Tag: Ukraine
Do Condominiums Pay Less for Heating?
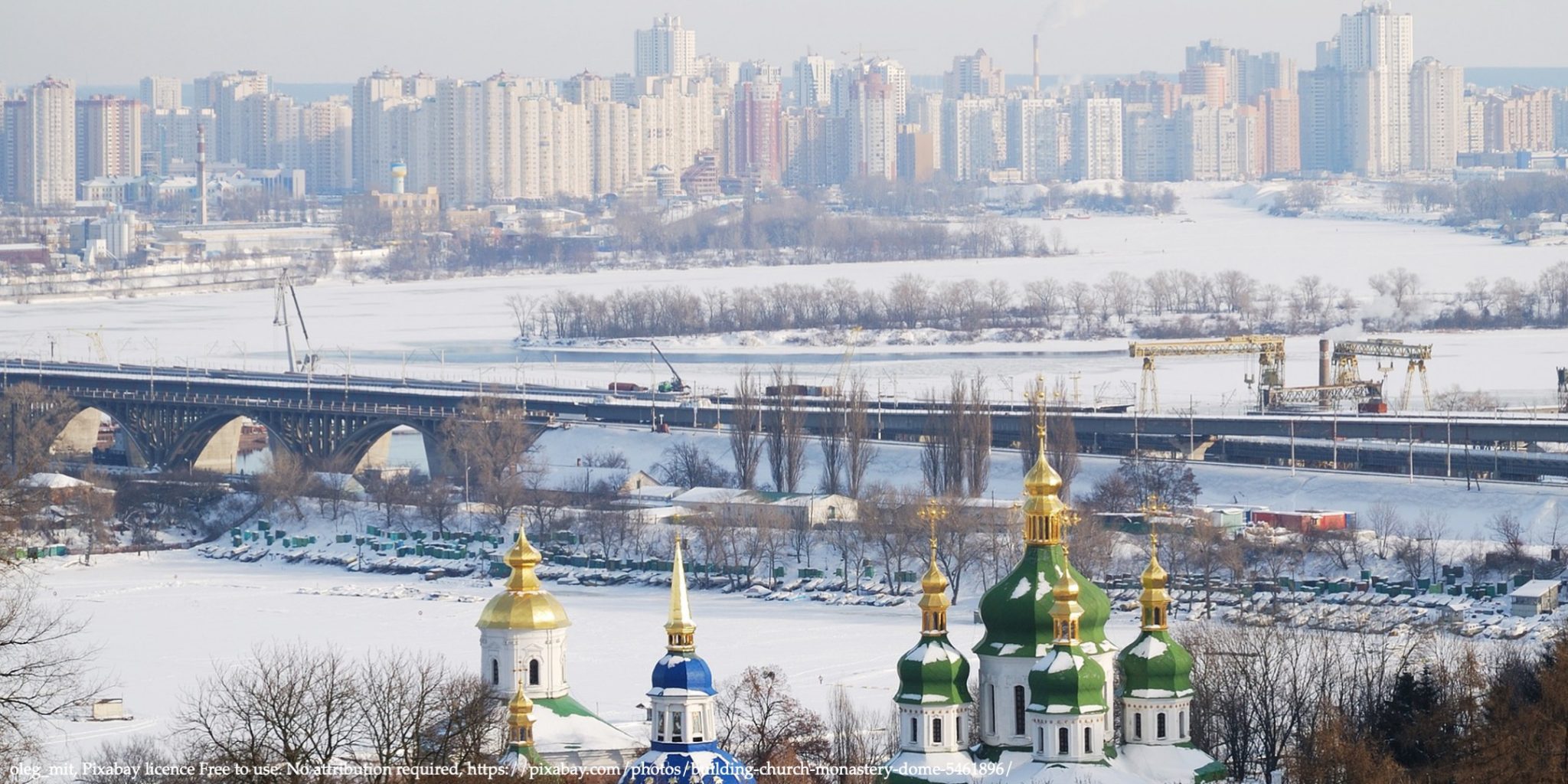
In Ukraine, a widely shared perception is that housing utility costs are too high. In this policy brief, we study if these costs can be alleviated by introducing a modern form of housing management practice, condominiums. We find that condominiums in old houses (built before 1991) pay 22% less for heating compared to old non-condominiums. Among new houses (built after 1991), we find that condominiums pay 29% less for heating. Considering the dynamics of condominium formation in 2018-2020, old houses do not show any significant immediate effect of condominium formation on heating costs relative to that of non-condominiums. However, condominium formation among new houses leads to a relative 18% decrease in heating costs. In addition, among condominiums in old houses, participation in an overhaul co-financing program is associated with a 15% lower heating bill. The immediate effect of the program in 2018-2020 is a 16% relative decrease in heating costs for old condominiums and 37% – for new ones.
Heating Costs and Condominiums
In recent years, the cost of housing utilities has been a common concern among Ukrainians. According to a recent survey, 80% of Ukrainians believe that tariffs on utilities are too high.
The form of housing management is a factor that could affect utility costs. Experiences from Slovakia, Hungary, Poland, and Romania in the 1990s suggest that state-owned housing maintenance companies are often associated with inefficient management. Residential buildings that are owned and managed collectively by its dwellers (hereafter referred to as condominiums) are more likely to choose a more efficient private housing maintenance company (Banks, O’Leary et. al., 1996). For instance, in Slovakia’s second-largest city, Kosice, one-third of houses that were privatized in the 1990s chose private maintenance companies with competitive prices. Residents perceived the services as “far more effective” (ibid).
This brief summarizes our analysis of the relationship between heating costs and the form of housing management in Ukraine. Analyzing a large sample of houses in Kyiv, we show that condominiums are associated with lower heating costs, both among the older houses, built before Ukrainian independence in 1991, and among newer houses.
Types of Housing Management Practices in Ukraine
The different housing management practices in Ukraine can be roughly divided into three types. The most commonly used practice is when housing maintenance is carried out by a municipally owned company (commonly referred to as ZhEK – “zhilischno-eksplotazionnaja kontora”, housing maintenance office). Usually, houses that have the ZhEK-type management were built before Ukrainian independence and have kept this practice since Soviet times. The second practice is when housing maintenance is done by a private company affiliated with the building developer. This management type is usually used by houses built after Ukrainian independence that did not form condominiums. These two practices are similar in the sense that dwellers are not directly involved in the decision-making, all decisions are made by the municipal or private company, respectively.
The third type of housing management practice, relatively new for Ukraine, is condominium ownership (the Ukrainian term for it is ОСББ, translated as “Association of Co-owners of Multi-Apartment House”). In a condominium, unlike in the previous two types, the house is managed collectively by the dwellers; in particular, they have the freedom to choose and/or change utility providers, invest in major overhaul, and participate in co-financing programs.
Houses with Condominiums Pay Less for Heating
In our analysis, we use monthly data on housing costs between 2018 and 2020 collected from the Ukrainian municipal enterprise Kyivteploenergo. The data covers more than 70% of residential buildings in Kyiv and includes information on heating costs per square meter, whether or not the house is a condominium, and other house-characteristics (including the source of heating production; the presence of the meter; type of the meter number of service days per month; and share of heat consumption by legal entities).
In addition, we have information on the year of building construction retrieved from the real estate portal LUN, and condominium formation date between 2018-2020, as well as data on house participation in overhaul co-financing programs obtained from the Kyiv state administration.
Our final sample contains 7957 houses. Since we only are interested in apartment housing, we exclude residential buildings with an area below 500 m2, which would normally correspond to a small private house (these constitute only a small part of our sample). The share of condominiums in the sample is 11%, the share of houses with ZhEK is 81% and the share of houses managed by private companies is 8%.
