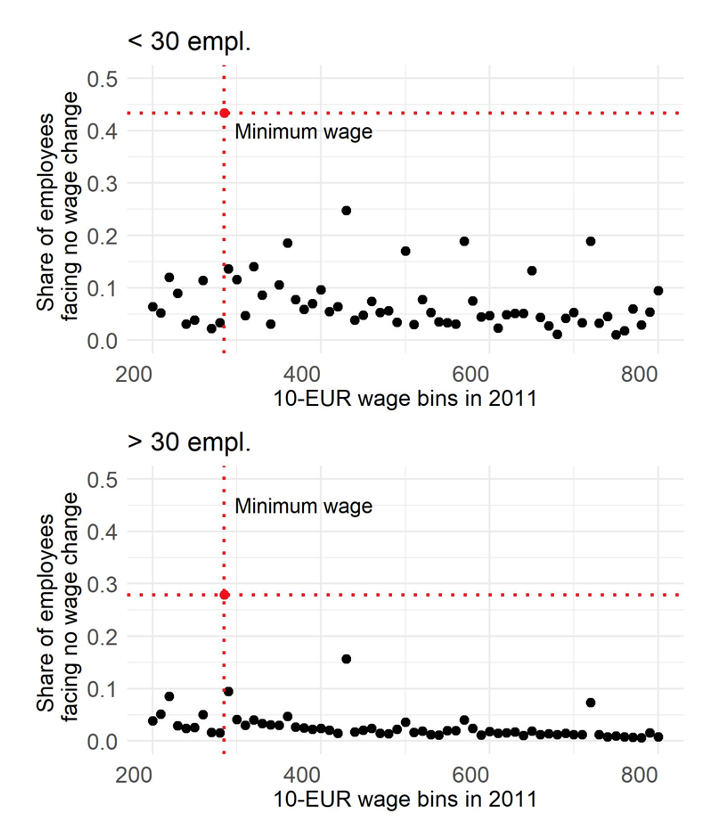
Note: Micro enterprises and public sector firms are excluded. Source: Authors’ calculations.
An alternative explanation for the large share of minimum wage earners who experience no wage growth could be that, for many of them, the minimum wage is binding. To rule this out, we perform the same calculations on a sample of young employees (24 or younger in 2011). Workers in the early stages of their careers tend to have higher returns to experience and tenure; thus, young workers are less likely to have no wage growth after three years of employment with the same firm. Figure 3 plots the results for young workers. In large firms, the spike at the minimum wage is more than twice as small as for the full sample of workers (12 percent vs. 28 percent), but in small firms it remains very high (33 percent).
Figure 3. Proportion of continuously employed young workers (aged 24 or less in 2011) facing no wage growth between 2011 and 2013, by wage bins, in small (< 30 empl.) and large (> 30 empl.) firms.
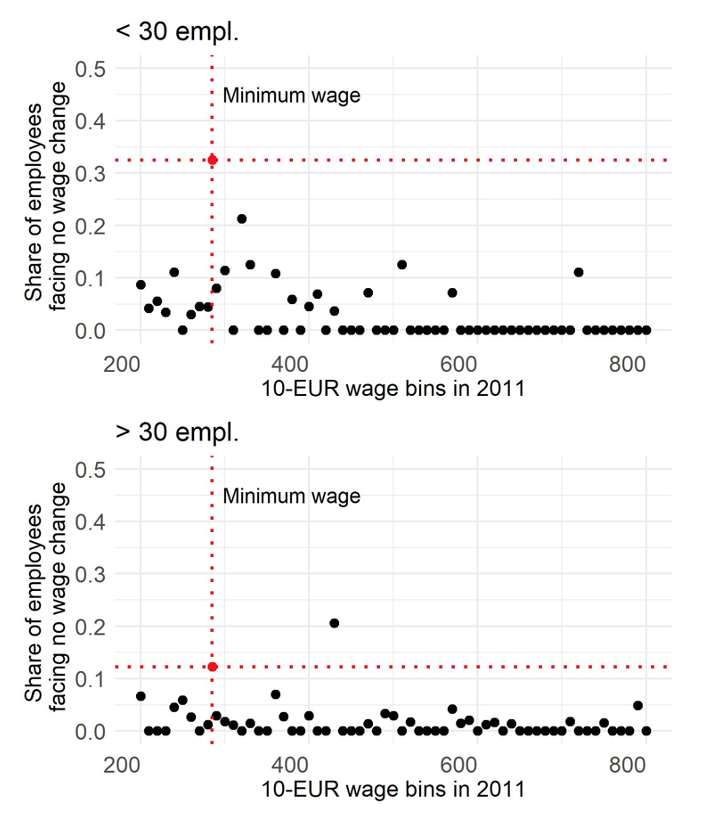
Note: Micro enterprises and public sector firms are excluded. Source: Authors’ calculations.
Conclusion
This brief documents highly prevalent tax evasion among minimum wage earners in Latvia. In such a context, the minimum wage is a powerful fiscal instrument as a higher minimum wage pushes non-compliant firms to disclose a larger share of their employees’ true earnings. In addition, wage underreporting among minimum wage earners can act as a shock absorber and cushion the negative employment effects of a minimum wage hike in countries where a large share of workers officially receive the minimum wage.
These upsides however come at a cost. The results presented in this brief by no means imply that all minimum wage earners are tax evaders; a notable share of employees receiving the minimum wage on paper do honestly earn only the minimum wage. In our paper (Gavoille and Zasova, 2022), we show that the flip side of the positive fiscal effect of a minimum wage hike is job losses among genuine low-wage earners and closures of tax-compliant firms that are affected by the hikes.
Acknowledgement
This brief is based on a recent article published in the Journal of Comparative Economics (Gavoille and Zasova, 2022). The authors gratefully acknowledge funding from LZP FLPP research grant No.LZP-2018/2-0067 InTEL (Institutions and Tax Enforcement in Latvia).
References
- Benkovskis, Konstantins; and Ludmila Fedejeva, 2022. “Chasing the Shadow: the Evaluation of Unreported Wage Payments in Latvia“. Latvijas Banka, Working Paper Nr. 1/2022.
- Bíró , Anikó; Dániel Prinz, and László Sándor, 2022. “The minimum wage, informal pay, and tax enforcement“. Journal of Public Economics, 215, 104728.
- Elek, Péter; János Köllő, Balázs Reizer, and Péter A. Szabó, 2012. “Chapter 4 Detecting Wage Under-Reporting Using a Double-Hurdle Model“. Emerald Group Publishing Limited, Rochester, NY, pp. 135–166.
- European Commission, 2014. “Undeclared Work in the European Union“, Special EUROBAROMETER 284.
- Gavoille, Nicolas; and Anna Zasova, 2022. “Minimum wage spike and income underreporting: A back-of-the-envelope-wage analysis“, Journal of Comparative Economics, forthcoming.
- Gavoille, Nicolas; and Anna Zasova, 2021. “What we pay in the shadow: Labor tax evasion, minimum wage hike and employment“. SSE Riga/BICEPS Research paper No.6.
- Hazans, Mihails, 2012. “How many people are working without a contract in Latvia and neighboring countries?”. Technical Report, University of Latvia.
- Kumler, Todd; Eric Verhoogen, and Judith Frías, 2020. “Enlisting Employees in Improving Payroll Tax Compliance: Evidence from Mexico“. The Review of Economics and Statistics, 102 (5), 881–896.
- Kukk, Merike; and Karsten Staehr, 2014. “Income underreporting by households with business income: evidence from Estonia“. Post-Communist Economies, 26(2), 257-276.
- OECD, 2008. “Declaring Work or Staying Underground“. OECD employment outlook 2008.
- Paulus, Alari, 2015. “Tax Evasion and Measurement Error: an Econometric Analysis of Survey Data Linked with Tax Records“. Working Paper 2015-10. ISER Working Paper Series.
- Putnins, Talis; and Arnis Sauka, 2015. “Measuring the shadow economy using company managers“, Journal of Comparative Economics, 43(2), 471-490.
- State Labor Inspectorate, 2010. “Latvia: Annual Report 2010”
- Tonin, Mirco, 2011. “Minimum wage and tax evasion: Theory and evidence“. Journal of Public Economics, 95(11-12), 1635-1651.
Disclaimer: Opinions expressed in policy briefs and other publications are those of the authors; they do not necessarily reflect those of the FREE Network and its research institutes.
Detecting Labor Tax Evasion Using Administrative Data and Machine-Learning Techniques
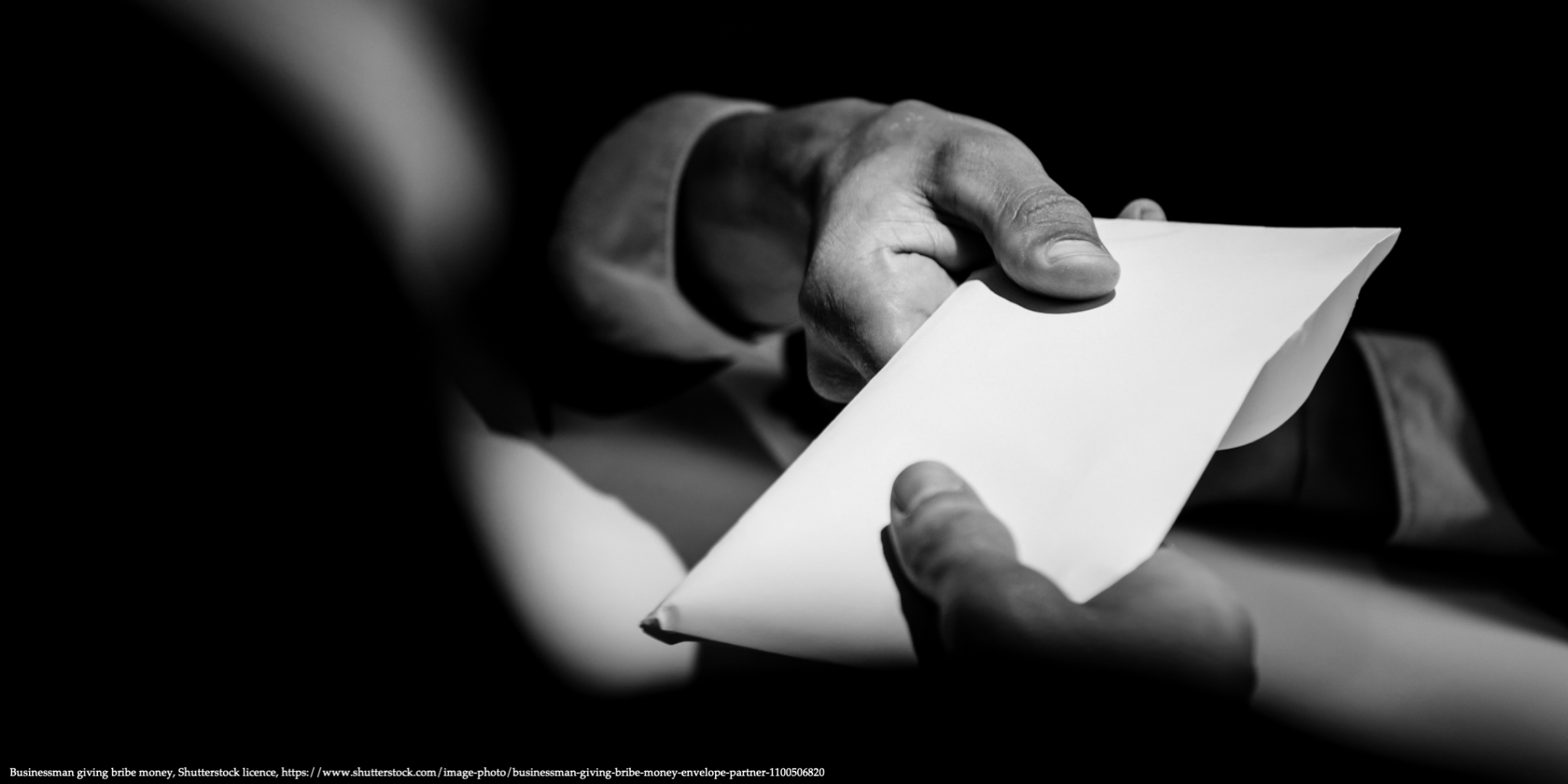
Labor tax evasion is a major policy issue that is especially salient in transition and post-transition countries. In this brief, we use firm-level administrative data, tax authorities’ audit data and machine learning techniques to detect firms likely to be involved in labor tax evasion in Latvia. First, we show that this approach could complement tax authorities’ regular practices, increasing audit success rate by up to 35%. Second, we estimate that about 30% of firms operating in Latvia between 2013 and 2020 are likely to underreport the wage of (some of) their employees, with a slightly negative trend.
Introduction
Tax evasion is a major policy issue that is especially salient in transition and post-transition countries. In particular, “envelop wage”, i.e., an unofficial part of the wage paid in cash, is a widespread phenomenon in Eastern Europe (European Commission, 2020). Putnins and Sauka (2021) estimate that the share of unreported wages in Latvia amounts to more than 20%. Fighting labor tax evasion is a key objective of tax authorities, which face two main challenges. The first is to make the best use of their resources. Audits are costly, so the choice of firms to audit is crucial. The second challenge is to track the evolution of the prevalence of labor tax evasion. For this purpose, most of the existing literature relies on survey data.
In our forthcoming paper (Gavoille and Zasova, 2022), we propose a novel methodology aiming at detecting tax-evading firms, using administrative firm-level data, tax authorities’ audit data and machine learning techniques.
This study provides two main contributions. First, this approach can help tax authorities to decide which firms to audit. Our results indicate that the audit success rate could increase by up to 20 percentage points, resulting in a 35% increase. Second, our methodology allows us to estimate the share of firms likely to be involved in labor tax evasion. To our knowledge, this paper is the first to provide such estimates, which are however of primary importance in guiding anti-tax evasion policy. We estimate that over the 2013-2020 period, about 30% of firms operating in Latvia are underreporting (at least some of) their workers’ wages.
Methodology
The general idea of our approach is to train an algorithm to classify firms as either compliant or tax-evading based on observed firm characteristics. Tax evasion, like any financial manipulation, results in artifacts in the balance sheet. These artifacts may be invisible to the human eye, but machine learning algorithms can detect these systematic patterns. Such methods have been applied to corporate fraud detection (see for instance Cecchini et al. 2010, Ravisankar et al. 2011, West and Bhattacharya 2016).
The machine learning approach requires a subsample of firms for which we know the “true” firm behavior (i.e., tax-evading or compliant) in order to train the algorithm. For this purpose, we propose to use a dataset on tax audits provided by the Latvian State Revenue Service (SRS), which contains information about all personal income tax (PIT) and social security contributions (SSC) audits carried out by SRS during the period 2013-2020, including the outcome of the audit. The dataset also contains a set of firm characteristics and financial indicators, covering both audited and non-audited firms operating in Latvia (e.g., turnover, assets, profit). Assuming that auditors are highly likely to detect misconduct (e.g., wage underreporting) if present, audit outcomes provide information about a firm’s tax compliance. Firms sanctioned with a penalty for, say, personal income tax fraud are involved in tax evasion, whereas audited-but-not-sanctioned firms can be assumed compliant. The algorithm learns how to disentangle the two types of firms based on the information contained in their balance sheets. Practically, we randomly split the sample of audited firms into two parts, the training and the testing subsamples. In short, we use the former to train the algorithm, and then evaluate its performance on the latter, i.e., on data that has not been used during the training stage. If showing satisfying performance on the training sample, we can then apply it to the whole universe of firms and obtain an estimate of the share of tax-evading firms.
In this study, we successively implement four algorithms that differ in the way they learn from the data: (1) Random Forest, (2) Gradient Boosting, (3) Neural Networks, and (4) Logit (for a review of machine learning methods, see Athey and Imbens, 2019). These four data mining techniques have previously been used in the literature on corporate fraud detection (see Ravisankar et al. 2011 for a survey). Each of these four algorithms has specific strengths and weaknesses, motivating the implementation and comparison of several approaches.
Results
Predictive Performance
Table 1 provides the out-of-sample performance of the four different algorithms. In other words, it shows how precise the algorithm is at classifying firms based on data that has not been included during the training stage. Accuracy is the percentage of firms correctly classified (i.e., the model prediction is consistent with the observed audit’s outcome). In our sample, about 44% of audited firms are required to pay extra personal income tax and social security contributions. This implies that a naive approach predicting all firms to be evading would be 44% accurate. Similarly, a classification predicting all firms to be tax compliant would be correct in 56% of the cases. This latter number can be used as a benchmark to evaluate the performance of the algorithms. ROC-AUC (standing for Area Under the Curve – Receiver Operating Characteristics) is another widespread classification performance measure. It provides a measure of separability, i.e., how well is the model able to distinguish between the two types. This measure is bounded between 0 and 1, the closer to 1 the better the performance. A score above 0.8 can be considered largely satisfying.
Table 1. Performance measures
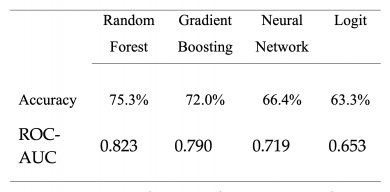
Source: authors’ calculations
Random Forest is the algorithm providing the best out-of-sample performance, with more than 75% of the observations in the testing set correctly classified. Random Forest is also the best performing model according to the ROC-AUC measure, with performance slightly better than Gradient Boosting.
Our results imply that a naive benchmark prediction is outperformed by almost 20 percentage points by Random Forest and Gradient Boosting in terms of accuracy. It is important to emphasize that this improvement in performance is achieved using a relatively limited set of firm-level observable characteristics that we obtained from SRS (which is limited compared to what SRS has access to), and that mainly come from firms’ balance sheets. This highlights the potential gain of using data-driven approaches for the selection of firms to audit in addition to the regular practices used by the fiscal authorities. It also suggests a promising path for further improvements, as in addition to this set of readily available information the SRS is likely to possess more detailed limited-access firm-level data.
Share of Tax-Evading Firms Over Time and Across NACE Sectors
We can now apply these algorithms to the whole universe of firms (i.e., to classify non-audited firms). Figure 1 shows the share of firms classified as tax-evading over the years 2014 to 2019 for our two preferred algorithms – Gradient Boosting and Random Forest. Random Forest (the best performing algorithm) predicts that 30-35% of firms are involved in tax evasion, Gradient Boosting predicts a slightly higher share (around 40%). Both algorithms, especially Random Forest, suggest a slight reduction in the share of tax-evading firms since 2014.
Figure 1. Share of tax-evading firms over time
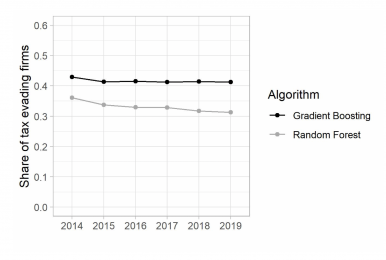
Source: authors’ calculations
The identified reduction, however, does not necessarily imply that the overall share of unreported wages has declined. In fact, existing survey-based evidence (Putnins and Sauka, 2021) indicate that the size of the shadow economy as a share of GDP remained roughly constant over the 2013-2019 period, and that there was no reduction in the contribution of the “envelope wages”. With our method, we are estimating the share of firms likely to be involved in labor tax evasion. Unlike the survey approach, our methodology does not allow the measurement of tax-evasion intensity. In other words, the share of non-tax compliant firms may have decreased, but the size of the envelope may have increased in firms involved in this scheme.
Next, we disaggregate the share of tax-evading firms by the NACE sector. Figure 2 displays the results obtained with Random Forest, our best performing algorithm.
Figure 2. Share of tax-evading firms by NACE, based on Random Forest
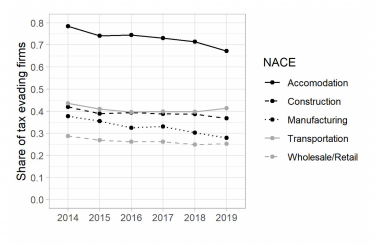
Source: authors’ calculations
First, the sector where tax evasion is the most prevalent is the accommodation/food industry, where the predicted share of tax-evading firms is 70-80%. Second, our results indicate that the overall decrease in the share of firms likely to evade is not uniform. It is mostly driven by the accommodation/food and manufacturing sectors. Other sectors remain nearly flat. This highlights the fact that labor tax evasion varies both in levels and in changes across sectors.
Conclusion
We show that machine learning techniques can be successfully applied to administrative firm-level data to detect firms that are likely to be involved in (labor) tax evasion. Machine learning techniques can be used to improve the selection of firms to audit in order to maximize the probability to detect tax-evading firms, in addition to the regular practices already used by SRS. Our preferred algorithms – Random Forest and Gradient Boosting – outperform the naive benchmark classification by almost 20 percentage points, which is a substantial improvement. Once implemented, the use of these tools can improve the audit effectiveness at virtually no extra cost.
Our findings also suggest a promising path for further improvements in the application of such methods. The improvement in predictive power achieved by our proposed algorithm is attained by using a limited set of variables readily available from the firms’ balance sheets. Given that SRS is likely to have access to more detailed firm-level information that cannot be provided to third parties, there is clear room for improving the performance of the algorithms by using such limited-access data.
Acknowledgement: The authors gratefully acknowledge funding from the Latvian State Research Programme “Reducing the Shadow Economy to Ensure Sustainable Development of the Latvian State”, Project “Researching the Shadow Economy in Latvia (RE:SHADE)”; project No VPP-FM-2020/1-0005.
References
- Athey, Susan, and Guido Imbens. 2019. “Machine Learning Methods That Economists Should Know About.” Annual Review of Economics 11: 685–725.
- Cecchini, Mark, and Haldun Aytug, and Gary J. Koehler, and Praveen Pathak, 2010. “Detecting management fraud in public companies“. Management Science 56, 1146-1160.
- European Commission, 2020. “Undeclared Work in the European Union. Special Eurobarometer 498” (Report)
- Gavoille, Nicolas and Anna Zasova, 2022. “Estimating labor tax evasion using tax audits and machine learning”, SSE Riga/BICEPS Research papers, forthcoming.
- Putnins, Talis, and Arnis Sauka, 2021. “Shadow Economy Index for the Baltic Countries 2009–2020” (Report), SSE Riga
- Ravisankar, Pediredla, and Vadlamani Ravi, and Gundumalla Raghava Rao, and Indranil Bose, 2011. “Detection of financial statement fraud and feature selection using data mining techniques“. Decision Support Systems, 50(2), 491-500.
- West, Jarrod, and Maumita Bhattacharya, 2016. “Intelligent financial fraud detection: a comprehensive review“. Computers & security, 57, 47-66
Disclaimer: Opinions expressed in policy briefs and other publications are those of the authors; they do not necessarily reflect those of the FREE Network and its research institutes.
Gender Gap Widens During COVID-19: The Case of Georgia
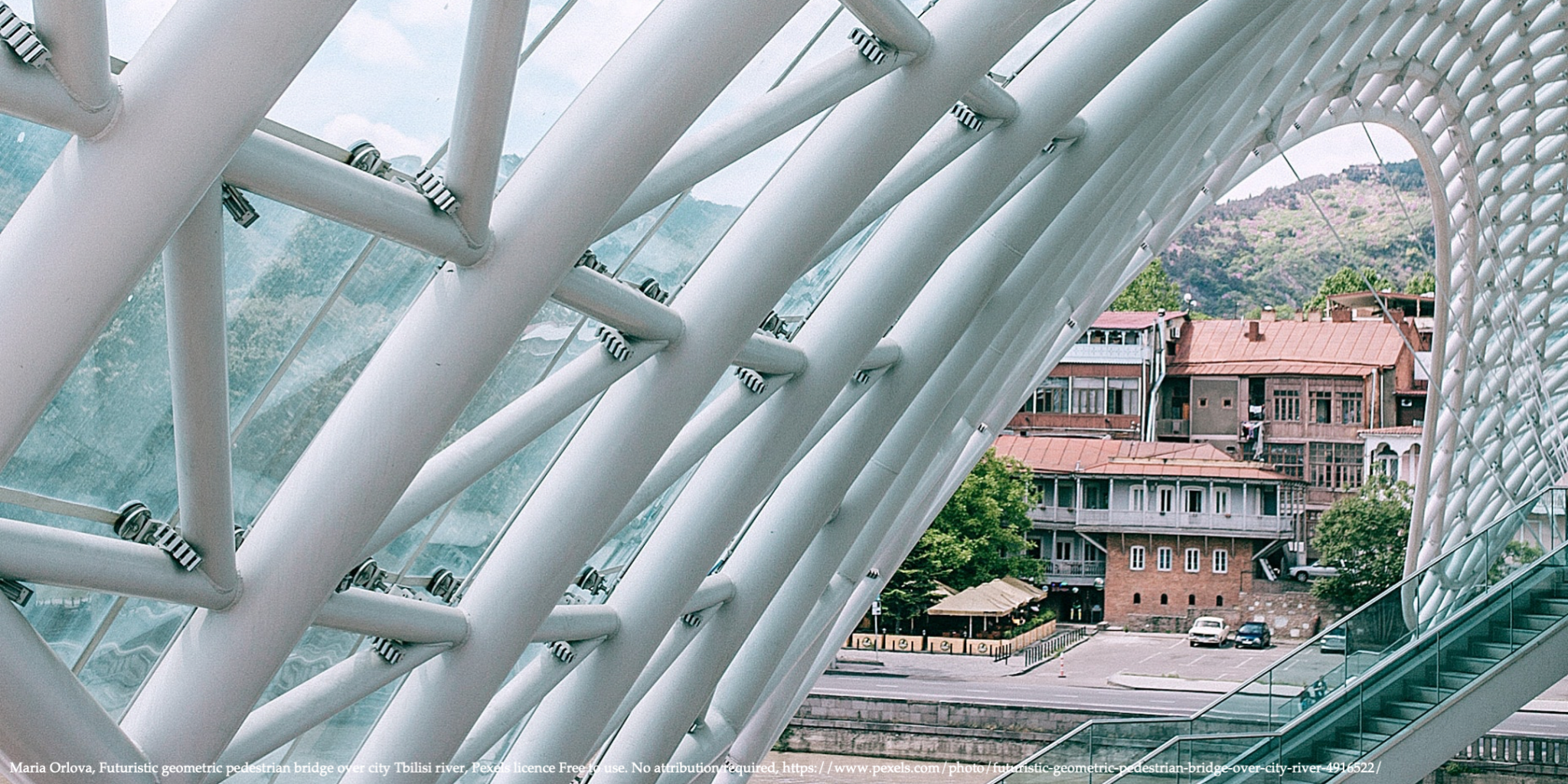
Gender inequality has been a persistent (albeit steadily improving) problem for years. The COVID-induced crisis put women in a disproportionately disadvantaged position, jeopardizing decades of progress achieved towards equality between men and women. However, these effects of the pandemic were not universal across countries. This policy brief aims to evaluate the gender-specific effects of the COVID-19 crisis in Georgia, looking at labor market outcomes and entrepreneurial activities. As expected, the impact of the pandemic was not gender-neutral in this regard, being especially harmful for women. As the Georgian economy rebounds after the crisis, we show that the widened gender gaps are partially offset only in certain aspects. In order to countervail the disproportionate effects of the pandemic, targeted policy measures are needed to stimulate women’s economic activity.
Introduction
Past economic recessions, including the COVID-induced crisis, have never been gender-neutral (e.g., Liu et al., 2021; Ahmed et al., 2020). While economic crises are usually associated with disproportionate negative impacts on labor market outcomes of men compared to women, the impact of the crisis is, debatably, more severe for women-led businesses as compared to their male-led counterparts (e.g., Torres, 2021; Nordman and Vaillant, 2014; Grimm et al.,2012).
The disproportionate labor market outcomes of economic crises are claimed to be due to the fact that men are predominantly employed in cyclical sectors such as construction or manufacturing; therefore, women have to increase their employment during economic downturns as a means of within-family insurance (Alon et al., 2021). The recent COVID-induced crisis, due to its unique nature, turns out to be an exception in this regard. The pandemic and the subsequently-adopted measures primarily adversely affected contact-intensive sectors (where the worker is required to perform tasks in close physical proximity to other people) that predominantly employ women (Mongey, Pilossoph, and Weinberg 2020; Albanesi and Kim 2021). Moreover, large-scale lockdowns increased the burden of unpaid care, which is generally shouldered by women disproportionately (Babych, 2021), leaving less available time for them to work. It should be noted that gender gaps in the labor market were a persistent (albeit steadily improving) problem even before the pandemic (Eurofound, 2016). Therefore, COVID-19 poses a threat jeopardizing the progress achieved in this direction and worsening gender inequality.
COVID-19 brought unprecedented adverse consequences for not only employed workers but entrepreneurs as well. Increased unpaid care and housework pose additional burdens on female top managers, making women-led businesses more vulnerable to the crisis.
The unequal gender implications of the COVID-19 crisis have been widely debated. Growing evidence (Albanesi and Kim 2021; Torres et al., 2021; Alon et al., 2020; Caselli et al., 2020, Fabrizio et al., 2021) attests that, on average, the effects of the pandemic put women in a disproportionately disadvantaged economic position. However, the extent of this effect varies across countries and is absent in some cases (Campa et al., 2021; Torres et al., 2021).
This policy brief aims to examine the gender-specific nature of the COVID-19 crisis in Georgia. With this aim, we study the differential effects of the pandemic on the economic activity of women in terms of labor market outcomes and entrepreneurship. First, we contrast labor market outcomes for Georgian men and women during the COVID-19 crisis. Secondly, we try to assess the magnitude of the disproportionate impact on women-led businesses compared to men-led ones. We calculate gender gaps across different measures of firm-level performance, such as sales revenue, liquidity and owners’ expectations of falling into arrears. Finally, we examine whether there are any signs of recovery yet in 2021 and draw policymakers’ attention to emerging issues.
Labor market highlights
The adverse effects of the pandemic on female employment were conditioned by both supply and demand-side factors. The latter include decreased economic activity, mainly in service-related sectors (hospitality, personal care, etc.) that are dominated by women (Eurofound, 2021). In Georgia, as of 2019, women constituted the majority of workers in sectors such as hospitality (56%), education (83%) and activities of households as employers of domestic personnel (99%) that experienced some of the sharpest declines in employment during 2020. Moreover, women are more likely to be employed in part-time and temporary jobs (14% of women, as opposed to 11% of men, were employed part-time as of 2019, Geostat Labor Force Survey 2019), leaving them more vulnerable during times of crisis. Supply-side factors were triggered by the unequal burden of unpaid work generally undertaken by women in Georgia, mainly due to cultural reasons as well as the higher opportunity cost of time for men (women in Georgia on average earned 64% of men’s salaries in 2019, Geostat). School and daycare closures and decreased childcare involvement of grandparents increased household responsibilities for women. A UN Women survey-based study showed that in the midst of the pandemic in Georgia, around 42% of women reported spending more time on at least one extra domestic task as opposed to 35% of men (UN Women, 2020). This would naturally lead to more women than men leaving the labor force. Indeed, looking at the data, we see that in one year after the COVID-19 outbreak, women contributed to 98% (48,000 individuals) of the decrease in the Georgian labor force in 2020 (Geostat). Moreover, a close look at the percentage point difference between the labor force participation rates of Georgian men and women reveals a notable growth in the gender gap starting from 2020. The same can be said about employment rates (Figure 2).