Figure 1. Median costs for heating per m2 across housing management types and house age.
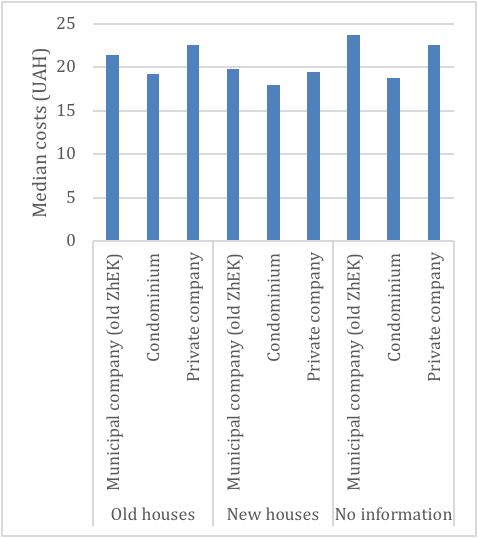
Source: Authors’ calculations. Old houses are those built before 1991, the year of Ukrainian independence, and new houses are built after 1991.
Figure 1 provides preliminary evidence towards our hypothesis, showing that the median heating costs are lower in condominiums, independent of the year of construction.
In our first econometric model, we use an OLS-approach to compare utility costs across different types of housing and management models, while controlling for a number of observable characteristics. We find that condominiums in old houses pay 22% less for heating than old non-condominium. Similarly, we find that condominiums in new houses pay 29% less for heating compared to new non-condominiums.
The lower heating costs observed in condominiums may have several explanations:
- First, condominium-type management could be more flexible in its response to weather conditions. Considering that they are profit-maximizing, heating providers in Ukraine tend to overheat houses during the heating season; it could be that condominiums reduce consumption of heating on the warmer days to a greater extent than other houses. In other words, condominiums could increase the efficiency of heating use.
- Second, it could be that condominiums have lower heating costs because they improve energy efficiency, for example, by installing individual heating points (an automatized unit transferring heat energy from external heat networks to the house heating, hot water supply, ventilation, etc.), new windows, or even insulating the house.
Is There an Immediate Effect?
The next step in our econometric analysis is to study the effect of condominium formation during 2018-2020. Here, we investigate whether non-condominium houses that became condominiums experienced changes in heating costs by utilizing a fixed-effects regression model. This approach not only allows us to assess the immediate effect of condominium formation but also controls for unobservable house-specific characteristics that are constant over time, such as differences in building materials.
For new houses, we find that condominium formation decreases heating costs by 18% compared to other new houses. For old houses, we find that the corresponding effect is statistically insignificant.
This estimation only evaluates the effect of condominium formation in a relatively short timeframe, between 2018 and 2020. While the data coverage does not allow us to give a precise quantitative assessment for a long-run effect, we argue that the positive impact of condominium formation on heating costs could potentially be higher in the longer-run. Indeed, our previous OLS estimation assesses the average utility costs for all condominiums in the sample (including those formed prior to 2018). It shows that the gap in heating costs between all condominiums and non-condominiums is higher than the corresponding gap derived from our fixed-effects estimation (22% for the old houses and 29% – for the new ones). While this difference in results can be driven by several reasons (e.g., fixed effect estimation taking into account unobservable house-specific characteristics), a stronger long-term effect could be among them.
Concerning the results for new vs. old houses, it might be the case that new houses are technically equipped to be more flexible when it comes to adjusting costs (e.g., are able to switch the heating on/off), while old houses might be inferior in this regard. If this is the case, old houses would only experience lower costs after some thermo-modernization, such as installing individual heating points.
Heating Costs and the Co-financing Program
Since 2015, the Kyiv city council offers a program that helps condominiums to finance major overhauls with the intent to improve the energy efficiency of the residential sector. Applicants compete in planning thermo-modernization projects where winning condominiums are awarded financing covering 70% of the overhaul cost.
Our results show that for old houses with condominiums, those who at some point participated in the co-financing program pay on average 15% less for heating compared to non-participants. The corresponding effect for new houses with condominiums is not significantly different from zero.
However, the immediate effect of program participation is present in both new and old houses with condominiums. Old and new condominiums that took part in the program in 2018-2019 experienced an immediate reduction in heating costs by 16% and 37% respectively.
Figure 2. The number of houses participating in the 70/30 co-financing program across the years.
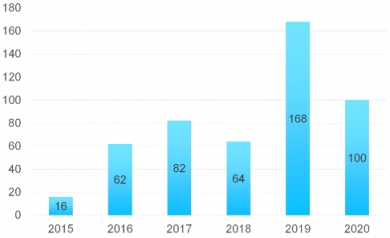
Authors’ calculations.
There are several potential explanations as to why we observe an immediate effect but no effect of ever participating in the program for the new houses with condominiums.
First, it could be that new houses with condominiums that are not participating in the program are investing in overhaul anyway, although somewhat delayed compared to investments made by participating new condominiums. The average difference in heating costs between participants and non-participants would then be visible in the short-run and fade away after a few years. If this is the case, the program is financing houses that would have invested in overhaul anyway, even without co-financing. This explanation is partly supported by the fact that the share of the new houses condominiums among participants is 32%, while the corresponding share is 15% among all houses. In other words, old houses with condominiums, that are usually in a worse condition, are underrepresented in the program.
If this is the case, the share of old houses with condominiums among participants should be increased. Given that the purpose of the program is to improve the energy efficiency of residential buildings, its efficient implementation implies encouraging overhauls in houses that are otherwise unable to fund it. In other words, the program should incentivize people living in energy-inefficient housing to form condominiums and undertake overhauls to improve their energy efficiency, rather than finance houses who are already doing well in that regard. To improve on such selection issues, the program could change the co-financing proportions, making participation more beneficial to old houses with condominiums, e.g. 80/20 – for old and 60/40 – for new condominiums.
Second, the new houses with condominiums that participate in the program might be in a much worse state before participation than those that do not. Program part-taking could make participants catch up to the average level of energy-efficiency (or perhaps do slightly better). If this is the case, the program fulfills its function in the sense that it targets the most energy-inefficient houses.
Government Policies That Should Be Changed
Above, we argue that the formation of condominiums leads to efficiency gains in energy use and cuts utility costs for dwellers. Given the design of the overhaul co-financing program, the Kyiv city council seems to recognize these benefits as well. However, there is a range of government policies currently in place that discourage people from condominium formation.
For example, there are cases when the government finances 100% of overhaul costs using a subvention (“subvention for socio-economic development”). In 2020, 17 houses in Kyiv got overhaul expenses funded by this type of subvention. At the same time, 85 houses that participated in the co-financing competition did not receive any state funding (there were 100 winners among 185 participants).
Considering that this type of subvention predominantly finances non-condominiums, we argue that this policy creates the wrong incentives. Dwellers will likely refrain from forming condominiums in the hope of eventually being selected for an overhaul fully financed by the state, instead of forming condominium and getting only part of overhauls expenses covered (70% of the overhaul funding if winning co-finance program competition, and no funding otherwise).
In addition, this subvention typically has a “pork-barrel” nature since it is often allocated to the constituencies of the ruling party’s MPs. State financed overhauls are often used as an advertisement tool to get popular support. This creates an additional problem in the sense that subvention is targeted to politically loyal regions and not necessarily to regions in need of support.
Along this line of reasoning, we suggest that this pork-barrel subvention should be cancelled and housing-overhauls should instead be funded through co-financing programs. The government should implement programs similar to the “70/30” and further encourage people to adopt condominium ownership.
Conclusion
Motivated by the common perception that utility costs are excessively high, we study one possible way of reducing the utility bill – condominium housing management.