Figure 1. Difference between male and female labor force participation and employment rates
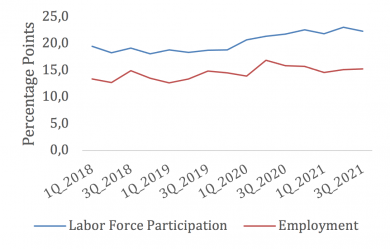
Source: Geostat
To further elaborate on the tendencies in employment, Bluedorn et al. (2021) look at the differences between employment rate changes among male and female workers in 38 advanced and emerging economies. Replicating the exercise with the Georgian data, we can observe results similar to those obtained in Bluedorn et al. (2021). In Figure 2, we see differences between female and male employment rate changes. For each gender group, the latter is computed as an absolute difference between the quarterly employment rate and its annual average level from the previous year. Once the difference takes a negative value, implying that the drop in employment was sharper for women, one could say that we observe a “She-cession” phenomenon as termed by Bluedorn et al. (2021). As we can see, in 2020, the employment rate of women fell more than that of men. This widened gender gap was partially offset in 2021.
Figure 2. Employment rate changes by gender (deviation from the previous year average)
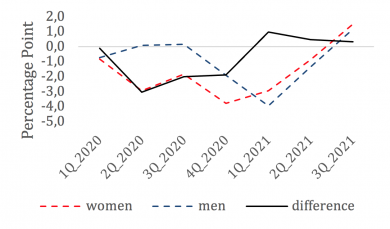
Source: Geostat
Remote work: a burden or a blessing for women?
One important aspect of the COVID-19 crisis was a wide-scale switch to remote work. This development had some gender-specific implications as well. The evidence shows that the prevalence of the switch to remote work was higher among women compared to men (41% vs. 37%) in the EU (Sostero et al., 2020). This tendency also holds in Georgia, where 11% of women as opposed to only 3% of men reported usually working from home in the last three quarters of 2020 (Julakidze and Kardava, 2021). It is not clear whether this tendency can be explained by gender-related occupational differences of male and female jobs (Dingel and Neiman, 2020; Boeri and Paccagnella, 2020; Sostero et al., 2020) or, rather, different personal choices of men and women working in the same occupations. Interestingly, across different countries, we observe a positive correlation between gender inequality (as measured by the Gender Inequality Index) and gender differences in the switch to remote work (measured by the ratio of the share of remote workers among female and male workers). To account for this observation, we can stipulate that gender differences in switching to remote work might be explained by differing gender roles in households, and in society at large, across countries (as proxied by the gender inequality index).
Figure 3. Relative prevalence of remote work among female and male workers
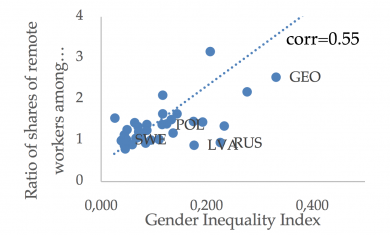
Source: Eurostat, Statistics Sweden, Statista, Geostat, UNDP Human Development Reports
Regardless of the reason, remote work is likely to have some important implications on gender roles. However, the directionality of these implications is not straightforward. On the one hand, remote work offers flexibility for women to juggle household and work responsibilities. On the other hand, since women compared to men have been shown to be more likely to use the time saved from commuting to engage in housework, the switch to remote work might increase their “total responsibility burden” (Ransome, 2007) and lead to time poverty (Peters et al., 2004; Hilbrecht, Shaw, Johnson and Andrey, 2008). Indeed, according to CARE International South Caucasus (2020), around 48% of female survey participants in Georgia placed additional effort into housework and childcare in the midst of the pandemic. Moreover, as women are more likely and expected to use remote working as a means of balancing work-life responsibilities (Moran and Koslowski, 2019) their bargaining power at work decreases relative to their male counterparts. This could have some adverse career implications for female workers. Recent enforced lockdowns might pose an opportunity in this regard, as once-remote work becomes something close to a “new normal” employers will likely decrease the penalty for remote workers.
Spotlight on women-led business performance during the COVID-19 crisis
Calamities brought by the pandemic worsened financial outcomes for enterprises, affecting their ability to operate and have stable financial income. Similar to other crises, the pandemic has not been gender-neutral (Liu et al., 2021; Ahmed et al., 2020) in terms of the effect on business performance.
Gaps in the performance of women- and men-led businesses have been prevalent beyond any economic crisis as well, and have been documented in a number of studies (e.g., Amin, 2011; Bardasi et al., 2011), registering gender differences in sales and productivity in favor of men-owned enterprises. As suggested by Campos et al. (2019), these performance gaps may be due to lower levels of capital owned by women as opposed to men, a smaller number of employees hired by women-owned firms, as well as different practices in using advanced business tools and innovation. In addition, the existence of these gender gaps has also been explained as stemming from the prevailing social norms that assign certain obligations to women. Nordman and Vaillant (2014) and Grimm et al. (2012) suggest that unpaid housework and family-care led to a constrained number of hours women could afford to spend on the work and management of firms, negatively affecting their productivity.
According to the Women Entrepreneurship Report (Global Entrepreneurship Monitor (GEM), 2021), the pandemic imposed an additional burden in terms of increasing family-care duties on women. The GEM survey (2021) conducted in 43 countries worldwide shows that the likelihood of enterprise closure is 20% higher for women-led compared to men-led businesses. The higher likelihood of closure reflects the adverse factors that may have hindered the operating capacity of firms. For example, a survey conducted by UNIDO (2020) suggests that, as a result of the Coronavirus crisis, African and Middle Eastern women-led firms experienced diminished revenues. In addition, 41% of women-led firms were short of cash flow and unable to fulfill financial obligations, while only 32% of male entrepreneurs were exposed to the same problem.
More rigorous analysis on this matter has been conducted by Torres et al. (2021) and Liu et al. (2021). They try to examine the asymmetric effects of the COVID-19 crisis on women-led firms in several dimensions utilizing new datasets from the World Bank: COVID-19 Follow-up Enterprise Survey and the World Bank Business Pulse Survey. The findings of Liu et al. (2021) for 24 countries from Central Europe & Central Asia and Sub-Saharan Africa confirm that during the pandemic women-led businesses are subject to a higher likelihood of closure than men-led businesses and that female top managers are more pessimistic about the future than their male counterparts. Finance and labor factors were mentioned to be the major contributors to these disadvantages; for example, women-led businesses were found to be less likely to receive bank loans compared to men-led businesses. Lastly, the disadvantages experienced by women-led firms were claimed to widen in highly gender-unequal economies and developing countries. Torres et al. (2021) study the impact of the early phase of the COVID-crisis on gender gaps in firm performance for 49 mostly low- and middle-income countries. The results demonstrate that women-led businesses experienced a greater reduction in sales and lower liquidity compared to their male counterparts, which has been reflected in a higher likelihood for women-led companies in several sectors to fall into arrears. On the other hand, as a response to changing circumstances, women-led firms were found to be more likely to increase the utilization of online platforms and make product innovations. Nevertheless, they struggled to obtain any form of public support.
The impact of the pandemic on firms was not gender-neutral in Georgia
The pandemic-induced fragile environment had an adverse impact on entrepreneurs in Georgia– the effects of the shock were significantly more severe for female entrepreneurs than for their male counterparts. In order to assess the gender differences in the impact of the pandemic on firms, we utilize firm-level data on Georgian enterprises from the second round of the World Bank COVID-19 Follow-up Enterprise Survey, conducted in October – November 2020.
Following the methodology as presented in Torres et al. (2021), we assess whether there are differences in the magnitude of reduction in sales revenue (self-reported percentage change in sales revenue one month before the interview as compared to the same period of 2019) and available liquidity for women- and men-led businesses, and whether falling into arrears in any outstanding liabilities is more expected by female top managers (in the next six months from the interview).
Depending on the type of dependent variable, continuous or binary, either Ordinary Least Squares (OLS) or Probit models are estimated, respectively. Along with the gender of the top manager of firms, we also control for sector and firm size. The Georgian database contains a total of 701 enterprises (581 SMEs and 120 micro-businesses).
Table 1. Magnitude of the disproportionate impact of COVID-19 on women-led businesses in Georgia, October-November 2020
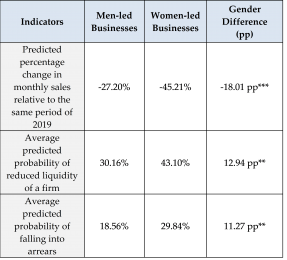
Source: The World Bank COVID-19 Follow-up Enterprise Survey, Second Round. Author’s calculations. ***Significant at the 1% significance level; ** significant at the 5% significance level.
Table 1 presents the results of the regression analysis of gender differences among Georgian enterprises in terms of the impact of the pandemic. As observed, women-led businesses reported larger declines in sales, revenues, and liquidity. The predicted drop in sales was 18 percentage points (pp) higher for enterprises with a female top manager than for men-led firms. The larger drop in sales should have been reflected in the reduced cash flow availability and in hardship to cover operating costs. Indeed, as the results demonstrate, women-led enterprises are on average 12.9 pp more likely to have reduced availability of liquidity. This may explain women’s negative future expectations. Moreover, the average predicted probability of expecting to fall into arrears is 11.3 pp higher for women-led firms in Georgia as compared to men-led businesses.
The unequal effect of the COVID-19 crisis on women-led businesses might have been fueled by the disproportionate burden of unpaid care and housework shouldered by women in Georgia, leaving less time available for work and managing enterprises. On the other hand, as Torres et al. (2021) claim, female business owners tend to employ more female workers (the social group more exposed to the unequal burden of the pandemic) than male owners. This, in turn, could further hamper the productivity of women-led businesses and increase their vulnerability to economic shocks.
On the road to recovery
2021 has been characterized by a rather rapid recovery for the Georgian economy, as evidenced by the 10.6% (preliminary estimate) annual growth rate of real GDP. Signs of recovery can also be observed in the labor market – the labor force increased by 4% (YoY) in the 3rd quarter of 2021, while employment was also characterized by a growing trend (1%, YoY).
Along the lines of economic recovery, the gender gap in the labor market also seems to be narrowing. For instance, the steadily growing gap between male and female labor force participation rates seems to stagnate over 2021 (Figure 1). Moreover, as is illustrated in Figure 2 above, the difference between women’s and men’s employment rate changes is positive in 2021, meaning that the employment rate was increasing more (or decreasing less) for women. If this tendency persists, we might stipulate that the disproportionate effects of the COVID-19 crisis on female employment are on the way to recovery.
To examine whether Georgian firms have experienced concurrent movement in their performance along with the economic recovery, we utilize third-round data (from September 2021) of the World Bank COVID-19 Follow-up Enterprise Survey and scrutinize whether the gender differences have narrowed since the previous round of the survey (Table 2).
Table 2. Magnitude of the disproportionate impact of COVID-19 on women-led businesses in Georgia, September 2021.
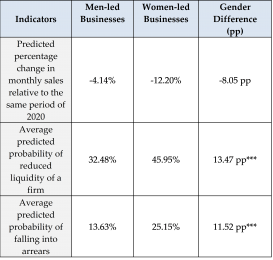
Source: The World Bank COVID-19 Follow-up Enterprise Survey, Third Round. Author’s calculations. ***Significant at the 1% significance level.
Although the third-round survey data suggests that the predicted percentage drop in sales sharply declined for both men- and women-led businesses, the findings are not statistically significant and therefore cannot claim any signs of recovery in the gender gap in this respect. No signs of recovery are observed in terms of average predicted probability of reduced liquidity of firms and expectations of falling into arrears, either. Gender gaps in these two indicators still persist and are as strong in magnitude as in the second-round survey estimates (from October-November 2020). It seems that despite the economic rebound, not all traces of the pandemic crisis for firms have been eradicated from a gender perspective.
Conclusion
The pandemic came with high economic costs. It hit women disproportionately harder, adversely affecting their employment and entrepreneurial prospects. The unequal burden of the COVID-crisis shouldered by women in Georgia could be one of the reasons for the massive labor force dropouts among female workers and poor performance of women-led businesses. Georgian enterprises with female owners experienced a significantly larger decline in sales compared to their male-owned counterparts, consequently suffering from a shortage of cash flow and fears of falling into arrears.
Despite the great rebound in growth after the initial COVID-19 shock, the pandemic-associated increase in the gender gap seems to have been only partially offset in Georgia. In particular, there is a larger positive upsurge in women’s employment rate, as well as a diminishing difference between male and female labor force participation and employment rates. Following the ongoing recovery in sales revenue of Georgian enterprises (though the predicted gender difference was statistically insignificant), the gender gap in sales is shrinking too. But, in spite of the economic rebound, differences in available liquidity and expectations of falling into arrears have not yet been eradicated, indicating that the adverse influence of the pandemic on women still persists. It leaves female entrepreneurs a still more vulnerable group, which could be of special interest to policymakers to ease their liquidity problems.
Policies should also be directed towards encouraging women to become more economically active. In this regard, remote work seems to pose an opportunity if coupled with affordable childcare support policies.
References
- Ahmed, Tanima; Muzi, Silvia; Ueda Kohei. 2020. “Do Crises Hit Female-Managed and Male-Managed Firms Differently?” Evidence from the 2008 Financial Crisis, Enterprise Note Series, 39.
- Albanesi, Stefania; and Jiyeon Kim, 2021. “The Gendered Impact of the COVID-19 Recession on the US Labor Market”, NBER Working Paper Series, 28505.
- Alon, Titan; Coskun, Sena; Doepke, Matthias; Koll, David; and Michèle Tertilt, 2021. “From Mancession to Shecession: Women’s Employment in Regular and Pandemic Recessions”, NBER Working Paper Series, 28632.
- Alon, Titan; Doepke, Matthias; Olmstead-Rumsey, Jane; and Michèle Tertilt, 2020. “This Time It’s Different: The Role of Women’s Employment in a Pandemic Recession”, NBER Working Paper Series, 27660.
- Amin, Mohammad, 2011. ” Labor Productivity, Firm-size and Gender: The Case of Informal Firms in Argentina and Peru”, World Bank Group Enterprise Note, 22.
- Babych, Yaroslava, 2021. “Global Gender Gap in Unpaid Care: Why Domestic Work Still Remains a Woman’s Burden”, FROGEE Policy Brief Series, 4.
- Bardasi, Elena; and Shwetlena Sabarwai, 2011. ” How do female entrepreneurs perform? evidence from three developing regions”, Small Business Economics, 37, 417–441.
- Bluedorn, John C.; Caselli, Francesca G.; Hansen, Niels-Jakob H.; Shibata, Ippei; and Marina Mendes Tavares, 2021. “Gender and Employment in the COVID-19 Recession: Evidence on “She-cessions””, IMF Working Papers, 21/095.
- Boeri, Tito; Caiumi, Alessandro; and Marco Paccagnella, 2020. “Mitigating the work-safety trade-off”, COVID Economics, 2, 60-66.
- Campa, Pamela; Roine, Jesper; and Svante Strömberg, 2021. “Unequal Labour Market Impacts of COVID-19 in Sweden – But Not Between Women and Men”, Intereconomics, 56.
- CARE, 2020. “Rapid Gender Assessment”.
- Caselli, Francesca G.; Grigoli, Francesco; Sandri, Damiano; and Antonio Spilimbergo, 2020. “Mobility under the COVID-19 Pandemic: Asymmetric Effects across Gender and Age”, IMF Working Papers, 20/282.
- Dingel, Jonathan I.; and Brent Neiman, 2020. “How Many Jobs Can be Done at Home?”, NBER Working Paper Series, 26948.
- Eurofound, 2016. “The gender employment gap: Challenges and solutions”, Publications Office of the European Union, Luxembourg.
- Eurofound, 2021. “COVID-19: Implications for employment and working life”, Publications Office of the European Union, Luxembourg.
- Fabrizio, Stefania; Gomes, Diego B. P.; and Marina Mendes Tavares, 2021. “COVID-19 She-Cession: The Employment Penalty of Taking Care of Young Children”, IMF Working Papers, 21/058.