Our analysis shows that old houses with condominiums pay 22% less for heating compared to old non-condominiums. For new houses, we find that condominiums pay 29% less in heating costs than non-condominiums. In addition, old houses with condominiums that participate in Kyiv’s co-financing program pay 15% less than other old condominiums. That is, condominium formation combined with the co-financing program could save more than one-third of a resident’s heating costs.
Our analysis suggests the following policy implications:
- Condominiums have a positive effect on energy efficiency, and utility cost savings, and should thus be promoted to the population as a preferable form of house management practice.
- State and municipal governments should provide incentives for condominium formation through, e.g., overhaul co-financing programs. Other state-provided forms of overhaul financing, such as pork-barrel subvention, should be cancelled.
- Co-financing programs should combine better targeting (e.g., to those houses that are in greater need of overhaul) with sufficient incentives for condominium formation.
References
- Hamaniuk, Oleksii; and Andrii Doschyn, 2020. “Let’s reduce the cost of heating by a third!” – ACMH and co-financing program for buildings”, https://voxukraine.org/en/let-s-reduce-the-cost-of-heating-by-a-third-acmh-and-co-financing-program-for-buildings/
- Banks, Christopher, Sheila O’Leary, and Carol Rabenhorst, 1996. Review of urban & regional development studies, vol. 8, issue 2. https://doi.org/10.1111/j.1467-940X.1996.tb00114.x
Disclaimer: Opinions expressed in policy briefs and other publications are those of the authors; they do not necessarily reflect those of the FREE Network and its research institutes.
How to Liberalise EU-Ukraine Trade under DCFTA: Tariff Rate Quotas
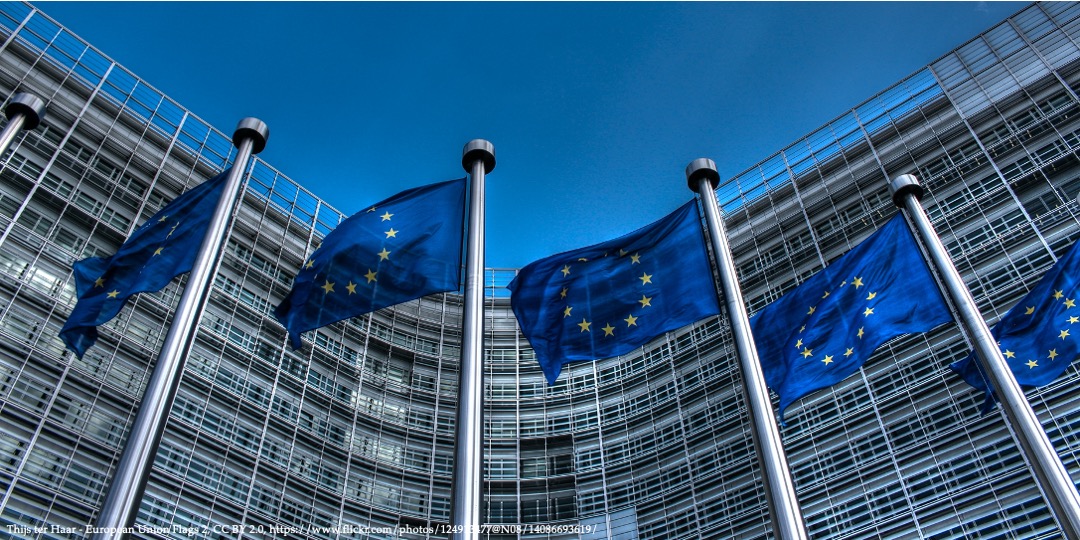
This policy brief focuses on trade relations between Ukraine and the EU amid preparations for the review of the Deep and Comprehensive Free Trade Agreement (DCFTA) due in 2021. In particular, it analyses Ukraine’s utilization of the DCFTA tariff rate quotas (TRQs) over 2016-2019. According to the results, Ukraine has been steadily increasing the level of TRQs usage – in terms of the number of utilized TRQs and export volumes within and beyond TRQs. For some DCFTA TRQs, total exports to the EU far outweigh quota volumes, while for other TRQs supply is limited by quota volume. The brief provides arguments and recommendations for the DCFTA TRQs update to increase Ukraine’s duty-free access to the EU market.
Why Update DCFTA TRQs for Ukraine?
EU-Ukraine trade under the Deep and Comprehensive Free Trade Agreement (DCFTA, in effect since January 1, 2016) progressed considerably. Ukraine’s exports of goods to the EU reached $20.8 billion in 2019 – a 54% increase compared to 2016 and a 24% increase compared to pre-crisis 2013.
According to the EU-Ukraine Association Agreement/DCFTA, the parties may initiate a review of its provisions in five years from its implementation – in 2021. So far, both governments confirmed their readiness to start such negotiations next year.
Ukraine advocates for further trade liberalisation with the EU through reducing the existing tariff and, most importantly, non-tariff barriers. This is an imperative for maintaining positive trade dynamics and providing new impetus to deepening bilateral economic integration.
Updating duty-free tariff rate quotas (TRQs) under the DCFTA is at the top of the EU-Ukraine 2021 negotiations agenda. Current quota volumes are based on outdated statistics, as it has been 10 years since the DCFTA negotiations (2008-2011).
Many TRQs are too low in terms of Ukraine’s current export and production capacities. For example, Ukraine’s total exports of grains (annual averages) increased from 19 million tons in 2008-2010 to 42.3 million tons in 2016-2018. Honey exports increased from 5.9 thousand tons in 2008-2010 to 58 thousand tons in 2016-2018. As a result, some TRQs are fully exhausted in the first days or months of the year.
High competition for access to duty-free quota volumes is a barrier first of all for SMEs that cannot compete effectively for it with large companies, while out-off-quota tariffs may be too restrictive for them.
Ukraine’s TRQs Utilisation During 2016-2019
DCFTA TRQs grant partial liberalisation of market access to the EU. Zero tariff rates are only applied to a specified quantity of imported goods inside a TRQ, while beyond TRQ imports to the EU are dutiable on a regular basis (subject to third-country tariff rates).
The EU applies TRQs for 36 groups of agro-food products originated in Ukraine plus 4 additional TRQs for certain product groups (in total 40 TRQs under DCFTA) – see Table 1. Ukraine applies TRQs for 3 groups of products plus 2 additional TRQs.
By the level of utilisation, TRQs fall into three groups: 1) fully utilised. They, in turn, can be divided into TRQs with and without over-quota supply; 2) partially utilised; and 3) not utilised.
The data indicate a general upward trend in Ukraine’s utilisation of TRQs under the DCFTA. In general, Ukrainian exporters utilised 32 TRQs in 2019 (80%) comparing to 26 TRQs in 2016 (65%).
Figure 1. Number of DCFTA TRQs utilized by Ukraine during 2016-2019.
Table 1 shows Ukraine’s utilization of 40 DCFTA TRQs over 2016-2019 – in tons and %. The main findings include:
The number of fully exhausted TRQs has been increasing. In 2019, Ukraine filled up 12 TRQs including honey; processed tomatoes; wheat; maize; poultry meat; barley groats and flour, other cereal grains; sugars; grape and apple juice; butter and dairy spreads starches; starch processed; as well as malt-starch processed products. For 9 of them, Ukraine’s supplies exceeded TRQs volumes.
The number of partially utilized TRQs increased from 16 in 2016 to 20 in 2019. In 2018-2019, Ukraine began using new TRQs such as fermented-milk processed products; malt-starch processed products; sugar syrups. High TRQs utilization rates (over 80%) in 2019 were observed for malt and wheat gluten; cereal processed products; eggs (main); barley, barley flour and pellets.