- Global Entrepreneurship Monitor, 2021, “Women’s Entrepreneurship 2020/21 – Thriving Through Crisis.”
- Grimm, Michael; Knorringa, Peter; and Jann Lay, 2012. “Constrained Gazelles: High Potentials in West Africa’s Informal Economy”, World Development, 40(7), 1352–1368.
- Hilbrecht, Margo; Shaw, Susan M.; Johnson, Laura C.; and Jean Andrey, 2008. “‘I’m Home for the Kids’: Contradictory Implications for Work–Life Balance of Teleworking Mothers”, Gender, Work and Organization, 15, 454-476.
- ILO, 2020. “Survey of Women Leading Micro, Small and Medium Businesses About the Main Challenges They Face as a Result of the Coronavirus Crisis.”
- Julakidze, Mery; and Gocha Kardava, 2021. “Five Ways Covid-19 Affected the Georgian Labor Market in 2020”, ISET Economist Blog.
- Liu, Yu; Wei, Siqi; and Jian Xu, 2021. “COVID-19 and Women-Led Businesses around the World”, Finance Research letters, 43.
- Mongey, Simon; Pilossoph, Laura; and Alex Weinberg, 2020. “Which Workers Bear the Burden of Social Distancing?”, NBER Working Paper Series, 27085.
- Moran, Jessica; and Alison Koslowski, 2019.“Making use of work–family balance entitlements: how to support fathers with combining employment and caregiving”, Community, Work and Family, 22, 111-128.
- Nordman, Christophe, Jalil; and Julia Vaillant, 2014.“ Inputs, Gender Roles or Sharing Norms? Assessing the Gender Performance Gap Among Informal Entrepreneurs in Madagascar”, IZA Discussion Paper.
- Peters, Pascale; Tijdens, Kea G.; and Cécile Wetzels, 2004. “Employees’ opportunities, preferences, and practices in telecommuting adoption”, Information and Management, 41,469-482.
- Ransome, Paul, 2007. “Conceptualizing boundaries between ‘life’ and ‘work’”, The International Journal of Human Resource Management, 18, 374-386.
- Sostero, Matteo; Milasi, Santo; Hurley, John; Fernancez Macias, Enrique; and Martina Bisello, 2020. “Teleworkability and the COVID-19 crisis: a new digital divide?”, JRC Working Papers Series on Labour, Education and Technology, 2020/05.
- Torres, Jesica; Maduko, Franklin; Gaddis, Isis; Iacovone, Leonardo; and Kathleen Beegle, 2021. “The impact of the Covid-19 pandemic on Women-Led Businesses”, The World Bank Policy Research Working Paper, 9817.
- UN Women, 2020. “Rapid Gender Assessment of the Covid-19 Situation in Georgia”.
- UN Women, 2021. “Women’s Entrepreneurship Expo 2021 report”.
- UNIDO, 2020. “Assessment of the impact of Covid-19 outbreak on Women and youth entrepreneurs in the manufacturing sector and manufacturing-related services.
Disclaimer: Opinions expressed in policy briefs and other publications are those of the authors; they do not necessarily reflect those of the FREE Network and its research institutes.
Assessing a Model for the Implementation of an Equal Pay Review and Reporting (EPRR) Methodology in Georgia
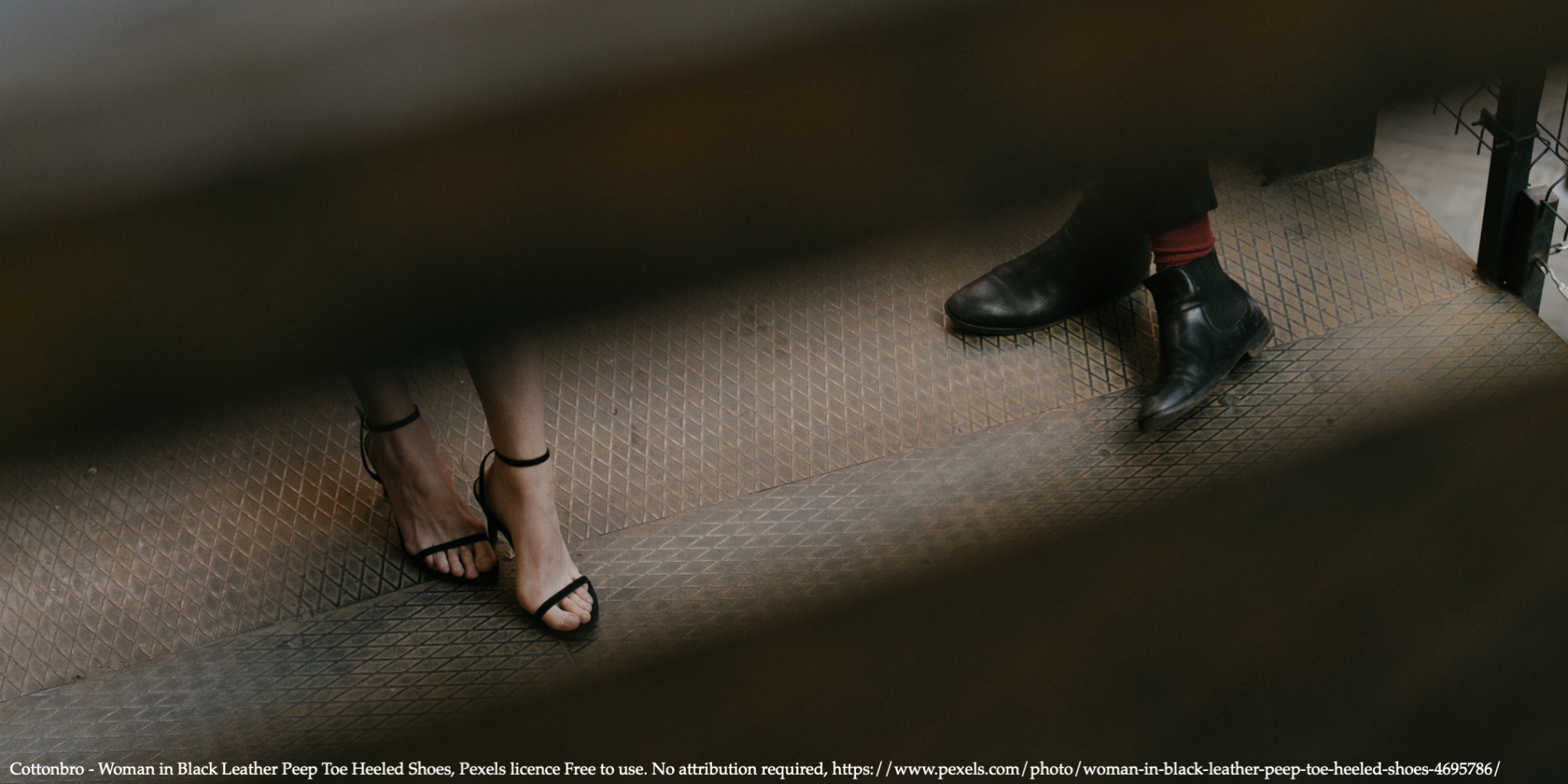
Georgia’s gender pay gap has started to attract the attention of the population and policymakers alike. The gap persists despite working women generally reporting better labor-market skills and personal characteristics. It has been argued that this could be the result of systematic gender-based workplace wage discrimination, resulting in unequal pay for equal work. The discussion that ensued highlights how the fight to guarantee equal pay for equal work could benefit from establishing an Equal Pay Review and Reporting Mechanism. In response, the ISET-PI team – after reviewing the best international practices – devised and tested an excel based tool that could help companies and governmental agencies identify, monitor, and fight gender discrimination in Georgia. The main quantitative result of the exercise identified that, should reporting be made mandatory, extending the obligation to companies that employ up to 50 people would make the administrative costs for companies and public administration up to twenty times higher; thus, the usefulness of the tool was found to be substantially limited when applied to smaller companies. Finally, the exercise emphasized the reluctance of companies to provide the data required, leading to the conclusion that the successful implementation of such an initiative would require the enforcing agency to have the legal authority to sanction failures to provide the necessary data.
Introduction
One of the key gender inequality indicators is the gender pay gap – or gender wage gap – calculated as the average difference between the remuneration for men and women in the labor market. Its evolution is monitored worldwide, and closing this gap is considered a key step towards more inclusive and prosperous economies and societies. According to the World Economic Forum, as of 2020, no country (including the top-ranked ones) had yet achieved gender parity in wages.
In Georgia, the unadjusted hourly gender pay gap amounts to 17.7 percent of the average male hourly wage (UN Women, 2020). Moreover, when controlling for personal characteristics of men and women, the adjusted hourly gender pay gap in Georgia is estimated to be 24.8 percent (UN Women, 2020). This implies that women, on average, have better observable labor-market characteristics but are still paid less than men.
These findings prompted a core discussion within the Georgian society on the presence of unequal pay for equal work in Georgia as one of the possible reasons for the gap and how to tackle the problem. The idea of equal pay for equal work entails that individuals in the same workplace are given equal pay if they perform the same type of work. Consequently, this potential source of the pay gap can only be verified at the individual employer level. This is accomplished by calculating the unexplained gender pay gap at the organizational/employer level and validating whether, and why, these differences exist.
Given the attention the topic holds in the national discourse, ISET Policy Institute created and tested an excel tool, built in line with the international best practices and adapted to the Georgian context, to help employers and government offices identify and measure the differences in wages between men and women performing equal work. During this process, the team learned several noteworthy lessons, as summarized in this policy brief.
International Experience
There is growing consensus that transparency is critical when dealing with pay inequality and, therefore, gender pay reporting should become the norm. Since 2010, several (mostly developed) countries have introduced reporting schemes to monitor gender pay gaps, promote awareness about gender equality issues throughout society (particularly among employees), and increase organizations’ accountability to address gender inequalities (Equileap, 2021).
However, the gender pay gap is a key issue for which the disclosure of information remains particularly low. Equileap’s 2021 report revealed that 85 percent of organizations worldwide did not publish information on remuneration differences between female and male workers in 2020.
Three countries, according to Equileap, lead the way in gender pay gap reporting: Spain, the UK, and Italy (Figure 1). In each of these top three countries, reporting is mandatory.
Figure 1. Percentage of organizations publishing gender pay information, per country
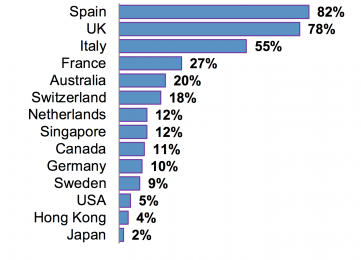
Source: Equileap, 2021. The figure only includes countries for which more than 49 surveyed organizations were included in the Equileap dataset.
However, even in these countries, and, more generally, in all countries scrutinized by Equileap but Iceland, firms with 50 or fewer employees are not required to report on gender pay gaps.
The Case of Georgia
Georgian legislation clearly establishes the principle of equal pay for equal work for all employees. The requirement applies to both public and private organizations. Nevertheless, enforcement of the law remains a significant challenge.
At present, Georgia has no reporting requirements regarding employee salaries for private organizations. It has not yet designed a reporting scheme for equal pay for equal work, nor has it assigned the task of collecting this information to any governmental body.
Moreover, Labour Inspectorate representatives state that few wage discrimination cases are currently being filed in the country. The main reason behind this is that norms regarding equal pay for equal work have never been properly specified. In addition, there are no explicit criteria defining the concept of ‘equal work’. Thus, employers and employees alike do not seem to fully understand the phrase – equal pay for equal work.
The Excel Tool
After a careful review of the three tools presently utilized to calculate gender pay inequality (the Swiss Logib, the German Logib-D, and the Diagnosis of Equal Remuneration (DER) tool developed by UN Women), ISET-PI built a Georgian model as a modified version of the DER tool that is adapted to the Georgian context and includes some variables from the Swiss tool.
The tool itself is an excel file with several worksheets. The two main facets are the inputted data sheet and the results sheet. Companies may input information on their employees in the data sheet, and the findings will then be demonstrated in the results sheet. The tool first identifies people performing the same work, and classifies jobs based on their official titles, alongside managerial responsibilities and skill requirements. After individuals are grouped by job, the tool calculates the average salary within each group separately for men and women. Thereafter, the pay gap is calculated based on the average salary for the two gender groups.
With the support of the Employers’ Association, several companies of all sizes were approached to test the tool. Unfortunately, only a few agreed to participate, and just two completed the trial: one small-sized enterprise (with 50 or fewer employees) and a large-sized enterprise (with 250 or more employees).
While low participation rates have significantly limited our analysis, we still obtained several important insights which are discussed in the next subsection.
Findings
Firstly, it is important to note that companies’ willingness to share anonymized salary data was very low, even among the companies that completed the test.
Secondly, the usefulness of the tool for obtaining a comprehensive view of equal pay for equal work in small companies (with 50 or fewer employees) appeared fairly limited as few people within the same firm perform the same job.
Thirdly, we performed a simple cost assessment exercise to evaluate the compliance costs – to both companies and the government – of collecting and reporting the gender pay gap. We found that extending the data collection requirement to small companies would increase the compliance costs by up to 20 times (high-cost scenario) compared to an example where small companies are exempt. This is because there are many more small companies in Georgia (146,802), than those classified as medium or large ones (2,752 and 609, respectively).
In addition, during the implementation of the exercise, we became aware of the following:
- Under the existing legal provisions, it would be extremely difficult to introduce the EPRR in a mandatory format – no governmental agency could sanction companies for failing to comply.
- Opting for the mandatory option and sanctioning the emergence of unequal pay in certain job categories could incentivize companies to manipulate the data input. In this case, therefore, it would be ill-advised to provide the full tool to companies, as they could more easily adjust data inputting to obtain more favorable indicators through successive iterations.
Conclusion
Setting up an EPRR system is one way to contribute to the implementation of the equal pay for equal work principle.
Designing the Georgian Model for the Implementation of an Equal Pay Review and Reporting Methodology generated several useful insights that might prove valuable for policymakers in Georgia and other developing countries:
1) The EPRR instrument can be utilized for the analysis of gender pay gaps within companies with more than 50 employees. Within smaller companies, evaluating the gender pay gap significantly increases the costs to society, while providing rather limited additional information.
2) The decisions about whether to provide the analytical part of the tool to companies, and whether reporting should be voluntary or mandatory should be taken jointly. If the goal is to provide an instrument to the agency enforcing the equal pay for equal work principle and to facilitate appeals from workers, the tool should be made mandatory. However, in this case, companies should only provide the input data, without having access to the part of the tool that assesses pay gaps at the job level. On the other hand, if the goal of the reform is to support willing companies in their efforts to eliminate unequal pay for equal work conditions, a non-mandatory form may be preferable. In this instance, companies should have access to the full version of the tool. This would allow them to better understand the dynamics that lead to unequal pay and thus put in place internal remedial actions.
3) If the goal is to provide a tool to the agency enforcing the equal pay for equal work principle, it is crucial that any gaps in the associated legislation are closed. As such, the enforcing agency should be capable of sanctioning failures to provide the required data, and prosecuting violations of the equal pay for equal work principle.
Finally, it is important to note that testing the application of the equal pay for equal work principle at the company level through an EPRR system, while useful for identifying potential causes of the gender pay gap and the existence of gender disparities within companies, is just a first step in a longer and more complex process. Once disparities are identified, both companies and enforcing agencies should follow up with additional research and analysis to determine whether these disparities are linked to discriminatory practices, and what type of remedial options could be adopted.
References
- Equileap. 2021. Gender equality global report & ranking. Equileap Research Paper. Available at: https://equileap.com/wp-content/uploads/2021/07/Equileap_Global_Report_2021.pdf
- Geostat. 2020. Business Sector in Georgia. Geostat. Available at: https://www.geostat.ge/media/35014/Krebuli-2020.pdf%20
- UN Women. 2020. Analysis of the Gender Pay Gap and Gender Inequality in the Labor Market in Georgia. Tbilisi: UN Women. Available at: https://georgia.unwomen.org/en/digital-library/publications/2020/03/analysis-of-the-gender-pay-gap-and-gender-inequality-in-the-labor-market-in-georgia
- UN Women. 2021. Assessment of the models for the implementation of the models for the implementation of the Equal Pay Review and Reporting (EPRR) methodology in Georgia. Tbilisi: UN Women. Available at: https://iset-pi.ge/en/publications/research-reports/3020-assessment-of-the-models-for-the-implementation-of-eprr-methodology-in-georgia
- WGEA. 2019. International Gender Equality Reporting Schemes. Workplace Gender Equality Agency Annual Report. Available at: https://www.wgea.gov.au/publications/international-gender-equality-reporting-schemes
- WEF. 2021. Global Gender Gap Report 2021. The World Economic Forum. Geneva, Switzerland. Available at: https://www.weforum.org/reports/ab6795a1-960c-42b2-b3d5-587eccda6023
Disclaimer: Opinions expressed in policy briefs and other publications are those of the authors; they do not necessarily reflect those of the FREE Network and its research institutes.
Vaccination Progress and the Opening Up of Economies
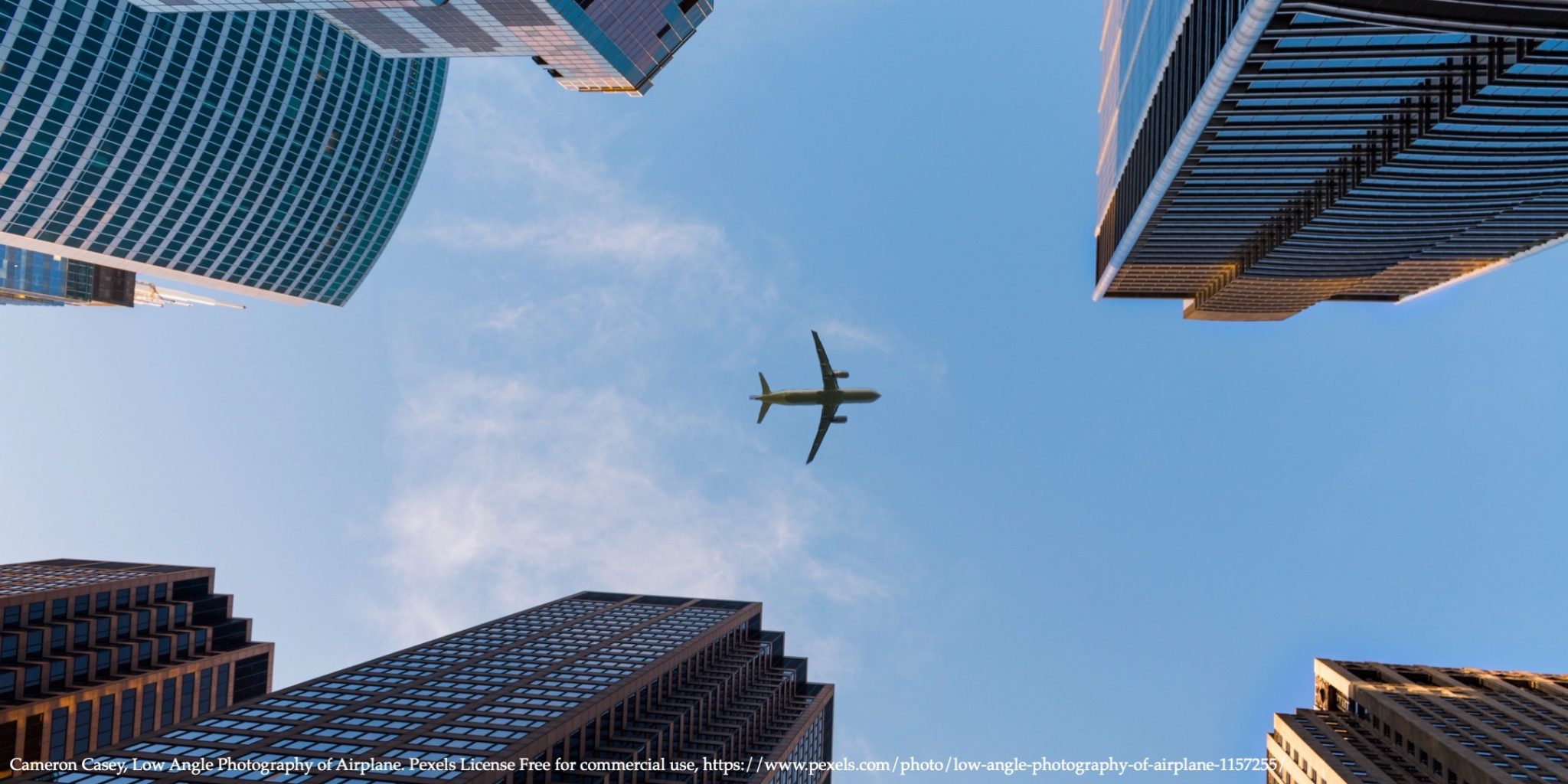
In this brief, we report on the FREE network webinar on the state of vaccinations and the challenges ahead for opening up economies while containing the pandemic, held on June 22, 2021. The current state of the pandemic in each respective country was presented, suggesting that infection rates have gone down quite substantially recently in all countries of the network, except in Russia which is currently facing a surge in infections driven by the delta-version of the virus. Vaccination progress is very uneven, limited by lacking access to vaccines (primarily Ukraine and Georgia) and vaccine scepticism among the population (primarily in Russia and Belarus but for certain groups also in Latvia, Poland and to some extent Sweden). This also creates challenges for governments eager to open their societies to benefit their economies and ease the social consequences of the restrictions on mobility and social gatherings. Finally, the medium to long term consequences for labour markets reveal challenges but also potential opportunities through wider availability of work–from-home policies.
Background
In many countries in Europe, citizens and governments are starting to see an end to the most intense impact of the Covid-19 pandemic on their societies. Infection and death rates are coming down and governments are starting to put in place policies for a gradual opening up of societies, as reflected in the Covid-19 stringency index developed by Oxford University. These developments are partially seasonal, but also largely a function of the progress of vaccination programs reaching an increasing share of the adult population. These developments, though, are taking place to different degrees and at different pace across countries. This is very evident at a global level, but also within Europe and among the countries represented in the FREE network. This has implications for the development within Europe as a whole, but also for the persistent inequalities we see across countries.
Short overview of the current situation
The current epidemiological situation in Latvia, Sweden, Ukraine, and Georgia looks pretty similar in terms of Covid-19 cases and deaths but when it comes to the vaccination status there is substantial variation.
Latvia experienced a somewhat weaker third wave in the spring of 2021 after being hit badly in the second wave during the fall and winter of 2020 (see Figure 1). The Latvian government started vaccinating at the beginning of 2021, and by early June, 26% of the Latvian population had been fully vaccinated.
Sweden, that chose a somewhat controversial strategy to the pandemic built on individual responsibility, had reached almost 15 thousand Covid-19 deaths by the end of June of 2021, the second highest among the FREE network member countries relative to population size. The spread of the pandemic has slowed down substantially, though, during the early summer, and the percentage of fully vaccinated is about to reach 30% of the population.
Figure 1. Cumulative Covid-19 deaths
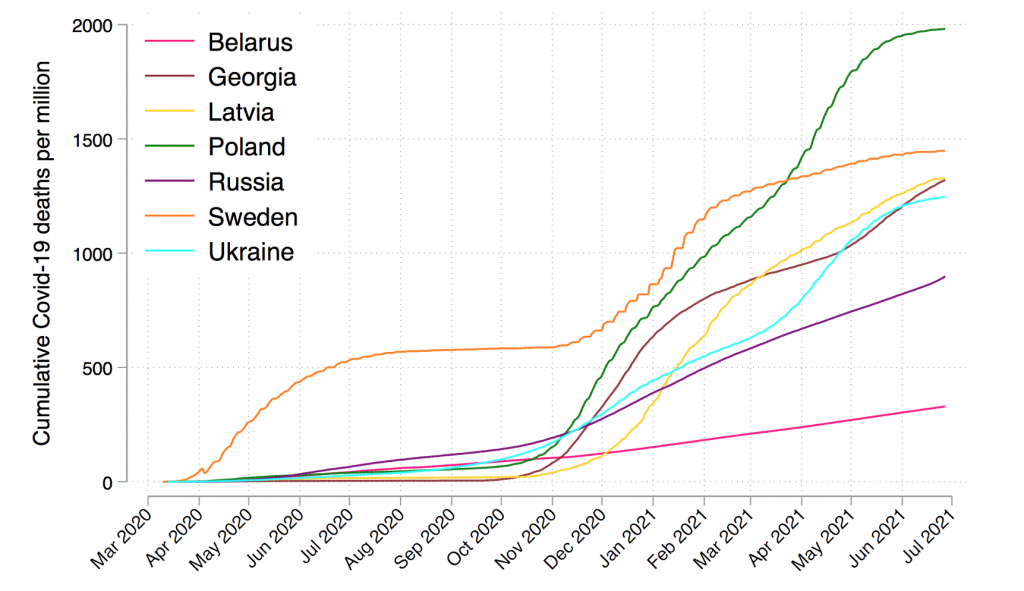
Source: Aggregated data sources from the COVID-19 Data Repository by the Center for Systems Science and Engineering (CSSE) at Johns Hopkins University, compiled by Our World in Data.
Following a severe second wave, the number of infected in Ukraine started to go down in the winter of 2020, with the total deaths settling at about 27 thousand in the month of February. Then the third wave hit in the spring, but the number of new daily cases has decreased again and is currently three times lower than at the beginning of the lastwave. However, a large part of the reduction is likely not thanks to successful epidemiological policies but rather due to low detection rates and seasonal variation.
In June 2021, Georgia faces a similar situation as Ukraine and Latvia, with the number of cumulative Covid-19 deaths per million inhabitants reaching around 1300 (in total 2500 people) following a rather detrimental spring 2021 wave. At the moment, both Georgia and Ukraine have very low vaccination coverage relative to other countries in the region(see Figure 5).
In contrast to the above countries, Russia started vaccinating early. Unfortunately, the country is now experiencing an increase in the number of cases (as can be seen in Figure 2), contrary to most other countries in the region. This negative development is likely due to the fact that the new Covid-19 delta variant is spreading in the country, particularly in Moscow and St. Petersburg. Despite the early start to vaccinations, though, the total number of vaccinated people remains low, only reaching 10.5% of the population.
Figure 2. New Covid-19 cases
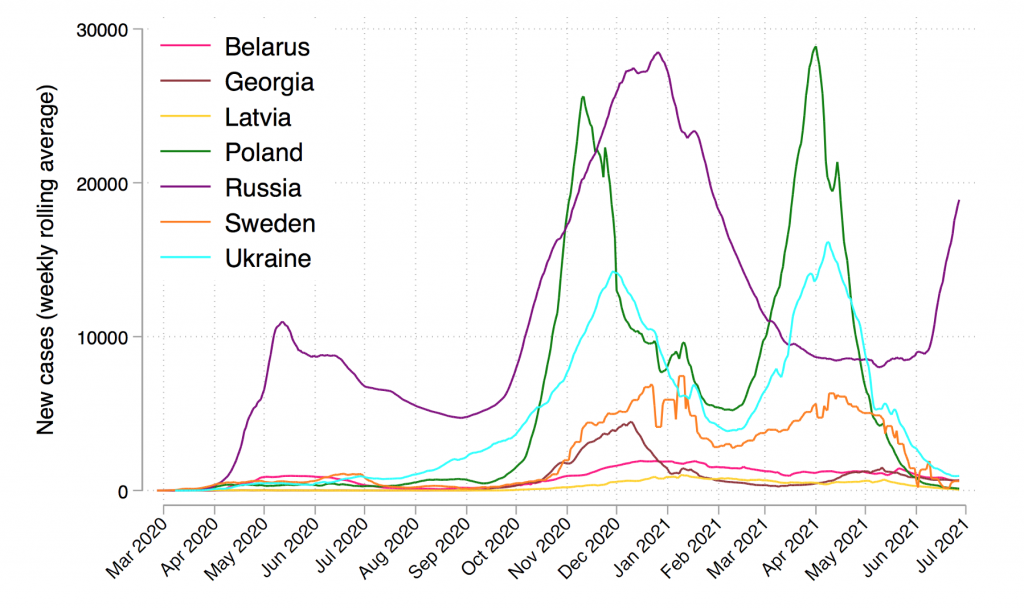
Source: Aggregated data sources from the COVID-19 Data Repository by the Center for Systems Science and Engineering (CSSE) at Johns Hopkins University, compiled by Our World in Data.
In some ways similar to Sweden, the government of Belarus did not impose any formal restrictions on individuals’ mobility. According to the official statistics, in the month of June, the rise in the cumulative number of covid-19 deaths and new daily infections has declined rapidly and reached about 400 deceased and 800 infections per one million inhabitants, respectively. Vaccination goes slowly, and by now, around 8% of the population has gotten the first dose and 5% have received the second.
There were two major waves in Poland during the autumn 2020 and spring 2021. In the latter period, the country experienced a vast number of deaths. As can be seen in Figure 3, the excess mortality P-score – the percentage difference between the weekly number of deaths in 2020-2021 and the average number of deaths over the years 2015-2019 – peaked in November 2020, reaching approximately 115%. The excess deaths numbers in Poland were also the highest among the FREE Network countries in the Spring of 2021, culminating at about 70% higher compared to the baseline. By mid-June, the number of deaths and cases have steeply declined and 36% of the country’s population is fully vaccinated.
Figure 3. Excess deaths
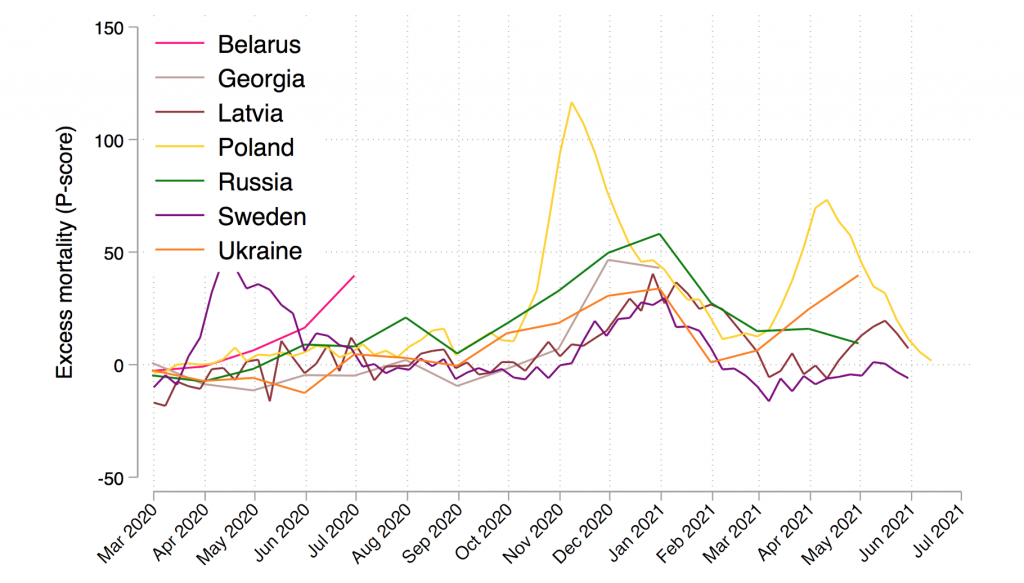
Source: Human Mortality Database & World Mortality Dataset compiled by Our World in Data.