Moreover, Ukraine increased utilisation of TRQs for processed products. For example, utilisation of a TRQ for cereal processed products increased from 2.7% in 2016 to 99.5% in 2019. This signifies the growing ability of Ukrainian producers to comply with the EU food safety requirements and standards for processed products. Exports of processed starch increased significantly in 2019 and exceeded TRQ volume by a lot.
Ukraine’s utilisation of some TRQs has decreased. For example, a TRQ for oats gradually decreased from 100% in 2016 to 31% in 2019 due to a decrease in total exports and domestic production of oats in Ukraine during this period. Low utilisation of other TRQs may also be attributed to high price competition and quality requirements in the EU, complex quota allocation procedure, etc.
The number of not utilized TRQs decreased from 14 in 2016 to 8 in 2019. For instance, no exports within TRQs were observed for beef, pork, sheep meat, as Ukraine has not yet been authorized to export these meat products to the EU.
Moreover, since October 2017, Ukraine has been able to use provisional TRQs that were granted by the EU as autonomous trade measures (ATM) for 3 years. They increased duty-free access for 8 groups of Ukrainian products – in addition to the relevant DCFTA TRQs. So far, Ukraine fully utilises 5 ATM TRQs including honey; processed tomatoes; barley groats and meal, cereal grains otherwise worked; wheat, flour and pellets; maize, flour and pellets.
Total Exports to the EU vs Duty-Free Exports Within TRQs
For most fully utilized DCFTA TRQs, Ukraine’s total exports of the covered products exceeded TRQ volumes during 2016-2019. Considerable over-quota supply occurred for: honey; processed tomatoes; barley groats and meal, cereal grains; apple and grape juice; maize, flour and pellets; poultry meat; wheat, flour and pellets; sugars; butter and dairy spreads; starch processed.
For instance, over-quota exports of processed tomatoes from Ukraine to the EU in 2019 (31.2 thousand t) more than doubled the quota volumes (10,000 t of the DCFTA TRQ and 3,000 t of the provisional ATM TRQ). See Figure 2 for more examples.
Figure 2. Ukraine’s exports to the EU within and beyond certain TRQs, 2016-2019.
Increasing exports beyond TRQs indicate significant demand for these Ukrainian products in the EU, and their competitiveness in terms of price and quality on the EU market.
It also signifies that volumes of these fully utilised DCFTA TRQs with increasing over quota exports are rather low in terms of Ukraine’s export and production potential. Therefore, these TRQs are the primary candidates for updating.
At the same time, for certain DCFTA TRQs (malt-starch processed products; starch, malt and wheat gluten), exports to the EU were about 100% of TRQ volume but did not go far beyond. This may indicate a significant restrictive impact of those TRQs and out-of-quota tariffs for Ukrainian exports. These TRQs also need to be further analysed and revised.
Тable 1. Utilisation of DCFTA tariff rate quotas by Ukraine, 2016-2019.
2016 | 2019 | |||||
Quota name | Quota volume | Utilised | Quota volume | Utilised | ||
t | t | % | t | t | % | |
“First-come, first-served” method for TRQ allocation | ||||||
Sheep meat | 1500 | 0 | 0,0% | 1950 | 0 | 0,0% |
Honey | 5000 | 5000 | 100% | 5600 | 5600 | 100% |
Garlic | 500 | 49 | 9,8% | 500 | 393 | 78,6% |
Oats | 4000 | 4000 | 100% | 4000 | 1239 | 31,0% |
Sugars | 20070 | 20070 | 100% | 20070 | 20070 | 100% |
Other sugars | 10000 | 5929 | 59,3% | 16000 | 1006 | 6,3% |
Sugar syrups | 2000 | 0 | 0,0% | 2000 | 7 | 0,4% |
Barley groats and meal, cereal grains otherwise worked | 6300 | 6300 | 100% | 7200 | 7200 | 100% |
Malt and wheat gluten | 7000 | 7000 | 100% | 7000 | 6319 | 90,3% |
Starches | 10000 | 1898 | 19,0% | 10000 | 10000 | 100% |
Starch processed | 1000 | 0 | 0,0% | 1600 | 1600 | 100% |
Bran, wastes and residues | 17000 | 7286 | 42,9% | 20000 | 14467 | 72,3% |
Mushrooms main | 500 | 0 | 0,1% | 500 | 0 | 0,0% |
Mushrooms additional | 500 | 0 | 0,0% | 500 | 0 | 0,0% |
Processed tomatoes | 10000 | 10000 | 100% | 10000 | 10000 | 100% |
Grape and apple juice | 10000 | 10000 | 100% | 16000 | 16000 | 100% |
Fermented-milk processed products | 2000 | 0 | 0,0% | 2000 | 866 | 43,3% |
Processed butter products | 250 | 0 | 0,0% | 250 | 0 | 0,0% |
Sweetcorn | 1500 | 13 | 0,9% | 1500 | 23 | 1,5% |
Sugar processed products | 2000 | 340 | 17,0% | 2600 | 417 | 16,0% |
Cereal processed products | 2000 | 55 | 2,7% | 2000 | 1989 | 99,5% |
Milk-cream processed products | 300 | 73 | 24,4% | 420 | 9 | 2,2% |
Food preparations | 2000 | 5 | 0,3% | 2000 | 65 | 3,2% |
Ethanol | 27000 | 1889 | 7,0% | 70800 | 6083 | 8,6% |
Cigars and cigarettes | 2500 | 0 | 0,0% | 2500 | 0 | 0,002% |
Mannitol-sorbitol | 100 | 0 | 0,0% | 100 | 0 | 0,0% |
Malt-starch processed products | 2000 | 0 | 0,0% | 2000 | 1998 | 99,9%* |
Import licensing method for TRQ allocation | ||||||
Beef meat | 12000 | 0 | 0,0% | 12000 | 0 | 0,0% |
Pork meat main | 20000 | 0 | 0,0% | 20000 | 0 | 0,0% |
Pork meat additional | 20000 | 0 | 0,0% | 20000 | 0 | 0,0% |
Poultry meat and preparations main | 16000 | 16000 | 100% | 18400 | 18400 | 100% |
Poultry meat and preparations additional | 20000 | 8552 | 42,8% | 20000 | 9174 | 45,9% |
Eggs and albumins main | 1500 | 232 | 15,5% | 2400 | 2027 | 84,5% |
Eggs and albumins additional | 3000 | 0 | 0,0% | 3000 | 1891 | 63,0% |
Wheat, flours, and pellets | 950000 | 950000 | 100% | 980000 | 980000 | 100% |
Barley, flour and pellets | 250000 | 249460 | 99,8% | 310000 | 249250 | 80,4% |
Maize, flour and pellets | 400000 | 400000 | 100% | 550000 | 550000 | 100% |
Milk, cream, condensed milk and yogurts | 8000 | 0 | 0,0% | 9200 | 250 | 2,7% |
Milk powder | 1500 | 450 | 30,0% | 3600 | 560 | 15,6% |
Butter and dairy spreads | 1500 | 690 | 46,0% | 2400 | 2400 | 100% |
Source: European Commission, own calculations * Note: We consider 99.9% usage rate as fully utilized TRQ.
Conclusion
The EU and Ukraine confirmed their readiness to initiate the update of the DCFTA due in 2021. Ukraine is interested in increasing duty-free trade under DCFTA with the EU in line with the current state of Ukraine’s production and export capacities, as well as EU-Ukraine bilateral trade developments.
Although many DCFTA TRQs did not limit over-quota exports, Ukraine wants to revise DCFTA TRQs to secure permanent broader duty-free access to the EU market and reduce access barriers for SMEs (as SMEs are more affected by TRQs and other non-tariff barriers). So far, the EU temporarily increased certain TRQs in 2017 for three years as autonomous trade preferences for Ukraine. The primary candidates for the update should include DCFTA TRQs demonstrating high utilization rates, with or without over-quota supply (honey; processed tomatoes; barley groats and meal, cereal grains; apple juice; sugars; butter and dairy spreads; starch processed, etc.).
Amid future DCFTA update negotiations, Ukraine should conduct a detailed analysis for each DCFTA TRQ (taking into account temporary ATM quotas) to prepare its suggestions how and to what extent to liberalise them. It is worth considering different options of such liberalisation – by either increasing TRQs’ volumes or setting up preferential tariff rates for Ukraine instead, etc.
In the framework of the future negotiations with the EU, a special emphasis should be placed on increasing duty-free access for Ukrainian processed goods to promote their exports to the EU – as stipulated in the Export Strategy of Ukraine. For this purpose, Ukraine may explore possibilities for modifying the structure of certain TRQs (such as wheat, flour and pellets; maize, flour and pellets; barley, flour and pellets) to separate primary and processed products and to ensure more duty-free volumes for processed products.
References
- European Commission, 21.04.2020. DG Agriculture and Rural Development. “AGRI TRQs – Allocation Coefficients and Decisions”.
- European Commission, 12.02.2020. Remarks by Commissioner Várhelyi at a press conference with Prime Minister of Ukraine, Oleksiy Honcharuk.
- European Commission, DG Taxation and Customs Union, 21.04.2020. Tariff quota consultation.
- European Commission, 21.04.2020. “Trade Helpdesk Statistics.”
- OECD, 2018. “Fostering greater SME participation in a globally integrated economy”.
- Official Journal of the European Union, 2014. “EU-Ukraine Association Agreement”.
Disclaimer: Opinions expressed in policy briefs and other publications are those of the authors; they do not necessarily reflect those of the FREE Network and its research institutes.
A Decade of Russian Cross-Domain Coercion Towards Ukraine: Letting the Data Speak
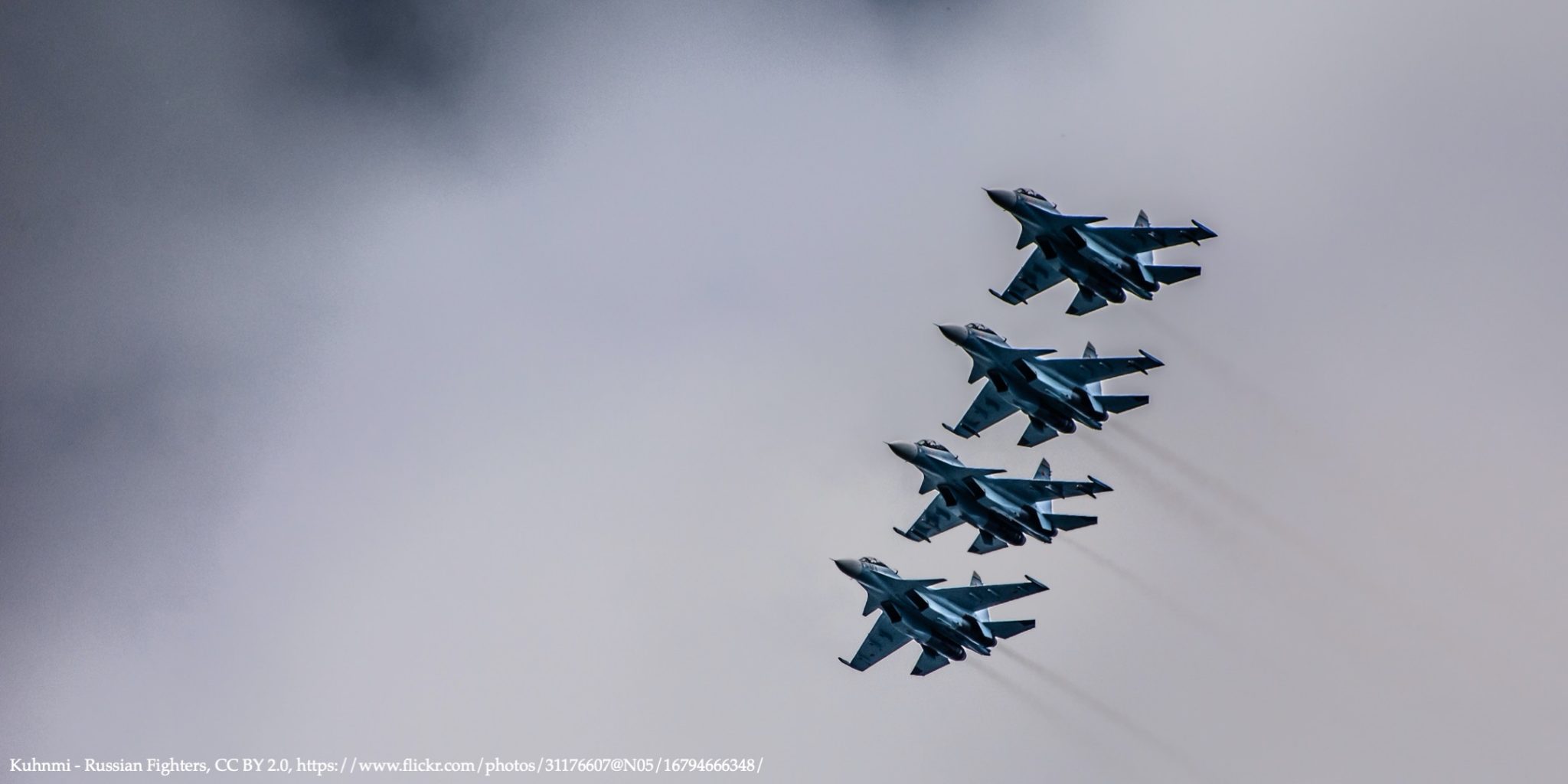
Russia’s coercion towards Ukraine has been a topic of major international events, meetings and conferences. It regularly makes the headlines of influential news outlets. But the question remains open – do we really understand it? We diligently collect and analyze data to make informed decisions in practically all domestic issues but is the same done for international relations? This research paper introduces a number of tools and methods that could be used to study Russia’s coercion towards Ukraine beyond its most visible manifestation, looking into latent trends and relations that could reveal more.
Introduction
For the past decade, Ukraine has been in the headlines of the major world news outlets more frequently than ever before. Ukrainian-Russian relations have been and still remain the topic of international summits and other events. The occupation of a part of Ukraine’s territory has been denounced and Russia’s coercion towards Ukraine is now generally accepted as a fact. But what do we really know about the underlying empirics and dynamics and how can this multi-domain assertiveness be measured and tracked? This paper presents a number of data-driven approaches that allow looking beyond the headline stories to identify and track various dimensions of Russia’s coercion towards Ukraine and the dynamics of its development.
Academic Interest
Mapping the landscape of scholarly literature reveals a number of interesting results. First, the body of works studying Russia’s coercion towards Ukraine remains relatively modest. It quintupled in 2014 but afterwards the interest started tapering off. A search for papers on this topic in Scopus and Web of Science with a very precise query (to increase the accuracy of search) and publication time of 2009-2019 returned 155 papers most of which were published in or after 2014.
Figure 1. Scholarly publications on Russian-Ukrainian relations.
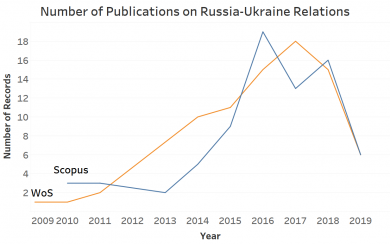
Source: WoS and Scopus, 2009-2019
A closer look at the content of these works with the use of a bibliometric software called CiteSpace shows that the majority of papers focus on Putin, once again emphasizing the significant role of his personality in Russia’s coercion towards Ukraine. The second largest cluster has the “great power identity” as its main theme and presumably looks beyond actions of Russia to identify its ideological grounds. Another group of publications is devoted to sanctions, pointing to their important role in studying Russian-Ukrainian relations.