Turning to the economy, after a devastating year, almost all countries are expected to bounce back by the end of 2021 according to the IMF (see Figure 4). Much of these predictions build on the expectations that governments across the region will lift Covid-19 restrictions. These forecasts may not be unrealistic for the countries where vaccinations have come relatively far and restrictions have started to ease. However, for countries where vaccination rates remain low and new variations of the virus is spreading, the downside risk is still very present, and forecasts contain much uncertainty.
Figure 4. GDP-growth
Vaccination challenges
Since immunization plays such a central role in re-opening the economy and society going back to normal, issues related to vaccinations were an important and recurring topic at the event. The variation in progress and speed is substantial across the countries, though.
Ukraine and Georgia are still facing big challenges with vaccine availability and have fully vaccinated only 1.3% and 2.3% of the population by the end of June, respectively. Vaccination rates have in the recent month started to pick up, but both countries face an uphill battle before reaching levels close to the more successful countries.
Figure 5. Percent fully vaccinated
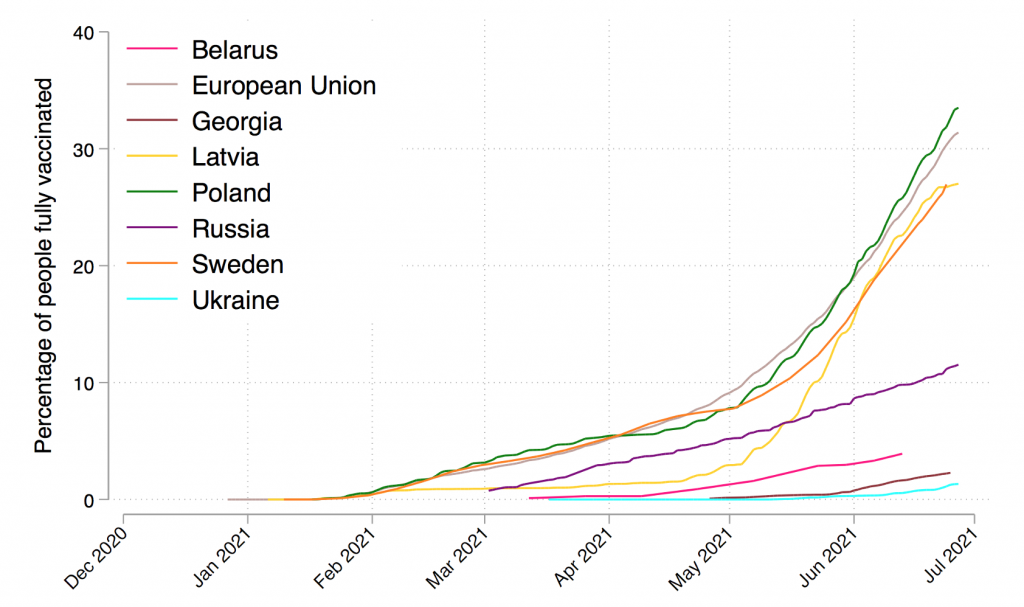
Source: Official data compiled by Our World in Data. See full source list at: https://github.com/owid/covid-19-data/blob/master/public/data/vaccinations/locations.csv
Other countries a bit further ahead in the vaccine race are still facing difficulties in increasing the vaccination coverage, though not so much due to lack of availability but instead because of vaccine skepticism. In Belarus, a country that initially had bottleneck issues similar to Ukraine and Georgia, all citizens have the opportunity to get vaccinated. However, Lev Lvovskiy, Senior Research Fellow at BEROC in Belarus, argued that vaccination rates are still low largely because many Belarusians feel reluctant towards the vaccine at offer (Sputnik V).
This vaccination scepticism turns out to be a common theme in many countries. According to different survey results presented by the participants at the webinar, the percentage of people willing or planning to get vaccinated is 30% in Belarus and 44% in Russia. In Latvia, this number also varies significantly across different groups as vaccination rates are significantly lower among older age cohorts and in regions with a higher share of Russian-speaking residents, according to Sergejs Gubins, Research Fellow at BICEPS in Latvia.
Webinar participants discussed potential solutions to these issues. First, there seemed to be consensus that offering people the opportunity to choose which vaccine they get will likely be effective in increasing the uptake rate. Second, governments need to improve their communication regarding the benefits of vaccinations to the public. Several countries in the region, such as Poland and Belarus, have had statements made by officials that deviate from one another, potentially harming the government’s credibility with regards to vaccine recommendations. In Belarus, there have even been government sponsored disinformation campaigns against particular vaccines. In Latvia, the main problem is rather the need to reach and convince groups who are generally more reluctant to get vaccinated. Iurii Ganychenko, Senior Researcher at KSE in Ukraine, exemplified how Ukraine has attempted to overcome this problem by launching campaigns specifically designed to persuade certain age cohorts to get vaccinated. Natalya Volchkova, Director of CEFIR at NES in Russia, argued that new, more modern channels of information, such as professional influencers, need to be explored and that the current model of information delivery is not working.
Giorgi Papava, Lead Economist at ISET PI in Georgia, suggested that researchers can contribute to solving vaccine uptake issues by studying incentive mechanisms such as monetary rewards for those taking the vaccine, for instance in the form of lottery tickets.
Labour markets looking forward
Participants at the webinar also discussed how the pandemic has affected labour markets and whether its consequences will bring about any long-term changes.
Regarding unemployment statistics, Michal Myck, the Director of CenEA in Poland, made the important point that some of the relatively low unemployment numbers that we have seen in the region during this pandemic are misleading. This is because the traditional definition of being unemployed implies that an individual is actively searching for work, and lockdowns and other mobility restrictions have limited this possibility. Official data on unemployment thus underestimates the drop in employment that has happened, as those losing their jobs in many cases have left the labour market altogether. We thus need to see how labor markets will develop in the next couple of months as economies open up to give a more precise verdict.
Jesper Roine, Professor at SITE in Sweden, stressed that unemployment will be the biggest challenge for Sweden since its economy depends on high labor force participation and high employment rates. He explained that the pandemic and economic crisis has disproportionately affected the labor market status of certain groups. Foreign-born and young people, two groups with relatively high unemployment rates already prior to the pandemic, have become unemployed to an even greater extent. Many are worried that these groups will face issues with re-entering the labour market as in particular long-term unemployment has increased. At the same time, there have been more positive discussions about structural changes to the labour market following the pandemic. Particularly how more employers will allow for distance work, a step already confirmed by several large Swedish firms for instance.
In Russia, a country with a labour market that allowed for very little distance work before the pandemic, similar discussions are now taking place. Natalya Volchkova reported that, in Russia, the number of vacancies which assumed distance-work increased by 10% each month starting from last year, according to one of Russia’s leading job-search platforms HeadHunter. These developments could be particularly beneficial for the regional development in Russia, as firms in more remote regions can hire workers living in other parts of the country.
Concluding Remarks
It has been over a year since the Covid-19 virus was declared a pandemic by the World Health Organization. This webinar highlighted that, though vaccination campaigns in principle have been rolled out across the region, their reach varies greatly, and countries are facing different challenges of re-opening and recovering from the pandemic recession. Ukraine and Georgia have gotten a very slow start to their vaccination effort due to a combination of lack of access to vaccines and vaccine skepticism. Countries like Belarus and Latvia have had better access to vaccines but are suffering from widespread vaccine skepticism, in particular in some segments of the population and to certain vaccines. Russia, which is also dealing with a broad reluctance towards vaccines, is on top of that dealing with a surge in infections caused by the delta-version of the virus.
IMF Economic Outlook suggests that most economies in the region are expected to bounce back in their GDP growth in 2021. While this positive prognosis is encouraging, the webinar reminded us that there is a great deal of uncertainty remaining not only from an epidemiological perspective but also in terms of the medium to long-term economic consequences of the pandemic.
Participants
- Iurii Ganychenko, Senior Researcher at Kyiv School of Economics (KSE/Ukraine)
- Sergejs Gubins, Research Fellow at the Baltic International Centre for Economic Policy Studies (BICEPS/ Latvia)
- Natalya Volchkova, Director of the Centre for Economic and Financial Research at New Economic School (CEFIR at NES/ Russia)
- Giorgi Papava, Lead Economist at the ISET Policy Institute (ISET PI/ Georgia)
- Lev Lvovskiy, Senior Research Fellow at the Belarusian Economic Research and Outreach Center (BEROC/ Belarus)
- Jesper Roine, Professor at the Stockholm Institute of Transition Economics (SITE / Sweden)
- Michal Myck, Director of the Centre for Economic Analysis (CenEA / Poland)
- Anders Olofsgård, Deputy Director of SITE and Associate Professor at the Stockholm School of Economics (SITE / Sweden)
Disclaimer: Opinions expressed in policy briefs and other publications are those of the authors; they do not necessarily reflect those of the FREE Network and its research institutes.
Laissez-faire Covid-19: Economic Consequences in Belarus
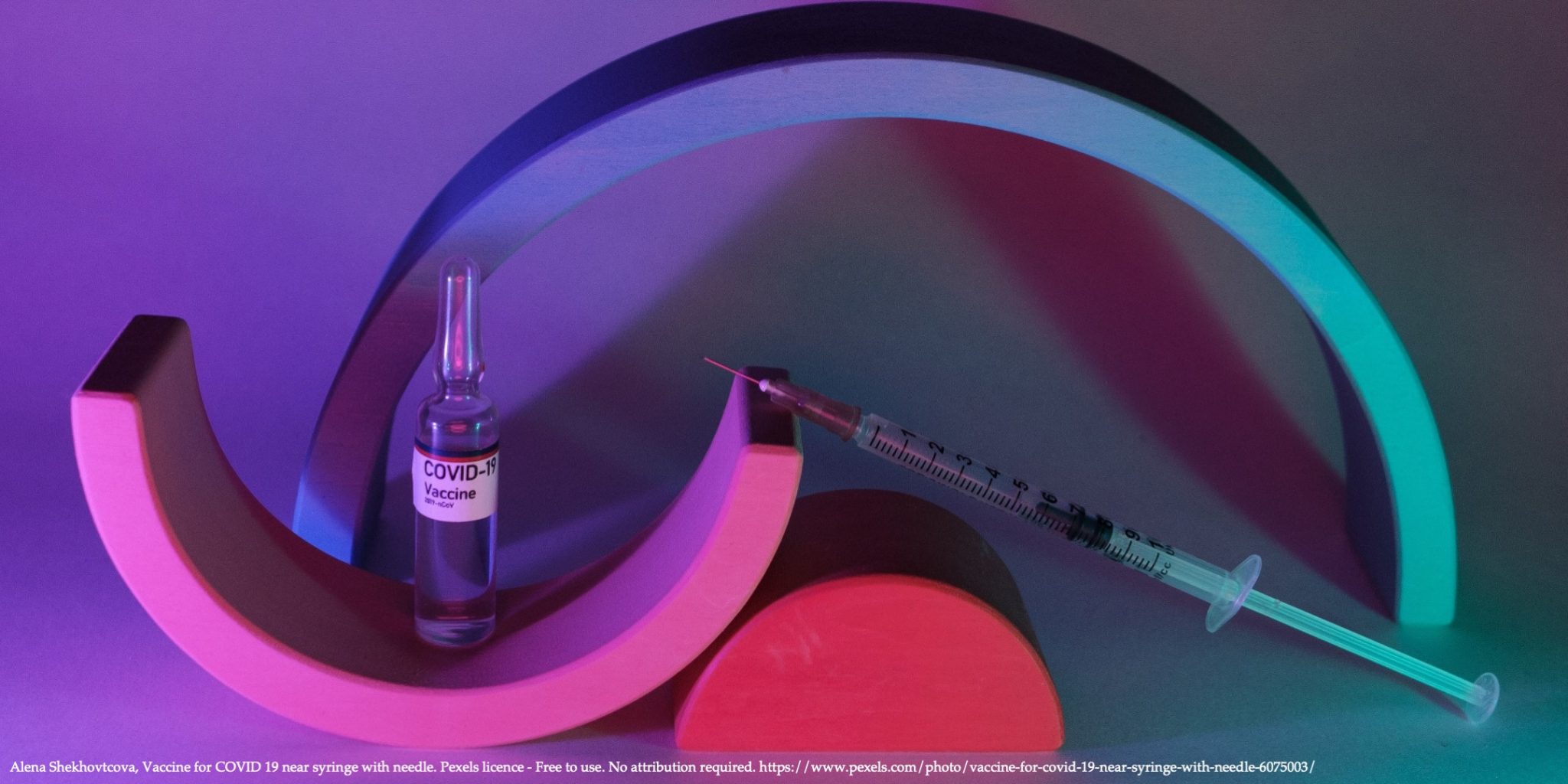
Despite its traditional paternalistic role, the Belarusian government chose minimal reaction to the Covid-19 pandemic. No meaningful economic or social measures were taken in response to the pandemic. We explore a unique dataset to document how major Covid-related shocks affected the earnings of Belarusians in 2020. We utilize the differential timing and sectoral effects of the shocks to identify the impact of Covid-19 on individual socioeconomic outcomes. Not surprisingly, we find that Covid-related shocks increase the probability of an income reduction. This effect is most pronounced for those employed in the private sector. In the absence of a social security net, vulnerable groups had to cope with the economic consequences of the pandemic on their own.
Introduction
Belarus had its first official case of Covid-19 registered on February 27 and its first death on March 31. At first, the increase in newly registered cases was slower than in most other countries, but at the beginning of April Belarus started to catch up. The peak of the first wave was recorded on May 18 with 943 new daily cases. According to the official statistics, the second wave started in September 2020 and was much more severe than the first one, reaching 1,890 new daily cases by the end of December.
Belarusian authorities did not undertake any substantial interventions, such as lockdowns, to fight the spread of the pandemic. Nevertheless, there were several other key mechanisms through which Covid-19 affected the Belarusian economy. The population’s reaction to the risks of contamination led to a substantial fall in mobility that resulted in decreased sales in retail and services requiring physical interaction. For example, sales in the restaurant industry decreased by 20% in 2020. Lockdowns in major international trade partners such as Russia have led to a decrease in demand for Belarusian exports of goods and transportation services. In the face of these economic challenges, the government focused its attention on supporting full employment and production in state-owned enterprises while ignoring the rest of the economy.
In this brief, we present evidence of the economic effects of Covid-19 in Belarus. We employ a unique dataset on socioeconomic outcomes collected by BEROC to study how individuals are affected by Covid-related shocks in mobility and exports. In order to isolate the effects of these shocks on the well-being of Belarusians, we exploit their timing and sectoral differences.
Measuring Covid-related Shocks
Figure 1 depicts changes in the Yandex self-isolation index which measures the use of Yandex services, including Yandex traffic monitoring and customer mobility compared to the average pre-pandemic day (Yandex DataLens, 2021). Individual everyday mobility started to decline in mid-March, and as the first wave of the pandemic gained momentum, mobility reached its lowest point at the end of April. It started to decline again in November-December 2020 following the second wave.
Figure 1. Yandex self-isolation index in Belarus, 2020
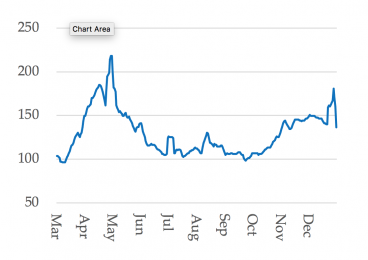
Source: Yandex. The average value during 24 Feb-8 March 2020 set to 100. Seven-day rolling average.
Belarus is a small and open economy with Russia as its main trading partner. The lockdown in Russia that lasted from the end of March until mid-May along with the spring lockdowns in Europe caused a major contraction in external demand for Belarusian goods. Figure 2 shows total physical exports and non-energy physical exports in 2020. The largest difference between total and non-energy exports can be observed in January, February, and March during which Russia and Belarus had an oil-supply dispute. To focus on the effects of the pandemic we use non-energy physical exports to approximate Covid-related exogenous shocks to the economy.