Figure 2. The landscape of topics in scholarly publications on Russian-Ukrainian Relations.
Expressions of Coercion
The “practical” side of Russia’s coercion towards Ukraine is also frequently associated with the personality of Vladimir Putin and his attitudes towards Ukraine. To analyze this perception further, we created a corpus of speeches of Russian presidents published on the Kremlin website, filtered them to keep only those that mention Ukraine, divided them into pre-2014 and 2014 and after, and then analyzed them using an LDA topic modeling algorithm. This algorithm is based on the assumption that documents on similar topics use similar words. So, the latent topics that a certain document covers can be identified on the basis of probability distributions over words. Each document covers a number of topics that are derived by analyzing the words that are used in it. In simple terms, the model assigns each word from the document a probabilistic score of the most probable topic that this word could belong to and then groups the documents accordingly.
Figure 3. Speeches of Russian presidents before 2014, LDA topic modeling.
Figure 4. Speeches of Russian presidents in 2014 and after, LDA topic modeling.
Quite surprisingly, we discovered that the overall rhetoric of speeches is very similar for the two periods. Although some speeches do differ and the later corpus includes new vocabulary to reflect some changes (i.e “Crimea”, “war”) the most common words remain practically the same. Thus, regardless of the apparent shift in relations between the two countries, Russian leadership still relies on the same notions of collaboration, interaction, joint activities, etc. The narrative of “brotherhood” between the nations persists despite and beyond the obvious narrative of conflict.
To include a broader circle of Russia’s leadership we also looked at the surveys of the Russian elite conducted regularly by a group of researchers led by William Zimmerman and supported by various funders over the years (in 2016 – the National Science Foundation and the Arthur Levitt Public Affairs Center at Hamilton College). Seven waves of the survey already took place; the most recent one in 2016. The respondents were the representatives of several elite groups (government, including executive and legislative branches, security institutions, such as federal security service, army, militia, private business and state-owned enterprises, media, science and education; for practical reasons from Moscow only).
The survey revealed a number of interesting observations. For instance, while the prevailing Russian opinion on Russia’s occupation of Crimea had been that it was not a violation of international law, a closer look at the demographic characteristics of respondents shows that they were not as coherent as it might seem from the outside. While the “green” answers from respondents with backgrounds such as media or private business may have been anticipated, the number of members of the legislative and especially executive branch and the military that had at least some doubt on the legality was surprisingly quite sizable, and they even demonstrated some support of the “violation of law” interpretation.
Figure 5. Elite and public opinion on Russia’s annexation of Crimea.
Comparing these elite opinions to the public opinion poll by Levada Center conducted in the same year shows that even the general public is slightly more likely to choose the most extreme “full legality” option than the respondents from the executive branch.
Beyond the elite or general opinion polls, we tried to develop a metric that might allow us to track Russian sensitivities towards Ukraine. For that, we examined two different ways of expressing “in Ukraine” in Russian language: ‘на Украине’ (the ‘official’ Russian expression) vs. ‘в Украине’ (the version preferred by Ukrainians). [In English, this can be compared so saying ‘Ukraine’ vs ‘the Ukraine’.]
Our first visual plots how many search queries were done on Google Search with both versions over the last decade.
Figure 6. Search queries for “в Украине” (green) versus “на Украине” (red), Google Trends, 2009-2019.
We can clearly observe that during less turbulent times the more politically sensitive version is much more common. This however drastically changes during the peaks of Russia’s coercion towards Ukraine when the number of searches with the less politically correct term increases significantly.
A different trend can be observed if we look at official media publications stored in the Factiva database. We estimated the ratio of search volumes for each term and observed that until the beginning of 2013, about a third of articles and news reports used “in Ukraine”. This changed around January 2013 when the ratio starts to decrease for “in Ukraine” searches and plummets to a mere 10% of outlets still preferring this term.
Figure 7. The ratio of “в Украине” to “на Украине” occurrences in large Russia media (2009 – 2019), Factiva.
Tracking Coercion Itself
What is the track record of Russia’s actual coercion over this decade? For this, we turn to a few recent datasets that try to systematically capture verbal and material actions (words and deeds): the automated event datasets. The largest one of those, called GDELT (Global Database of Events, Language, and Tone), covers the period from 1979 to the present, and contains over three quarters of a billion events. It is updated every fifteen minutes to include all “events” reported in the world’s various news outlets. To exclude multiple mentions of the same event by one newswire, the events are “internally” deduplicated. The events are not compared across newswires.
An event consists of a “triple” coded automatically to represent the actor (who?), the action (what?) and the target (to whom?) as well as a number of other parameters such as type (verbal or material; conflict or cooperation; diplomatic, informational, security, military, economic), degree of conflict vs cooperation etc. Other similar datasets include ICEWS (Integrated Crisis Early Warning System) and TERRIER (Temporally Extended, Regular, Reproducible International Events Records). For this analysis, we filtered out only those events in which Russia was the source actor and Ukraine was the target country. We present two metrics: (1) the percentage of all world events that this subset of events represents and (2) the monthly averages of the Goldstein score, which captures the degree of cooperation or conflict of an event and can take a value from -10 (most conflict) to +10 (most cooperation). Also, to add a broader temporal perspective, we looked beyond the last decade. It can be clearly seen that the number of events before 2013 was significantly lower, especially in “material” domains. Some verbal assertions from Russia towards Ukraine happened during the Orange Revolution and so-called “gas wars”.
The situation changes radically starting from 2013. The proportion of events increases with some especially evident peaks (i.e. during the occupation of Crimea). The verbal events remain quite neutral while the actions towards Ukraine move from some fluctuations to steadily conflictual.
Figure 8. Russia’s negative assertiveness towards Ukraine, 2000-2019.
Measuring Influence
We have seen that the past decade was exceptional in the scale of Russian assertiveness towards Ukraine. But what do we know about Russia’s influence on Ukraine and Ukraine’s dependence on Russia? Influence measures the capacity of one actor to change the behavior of the other actor in a desired direction. In an international context this often concerns the relations between countries. Influence can be achieved by various means, one of which is to increase the dependence of the target country upon the coercive one. This strategy is frequently employed by Russia willing to regain and/or increase control over the former post-Soviet countries. The Formal Bilateral Influence Capacity (FBIC) Index developed by Frederick S. Pardee (Center for International Future) looks at several diplomatic (i.e. intergovernmental membership), economic (trade, aid) and security (military alliances, arms import) indicators allowing to identify the level of dependence of one country upon another. This is especially interesting from a comparative perspective. Figure 9 shows that countries such as Armenia and Belarus remain highly dependent on Russia. For half of the decade, Ukraine was number three on this list. Today the situation has changed. Ukraine’s dependence on Russia has gradually decreased and has become even smaller than Moldova’s, moving closer to the steadily low level of dependence of Georgia. This may signify a positive trend and a break of a decade-long relationship of dependence.
Figure 9. Dependence of post-Soviet countries on Russia, FBIC.
Conclusion
Consequently, Russia and Ukraine have become much more visible in the international academic and policy research efforts. This can be measured through a number of instruments, including a comprehensive mapping of the academic landscape itself with regard to salience and topics that are being studied, analysis of the word choice (that could be represented by the use of the terms to describe events in Ukraine by the government media and Google search users (“на Украине” versus “в Украине”); speeches of Russian presidents that use the same rhetoric of collaboration when talking about Ukraine despite the obvious change in relationships) and material coercion (significant increase in number of assertive conflictual Russia’s actions towards Ukraine). Some findings do give hope for change: the opinions of the Russian elite on recent Russian actions towards Ukraine while remaining generally unfavourable are not as cohesive as it might appear and Ukraine’s dependence on Russia has decreased significantly.
Disclaimer
This research is a part of a larger research effort titled RuBase funded by the Carnegie Foundation of New York and implemented jointly by The Hague Centre for Strategic Studies and Georgia Tech with the help of the Kyiv School of Economics StratBase team in Ukraine. The ‘Ru’ part of the title stands for Russia; and ‘base’ has a double meaning – both the knowledge base built during the project, and the (aspirationally) foundational nature of this effort. The project intends to look beyond the often-shallow traditional understanding of coercion and apply innovative tools and instruments to study coercion in its multifaceted form. This is only a small selection of the tools that have been successfully tested in the course of this (ongoing) research project and applied to the study of Russia’s coercion in different domains. The prospects of any progress in resolving the Russian-Ukrainian conflict are currently slim, thus further work that would allow identifying patterns and trends that the human eye may oversee to understand Russia better and develop an informed foreign policy strategy both for Ukraine and the West is crucially important.
References
- Boschee, Elizabeth et al. (2019). “ICEWS Automated Daily Event Data.” (November 12, 2019).
- Clarivate Analytics (2019). “Web of Science Core Collection.” Web of Science Group. (January 20, 2020).
- Dow Jones (2019). “Factiva – Global News Monitoring & Search Engine.” Dow Jones. (December 2, 2019).
- Elsevier (2019). “Scopus.” (December 3, 2019).
- Google (2018). “Google Trends – The Homepage Explained – Trends Help.” (January 20, 2020).
- Holynska, Khrystyna, Yevhen Sapolovych, Mikhail Akimov, and Stephan De Spiegeleire (2019). “Events Datasets and Strategic Monitoring: Method Piece” (Forthcoming). The Hague Centre For Strategic Studies.
- Levada-Center (2019). “Levada Center.” (December 3, 2019).
- Moyer, Jonathan D., Tim Sweijs, Mathew J. Burrows, and Hugo Van Manen (2018) “Power and Influence in a Globalized World.” Atlantic Council. (November 26, 2019).
- OU Event Data (2018). “Terrier (Temporally Extended, Regular, Reproducible International Event Records)”. (January 29, 2020).
- The GDELT Project (2015). “GDELT 2.0: Our Global World in Realtime.” GDELT Blog. (October 11, 2018).
- Zimmerman, William, Sharon Werning Rivera, and Kirill Kalinin. (2019). “Survey of Russian Elites, Moscow, Russia, 1993-2016”. Version 6.” (November 26, 2019).
- Президент России (2019). “Президент России.” Президент России. (November 26, 2019).
Russia’s Real Cost of Crimean Uncertainty
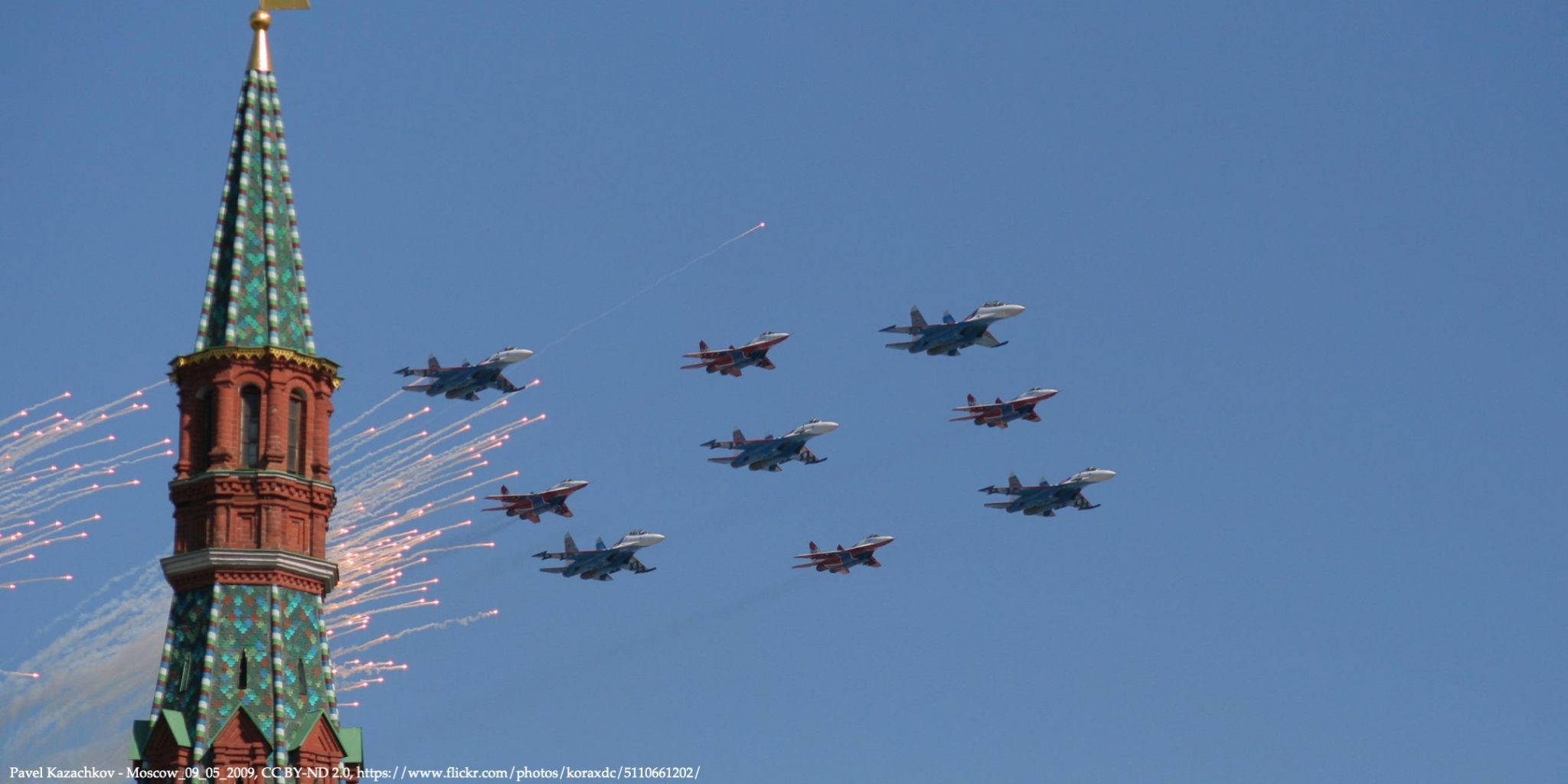
The annexation of Crimea has real costs to the Russian economy beyond what is measured by some items in the armed forces’ budget; social spending in the occupied territories; or the cost of building a rather extreme bridge to solve logistics issues. Russia’s real cost of the annexation of Crimea is also associated with the permanent loss of income that the entire Russian population is experiencing due to increased uncertainty, reduced capital flows and investment, and thus a growth rate that is significantly lower than it would have been otherwise. Since the years of lost growth are extremely hard to make up for in later years, there will be a permanent loss of income in Russia that is a significant part of the real cost of annexing Crimea and continuing the fighting in Eastern Ukraine. It is time to stop not only the human bleeding associated with Ukraine, but also the economic.
Estimating the real cost of Russia’s annexation of Crimea and the continued involvement in Eastern Ukraine is complicated since there are many other things going on in the Russian economy at the same time. In particular, oil prices fell from over $100/barrel in late 2013 to $30/barrel in 2016 (Figure 1). Becker (2016) has shown that 60-80 percent of the variation in GDP growth can be explained by changes in oil prices, so this makes it hard to just look at actual data on growth to assess the impact of Crimea and subsequent sanctions and counter sanctions.