Figure 2. Physical export indices, Belarus
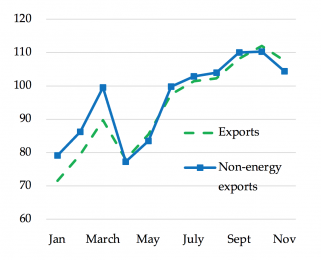
Source: Belstat. December 2019=100.
Income Dynamics
To measure the impact of Covid-19 on Belarusian society, BEROC, in cooperation with the marketing and opinion research company SATIO, conducted a series of online surveys representative of the urban population of Belarus (Covidonomics, 2021). The five waves of the 2020 survey were carried out on April 17-22, May 8-11, June 8-15, September 11-16, and November 25-30.
Respondents were asked about recent changes to their income, and also to specify the reasons for income reduction (if this was the case), including depreciation of the ruble, salary cut, furlough, etc. Figure 3 depicts the percentage of individuals who reported an income reduction in the previous month for reasons other than currency depreciation by sector of employment. The income reductions peaked in April-June, with the situation relatively stabilizing by September.
Figure 3. Income dynamics by sector
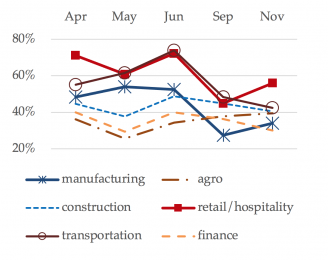
Percentage of respondents reporting income reductions in the previous month for reasons other than currency depreciation, Source: BEROC/SATIO data
The fact that the share of respondents reporting termination peaked at 2.9% in May indicates that firms did not use employment reduction to adapt to the pandemic environment. A big share of respondents employed in the service sector reported domestic demand contraction (fewer orders/clients) as a key factor for their income reduction. The industries that took the hardest hit were hospitality-retail and transportation. In early spring, manufacturing appeared to be one of the most affected industries. However, as exports started to recover in June, the share of manufacturing workers that reported an income reduction decreased significantly, becoming one of the lowest across industries.
Identifying the Effects of Covid-19 Shocks
In this section, we estimate the probability of facing a reduction in individual income as well as the likelihood of being furloughed due to the Covid-19 pandemic.
In 2020, the Belarusian economy suffered due to the oil-supply dispute with Russia, the Covid-19 pandemic, and the national political crisis. To isolate the effects of Covid-19 from those driven by the oil dispute and the political crisis, we add interactions between Covid-related shocks and dummies indicating industries affected by those shocks. This implies three interactions with different binary indicators: exports and manufacturing, exports and transportation, and mobility and hospitality/retail.
To estimate these effects, we use a fixed-effects probit regression controlling for sector of employment, education, age, and gender.
Table 1. Probability of income reduction and furlough
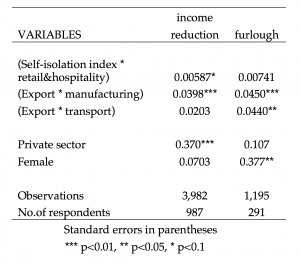
Source: Own estimates from BEROC/Satio data. Controls include age, sector of employment, and education level.
Table 1 shows that individuals employed in the hospitality and retail industry face higher risks of an income reduction due to decreased mobility caused by self-isolation behavior. A 10-percentage-point increase in the self-isolation index is associated with a 1.3 percentage point increase in the probability of income reduction for those employed in the retail and hospitality industry. The interaction term between exports and the manufacturing dummy also appears to be statistically significant for various specifications. A 10-percentage-point decline in physical volumes of exports is associated with a 8.6 percentage point increase in the probability of income reduction for manufacturing workers.
Notably, the private sector employment coefficient shows strong statistical significance which highlights the choice of the authorities to support SOEs, with little to no support for the private sector. Being employed in the private sector increases the probability of facing an income reduction by 7.9 percentage points.
The Gender Dimension
Despite concerns that women experience larger economic losses due to consequences of the pandemic (Dang and Nguyen, 2021; Alon et al., 2020b), we do not find a statistically significant effect of gender in our sample. In particular, our results offer no evidence of women being more likely to experience an income reduction during the pandemic, similar to findings in Germany (Adams-Prassl et al. 2020c).
While job losses were uncommon during the Covid-19 crisis in Belarus, being furloughed was one of the most common reasons for an income reduction (11.3% of respondents reported being furloughed in May). We also investigate the separate channels through which individuals lose income due to the Covid-related shocks. Notably, the only channel of income reduction that is more prevalent among women than men is through furlough. This finding is consistent with Adams-Prasslet al. (2020a) who argue that this discrepancy can be explained by gender differences in childcare responsibilities.
Conclusion
Belarus is close to unique in having almost no government response to the Covid-19 pandemic. Despite the absence of lockdowns and other restrictions, the Belarusian economy has experienced several Covid-associated shocks. Due to the economy’s openness to trade, it was seriously affected by export contractions. Belarusians have voluntarily reduced their mobility to minimize health risks which has affected the hospitality and retail industry.
We utilize the differential timing and sectoral impact of Covid-related shocks to estimate the pandemic’s effect on the socioeconomic outcomes of individuals. By using a unique dataset, we find evidence that the pandemic increased the likelihood of income reductions for Belarusians, mainly due to the effects of decreased mobility and fall in exports. We also find that those employed in the private sector were more likely to suffer from negative shocks, reflecting the policy choice of the Belarusian government to only provide economic support to the state sector. Finally, we show that, while women are as likely as men to see their income reduced, they are significantly more likely to be furloughed.
Many Belarusians saw their well-being deteriorating as a result of the Covid-19 pandemic. In the absence of unemployment benefits and other social protection mechanisms (Umapathi, 2020), those economically affected had to bear the cost of the shocks on their own.
References
- Adams-Prassl, A., Boneva, T., Golin, M., and Rauh, C. (2020a). Furloughing. Fiscal Studies, 41(3):591–622.
- Adams-Prassl, A., Boneva, T., Golin, M., and Rauh, C. (2020b). Inequality in the impact of the coronavirus shock: Evidence from real time surveys. Journal of Public Economics, 189:104245.
- Covidonomics project (2020). BEROC and Satio. http://covideconomy.by/
- Dang, H.-A. H. and Nguyen, C. V. (2021). Gender inequality during the Covid-19 pandemic: Income, expenditure, savings, and job loss. World Development, 140:105296.
- Umapathi, N. (2020). Social protection system in Belarus: perspective. Bankovskiy Vestnik, (3):75–80. (in Russian).
- Yandex (2021) Yandex DataLens, https://datalens.yandex.ru/
Disclaimer: Opinions expressed in policy briefs and other publications are those of the authors; they do not necessarily reflect those of the FREE Network and its research institutes.
Labor Market Adaptation of Internally Displaced People: The Ukrainian Experience
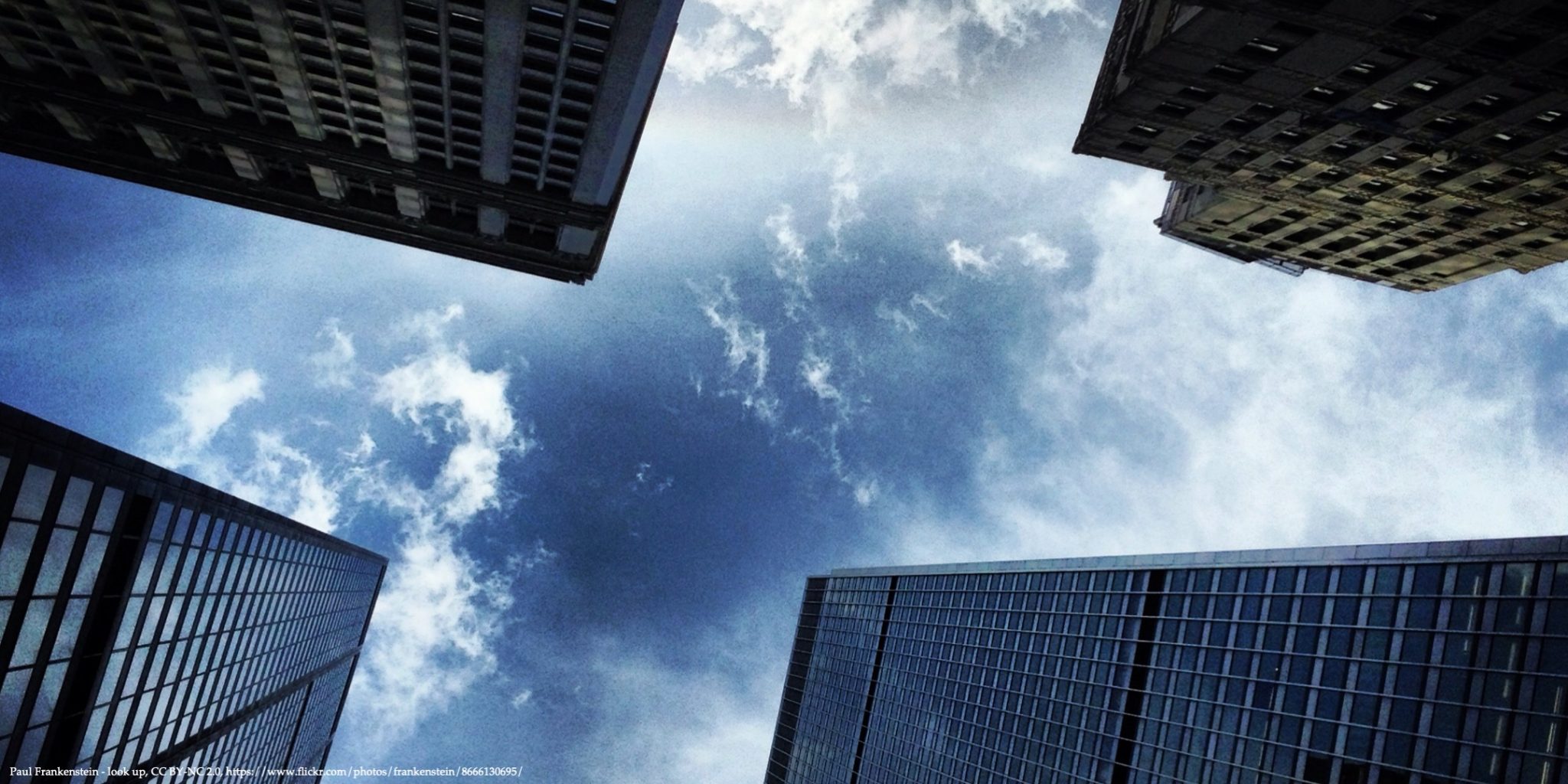
This brief is based on research that investigates the probability of employment among displaced and non-displaced households in a region bordering territory with an ongoing military conflict in Eastern Ukraine. According to the results, internally displaced persons (IDP) are more educated, younger and more active in their job search than locals. Nevertheless, displaced individuals, particularly males, have experienced heavy discrimination. After controlling for personal characteristics, the structure of the household, location, non-labour incomes and endogeneity of displacement, IDP males are 17% less likely to be formally employed two years after resettlement than locals.
Internally displaced persons in Ukraine
In 2014, 23 years after independence, Ukraine suddenly found itself among the top-ten of countries with the largest internally displaced population. During the period 2014–2016, 1.8 million persons registered as internally displaced. Potentially, about 1 million more reallocated to Russia and about 100,000 to other countries nearby, where they sought refugee or labour migrant status (Smal, 2016).
The Ministry of Social Policy of Ukraine (MSPU) has regularly published very general reports on displaced persons. According to these reports, at the end of February 2016, the internally displaced persons in Ukraine included 22,000 individuals from Crimea and over 1.7 million citizens from Eastern Ukraine. These are mostly individuals who registered as IDPs to qualify for financial assistance from the state and some non-monetary benefits. Among them, 60% are retired people, 23.1% are individuals of working age, 12.8% are children and 4.1% are people with disabilities (Smal and Poznyak, 2017). In fact, the MSPU registers not only displaced persons but also those who de facto live in the occupied territories and occasionally travel to territories controlled by the Ukrainian authorities to receive their pension or social benefits (so called ‘pension tourism’). On the other hand, some IDPs did not register either to avoid bureaucracy or because they were unable to prove their status due to lack of documents. Recent publications that are based on surveys portray a more balanced distribution: 15% are retired people, 58% are individuals of working age, 27% are children and 13% are people with disabilities (IOM and the Ukrainian Centre for Social Reforms, 2018).
Only limited information is available about IDPs’ labour market activity. According to the State Employment Service (SES), between March 2014 and January 2016, only 64,300 IDPs or 3.75% referred to the SES for assistance (Smal and Poznyak, 2017). On the one hand, this figure reflects the relatively low reliance of displaced Ukrainians on the SES services in their job search. On the other hand, the geographical variation in the share of SES applicants suggests that Ukraine’s IDPs who moved further from the war zone and their homes were more active in trying to find a job.
Data
Our primary data were collected in June–August 2016 by REACH and provided by the Ukraine Food Security Cluster (UFSC) as a part of the needs assessment in Luhansk and Donetsk oblasts of Ukraine – two regions that were directly affected by the conflict. These two regions have hosted roughly 53% of all IDPs in Ukraine (Smal and Poznyak, 2017). We argue that households that did not move far from the place of conflict are most likely to be driven by conflict only, while long-distance movers may combine economic and forced displacement motives.
The data set offers information on 2500 households interviewed in 233 locations and is statistically representative of the average household in each oblast. It includes respondents currently living in their pre-conflict settlements (non-displaced, NDs) and respondents who report a different place of residence before the conflict (IDPs). The IDP group comprises individuals with registered and unregistered status and from both sides of the current contact line. The non-IDP group includes only households living on the territory controlled by the Ukrainian Government that did not move after the conflict had started.
Our sample covers 1,135 displaced households that came from 131 settlements. Most of the reallocations took place in early summer 2014 with the military escalation of the conflict in Eastern Ukraine. Thus, the average duration of displacement up to the moment of the interview was 637 days (or 21 months). This is a sufficiently long period for adaptation and job search. However, there is enough variation in this indicator – some families left as early as March–April 2014, while others were displaced in June 2016, just a few days before the interviews started.
Results
Simple comparison shows that heads of displaced households are on average almost four years younger than those of non-displaced households (Table 1). In terms of education, displaced households are found to be more educated than non-displaced households, as there are significantly more IDP household heads with tertiary education and significantly fewer individuals with only primary, secondary or vocational degrees. In particular, 37% of IDP household heads hold a university degree compared with 22% of household heads among the local population. This seems to suggest positive displacement selection. IDPs are slightly more likely to be headed by females and unmarried persons, although these differences are statistically insignificant. Displaced households include more children aged under five (0.35 vs. 0.22 children per non-displaced household) and 6 to 17 years (0.42 vs. 0.34, respectively) and fewer members aged over 60 years (0.58 vs 0.66, respectively). There is no difference in the number of working-age adults or disabled individuals per household among IDPs and non-IDPs. The average household size is statistically similar for the groups (2.74 vs. 2.65 persons per IDP and non-IDP household, respectively).
Table 1. Selected descriptive statistics
Internally displaced households | Non- displaced households | |
Household head employed | 0.43*** | 0.48*** |
Household head characteristics | ||
Age (years) | 48.10*** | 52.85*** |
Male | 0.49 | 0.52 |
Education | ||
vocational | 0.42*** | 0.49*** |
university | 0.37*** | 0.22*** |
Household characteristics | ||
Size (persons) | 2.74 | 2.65 |
Number of children 0-5 | 0.35*** | 0.21*** |
Number of children 6-17 | 0.42*** | 0.34*** |
Number of members 60+ | 0.58** | 0.66** |
IDP payments | 0.50*** | 0*** |
Humanitarian assistance | 0.78*** | 0.28*** |
There are further differences in the types of economic activity and occupations among IDPs and non-IDPs. Prior to the conflict, displaced respondents were more likely (than non-displaced persons) to be employed as managers or professionals and less likely to hold positions as factory or skilled agricultural workers. This result also speaks in favor of a positive displacement selection story.
As expected, the conflict has had a negative effect on human capital in the government controlled areas of Donetsk and Luhansk regions. We observe some deskilling at the time of the interviews, which is especially pronounced for IDPs. In particular, the share of managers among the IDPs had reduced from 12% to 5% and that of technicians from 15% to 12%, while the proportion of service and sales employees had increased from 10% to 13%, that of factory workers from 11% to 15% and that of skilled agricultural workers from 2% to 6%.
Considering the economic activity in the current location, we can note that on average the heads of displaced households are 5% less likely to be employed than those of non-displaced households (43% vs. 48%, respectively). In both groups, a large share of respondents report difficulties in their job search, but IDPs are 13% more likely to experience this problem. They report changing their pre-conflict occupation three times more often than non-IDPs (37% vs. 11%).
Government and non-government assistance may also drive the differences in employment. Economic theory states that individuals are less likely to work if they have some backup in the form of non-labour earnings. Financial support and humanitarian assistance are widely used to smooth a displacement shock. At the same time, improperly designed assistance schemes may reduce the stimulus to search for a job.
IDPs are 9% less likely to include earnings in their household’s top three main sources of income than the non-displaced population (46% vs. 55%, respectively), meaning that they rely more on various social payments and pensions. In addition, displaced households may be slightly more reluctant to search for a job due to displacement assistance from the government (received by 50% of IDPs compared with 0% for non-IDP households), although the amounts are quite modest. According to the existing legislation, IDPs can receive regular monthly state payments and one-time state payments. Regular monthly payments can be received by any IDP and cannot exceed UAH 3,000 (~$111) for an ordinary household, UAH 3,400 for a household with disabled people and UAH 5,000 (~$185) for a household with more than 2 children. Eligibility and the size of the one-time payment are determined by the local government. In the data set, 95% of IDPs receive less than UAH 3,000 while the 2016 average monthly wage was UAH 6,000 in Donetsk and UAH 4,600 in Luhansk regions.
In addition, IDPs are three times more likely to receive humanitarian assistance (78% vs. 28% among displaced and non-displaced persons, respectively). This support includes mostly food and winterisation items but also cash (26% among displaced vs. 12% among non-displaced assistance receivers). On the other hand, to cover reallocation and adaptation costs, some IDPs use their financial reserves, and as a result they are by 10 p.p. more likely to report no or already depleted savings. This may increase their stimulus to engage in a more active job search.
After taking into account the observed and unobserved differences between the groups as well as controlling for the location fixed effect, we find that the difference in the probability of employment between displaced and non-displaced persons increases from a casually observed slit of 5% to a chasm of 17.3%. This result suggests that IDPs are [negatively] discriminated despite being younger, more educated, skilled and more ‘able’ in the labour market. Specifically, 7 out of 17 p.p. (41% of the gap) are due to the variation in observed household head characteristics and family composition, while unobserved displacement-related features (such as attitude towards change, activism, mental and physical ability to reallocate) account for 5 p.p. (29%) of the gap. Controlling for particularities of a current location does not substantially affect the estimated differences.
Figure 1. Main results
We re-estimate these regressions using an employment indicator that includes both formal and informal employment (as defined by the respondents), accounting for occasional and irregular employment, including subsistence agricultural work. Since informal work is more common among IDPs, this definition of employment leads to a reduction in the average casually observed gap from 5% to 3%. However, after controlling for all the factors, we obtain the same result – a 17.8% difference between displaced and non-displaced households.
Conclusion
Policy makers and international donors should not be misled by the seemingly comparable probability of employment among IDPs and non-IDPs based on simple statistics. The average 0–5% difference in unconditional employment rates conceals the actual 17% gap in the likelihood of having a job. The contribution of unobserved displacement-related factors in hiding the true gap is large, especially for males seeking formal employment. Without adjusting for it, we would underestimate the real difference in employment probability by one-third to one-half.
Our study produces firm evidence that displaced individuals in Ukraine, particularly males, have been discriminated against in terms of employment. Our results further suggest that male heads of displaced households experience more discrimination in the formal labour market, while the situation is the opposite for females, who are more likely to face unequal treatment in the informal sector. Policy makers and volunteers should take this difference into account in the adaptation of male- and female-headed households.
Humanitarian assistance to displaced individuals was found to have no negative effect on their employment, which suggests that it is provided in an effective manner. Thus, this tool can be used to mitigate the discrimination.
References
- IOM and the Ukrainian Centre for Social Reforms. (2018). ’National Monitoring System Report on the Situation of Internally Displaced Persons.’
- Smal, V. and O. Poznyak. (2017) ‘Internally displaced persons: social and economic integration in hosting communities’, PLEDDG Project.
- Smal, V. 2016. ’Внутрішньо Переміщені Особи: Соціальна та економічна інтеграція в приймаючих громадах.’
- Vakhitova, H. and P. Iavorskyi, “Employment of Displaced and Non-displaced Households in Luhansk and Donetsk Oblasts”, Europe-Asia studies, (forthcoming).
Disclaimer: Opinions expressed in policy briefs and other publications are those of the authors; they do not necessarily reflect those of the FREE Network and its research institutes.
Individual Retirement Timing in Russia: Implications for Pension Age
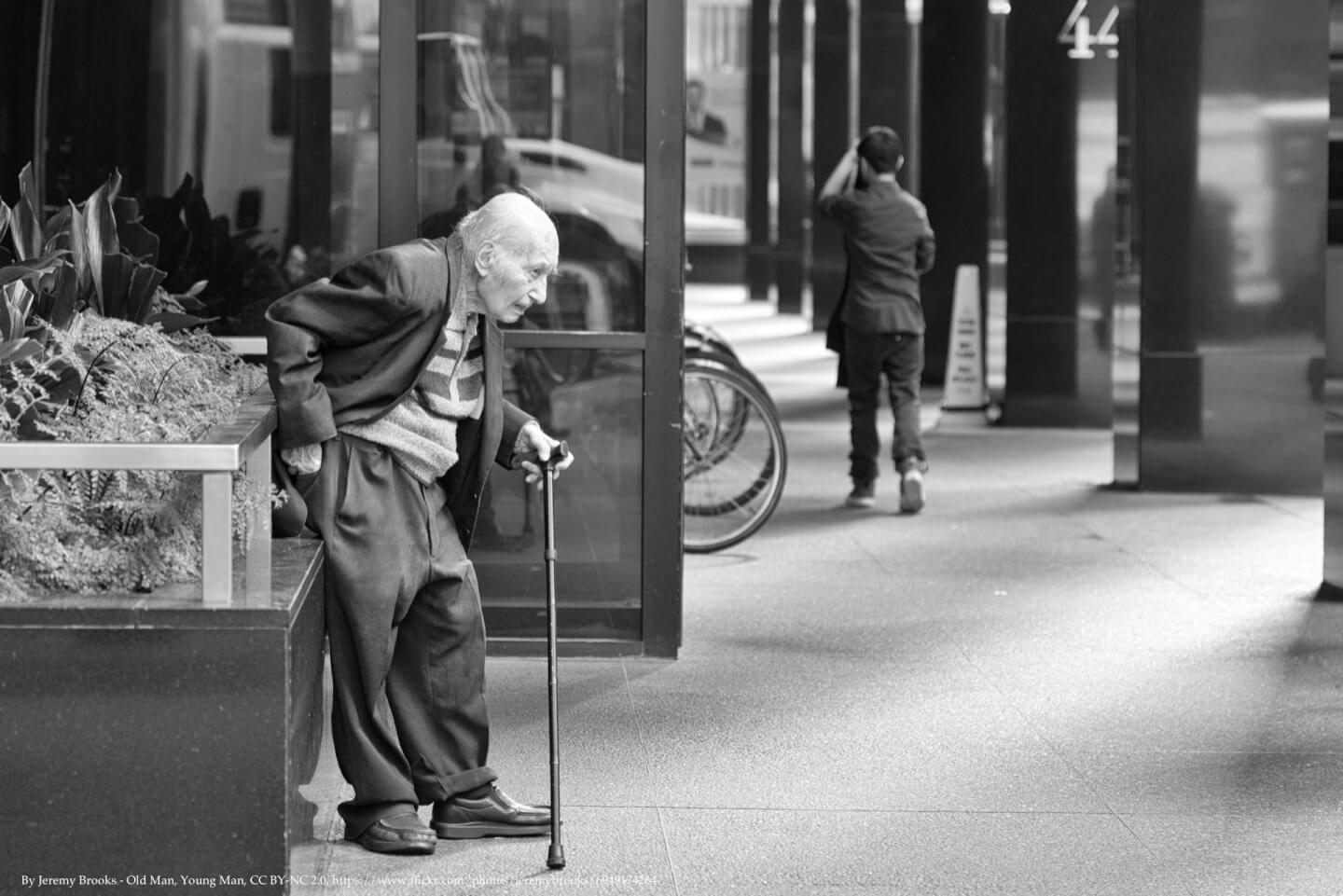
This policy brief summarizes the findings in a paper where individual exit trajectories of Russians from the labor market to economic inactivity are examined using survival analysis methods based on the Russian Longitudinal Monitoring Survey for 1995-2015. Among other results, the analysis shows that the statutory retirement age has a significant impact on the time of exit from the labor market for both men and women, but the effect is very high for women. This is an interesting and unexpected result, given no penalty for working beyond the pension age of those already retired, the five-year difference in statutory retirement age between males and females, and the low pension age in Russia on an international scale. This questions the painlessness of rising the retirement age for women, should the decision finally be taken.
An ageing population, combined with a slowdown in economic growth, challenges the Russian public finances with an increased deficit of the Pension fund. In addition, the persistently negative natural population growth against the backdrop of ageing has predetermined a decline in the working-age population in the foreseeable future. Older cohorts are therefore becoming a potentially attractive source to increase the size of the labor force. All this has actualized the discussion about the need to increase the Russian retirement age (see, for instance, Maleva and Sinyavskaya, 2010). However, little is known about the labor market situation of older age groups and, in particular, about the process of their exit from the labor market
The Russian pension system, unlike the pension systems of many developed countries, hardly penalizes continuation of work after reaching retirement age and documenting a pension (working pensioners lose only pension indexation). The changes in pension law that have entered into effect since 2015 encourage continued work without recourse to retirement, but there have been few responses to the innovation so far. Coupled with the low pension replacement rate (i.e., the proportion of wages substituted by pension), this makes the process of leaving the labor market nontrivial, since a large number of people of retirement age remain on the labor market after reaching retirement age.
Denisova (2017) examines individual exit trajectories of Russians from the labor market to pension-age economic inactivity applying survival analysis to the Russian Longitudinal Monitoring Survey (RLMS-HSE). The major research questions are the following: What determines the length of stay of older age groups in the Russian labor market? What is the role of the statutory retirement age in this process?
Data and research methodology
The RLMS-HSE for the period of over 20 years, from 1995 to 2015, is the empirical basis of the research (http://www.cpc.unc.edu/rlms). I limit the sample to age 45-72 as there is practically no retirement by age before age 45, and 72 years is the upper boundary of the working age definition internationally accepted by statisticians. I exclude from the sample those who are on retirement and did not work or seek work for the entire period of observation, since their decision to end working activity remained outside the observation period.
An episode in the survival analysis of exit from the labor market into pension-age inactivity is an episode of working life. The analytical time in this case is the age of the respondent. The failure event (the moment of exit from the labor market to pension-age economic inactivity) is defined by the simultaneous fulfillment of three conditions: the respondent does not work, does not look for a job, and receives retirement pension. Only the final exits from the labor market into inactivity are considered, while temporary exits are disregarded.
I evaluate proportional hazard models, which suggest that exogenous economic factors shift the baseline hazard function (which reflects the average entire sample hazard rate at each age) proportionally. A semi-parametric Cox model specification with robust errors clustered at individual level is used.
The vector of explanatory characteristics includes education; marital status; experience in the labor market (work at an enterprise with a state share; entrepreneurship versus work for wages); health characteristics (subjective and objective); settlement type; and attainment of statutory retirement age. In all cases, I control for the year of the survey.
Given the differences in the behavior of men and women in the labor market, the regression analysis is run separately for the subsamples of men and women. The statistical significance of the differences in returns to factors between men and women is tested based on the results of the full sample regression with interaction terms.
Averaged process of exit from the labor market
The averaged process of leaving the labor market pending on age is conveniently described through so-called Kaplan-Mayer’s survival function (an estimate of the survival process). As seen from Figure 1, the process of exit prior to age 55 for women and 60 for men is very slow, while the rate of exit becomes almost permanent and slows down after 70 years. Men stay in the labor market longer: 25% of women leave the labor market at the age of 58 years, whereas for men this age is 60. The threshold of 75% of the sample that left the labor market is reached in the sample of women by the age of 70, and 71 for men.
Determinants of exit
The analysis of older cohorts’ exit from the labor market via survival methods confirms important determinants of the process, previously identified in literature. The impacts of health and of financial incentives are in this group of results.
Figure 1. Survival functions, men and women
Source: Author’s calculations based on RLMS-HSE 1995-2015 data
Health status is the key factor for men’s exit into inactivity: the exit to inactivity is accelerated by 71 percentage points for males with bad health, whereas for women this factor is statistically irrelevant.
A higher per capita household income is correlated with later exit from the labor market. A higher income from the main place of employment has no statistically significant effect when we control for household income and is at an extended boundary (15%) of statistical significance if we do not. Both variables indirectly reflect the pension replacement rate, and I interpret the results as an indirect confirmation that workers at the top part of the income distribution, being inadequately insured by the pension system, remain on the labor market longer.
The identified peculiarities of the exit to pension-age inactivity of the Russian elderly are of major interest. Unlike many developed countries, only highly skilled persons remain in the labor market longer than others, while the behavior of middle-skilled groups, and skilled and unskilled workers does not statistically differ between them.
Employment at state-owned enterprises slows down women’s exit to inactivity but is not significant for men. Self-employment and entrepreneurship prolong the presence in the labor force, by 41 percentage points for women.
The regression analysis demonstrates that the statutory retirement age has a significant impact on the time of exit from the labor market for both men and women, and the effect is significantly higher for women: the hazard rate of inactivity rises by 63 percentage points when a woman reaches 55 years, and by 25% when a man reaches 60. For men, an effect comparable in size is the self-assessment of health as poor.
Discussion
The results, on the one hand, confirm those for developed countries: health status is the key factor for men’s exit into inactivity, and financial motives have a significant impact. At the same time, the peculiarities of the Russian labor market are reflected in a differing labor market exit process of various professional groups, in the sense that self-employment and entrepreneurship and work at state enterprises postpone exit into inactivity. The high sensitivity of women to the statutory retirement age, which by 2.5 times exceeds the sensitivity of men, is one of the new and unexpected results, taking into account that the statutory retirement age for women in Russia is very low by international standards. This questions the painlessness of rising the retirement age for women, should the decision finally be taken. Indeed, given the very low pension age for females, an (gradual) increase in the retirement age for women would seem not to raise strong objections. However, our result testifies that the normative border of the retirement age has a decisive influence on women’s choice of time of exit from the labor market, even under control (as far as data permits) on differences in education, situation in the labor market and family circumstances. In this situation, the process of rising the retirement age, if such a decision is taken, can be rather painfully accepted by those who so strongly focus on its current meaning in their life plans.
References
- Denisova, Irina, 2017, “Exit of senior age cohorts from the labor market: survival analysis approach” – forthcoming in Population and Economics.
- Maleva T.M., Sinyavskaya O.V., 2010 “Raising the retirement age: pro et contra, Journal of the New Economic Association, No. 8, pp. 117-139.