Figure 1. Russian GDP and oil price
Source: Becker (2019)
The approach here is instead to focus on one channel that is likely to be important for growth in these circumstances, which is uncertainty and its impact on capital flows and investment.
From uncertainty to growth
The analysis presented here is based on several steps that link uncertainty to GDP growth. All the details of the steps in this analysis are explained at some length in Becker (2019). Although this brief will focus on the main assumptions and estimates that are needed to arrive at the real cost of Crimea, a short description of the steps is as follows.
First of all, in line with basic models of capital flows, investors that can move their money across different markets (here countries) will look at relative returns and volatility between different markets. When relative uncertainty goes up in one market, capital will leave that market.
The next step is that international capital flows affect investment in the domestic market. If capital leaves a country, less money will be available for fixed capital investments.
The final step is that domestic investments is important for growth. Mechanically, in a static, national accounts setting, if investments go down, so does GDP. More long term and dynamically, investments have a supply side effect on growth, and if investments are low, this will affect potential as well as actual growth negatively.
These steps are rather straightforward and saying that uncertainty created by the annexation of Crimea leads to lower growth is trivial. What is not trivial is to provide an actual number on how much growth may have been affected. This requires estimates of a number of coefficients that is the empirical counterparts to the theoretical steps outlined here.
Estimates to link uncertainty to growth
In short, we need three coefficients that link: domestic investments to growth; capital flows to domestic investments; and uncertainty to capital flows.
There are many studies that look at the determinants of growth, so there are plenty of estimates on the first of these coefficients. Here we will use the estimate of Levine and Renelt (1992), that focus on finding robust determinants of growth from a large set of potential explanatory variables. In their preferred specification, growth is explained well by four variables, initial income, population growth, secondary education and the investments to GDP ratio. The coefficient on the latter is 17.5, which means that when the investment to GDP ratio increases by 10 percentage points, GDP grows an extra 1.75 percentage points per year. Becker and Olofsgård (2018) have shown that this model explains the growth experience of 25 transition countries including Russia since 2000 very well, which makes this estimate relevant for the current calculation.
The next coefficient links capital flows to domestic investments. This is also a subject that has been studied in many empirical papers. Recent estimates for transition countries and Russia in Mileva (2008) and Becker (2019) find an effect of FDI on domestic investments that is larger than one, i.e., there are positive spillovers from FDI inflows to domestic investments. Here we will use the estimate from Becker (2019) that finds that 10 extra dollars of FDI inflows are associated with an increase of domestic investments of 15 dollars.
Finally, we need an estimate linking uncertainty with capital flows. There are many studies looking at risk, return and investment in general, and also several studies focusing on international capital flows and uncertainty. Julio and Yook (2016) look at how political uncertainty around elections affect FDI of US firms and find that FDI to countries with high institutional quality is less affected by electoral uncertainty than others. Becker (2019) estimates how volatility in the Russian stock market index RTS relative to the volatility in the US market’s S&P 500 is associated with net private capital outflows. The estimate suggests that when volatility in the RTS goes up by one standard deviation, this is associated with net private capital outflows of $30 billion.
These estimates now only need one more thing to allow us to estimate how much Crimean uncertainty has impacted growth and this is a measure of the volatility that was created by the annexation of Crimea.
Measuring Crimean uncertainty
In Becker (2019), the measure of volatility that is used in the regression with net capital outflows is the 60-day volatility of the RTS index. Since we now want to isolate the uncertainty created by Crimea related events, we need to take out the volatility that can be explained by other factors in order to arrive at a volatility measure that captures Crimean induced uncertainty. In Becker (2019) this is done by running a regression of RTS volatility on the volatility of international oil prices and the US stock market as represented by the S&P 500. The residual that remains after this regression is the excess volatility of the RTS that cannot be explained by these two external factors. The excess volatility of the RTS index is shown in figure 2.
It is clear that the major peaks in excess volatility are linked to Crimea related events, and in particular to the sanctions introduced at various points in time. From March 2014 to March 2015, there is an average excess volatility of 0.73 standard deviations with a peak of almost 4 when the EU and the USA ban trade with Crimea. This excess volatility is our measure of the uncertainty created by the annexation of Crimea.
Figure 2. RTS excess volatility
Source: Becker (2019)
From Crimean uncertainty to growth
The final step is simply to use our measure of Crimean induced uncertainty together with the estimates that link uncertainty in general to growth.
The estimated excess volatility associated with Crimea is conservatively estimated at 0.7 standard deviations. Using this with the estimate that increasing volatility by one standard deviation is associated with $30 billion in capital outflows, we get that the Crimean uncertainty would lead to $21 billions of capital outflows in one quarter or $84 billions in one year. If this is in the form of reduced FDI flows, we have estimated that this means that domestic investments would fall by a factor of 1.5 or $126 billions.
In this period, Russia had a GDP of $1849bn and fixed capital investments of $392bn. This means that $126 billions in reduced investments correspond to a reduction in the investments to GDP ratio of 7 percentage points (or that the investments to GDP ratio goes from around 21 percent to 14 percent).
Finally, using the estimate of 17.5 from Levine and Renelt, this implies that GDP growth would have been 1.2 percentage points higher without the estimated decline in investments to GDP.
In other words, the Crimean induced uncertainty is estimated to have led to a significant loss of growth that has to be added to all the other costs of the annexation of Crimea and continued fighting in Eastern Ukraine. Note that recent growth in Russia has been just barely above 1 percent per year, so this means that growth has been cut in half by this self-generated uncertainty.
Of course, the 1.2 percentage point estimate of lost growth is based on many model assumptions, but it provides a more sensible estimate of the cost of Crimea than we can get by looking at actual data that is a mix of many other factors that have impacted capital flows, investments and growth over this period.
Policy conclusions
The annexation of Crimea and continued fighting in Eastern Ukraine carry great costs in terms of human suffering. In addition, they also carry real costs to the Russian economy. Not least to people in Russia that see that their incomes are not growing in line with other countries in the world while the value of their rubles has been cut in half. Some of this is due to falling oil prices and other global factors that require reforms that will reorient the economy from natural resource extraction to a more diversified base of income generation. This process will take time even in the best of worlds.
However, one “reform” that can be implemented over night is to stop the fighting in Eastern Ukraine and work with Ukraine and other parties to get out of the current situation of sanctions and counter-sanctions. This would provide a much-needed boost to foreign and domestic investments required to generate high, sustainable growth to the benefit of many Russians as well as neighboring countries looking for a strong economy to do trade and business with.
References
- Becker, T, (2019), “Russia’s macroeconomy—a closer look at growth, investment, and uncertainty”, forthcoming SITE Working paper.
- Becker, T. and A. Olofsgård, (2018), “From abnormal to normal—Two tales of growth from 25 years of transition”, Economics of Transition, vol. 26, issue 4.
- Becker, T. (2016), “Russia and Oil – Out of Control”, FREE policy brief, October.
- Julio, B. and Yook, Y. (2016), ‘Policy uncertainty, irreversibility, and cross-border flows of capital’, Journal of International Economics, Vol. 103, pp. 13-26.
- Levine, R. and Renelt, D. (1992). ‘A Sensitivity Analysis of Cross-Country Growth Regressions’, American Economic Review, 82(4), pp. 942–963.
- Mileva, E. (2008), ‘The Impact of Capital Flows on Domestic Investment in Transition Economies, ECB Working Paper No. 871, February.
Disclaimer: Opinions expressed in policy briefs and other publications are those of the authors; they do not necessarily reflect those of the FREE Network and its research institutes.
Labor Market Adaptation of Internally Displaced People: The Ukrainian Experience
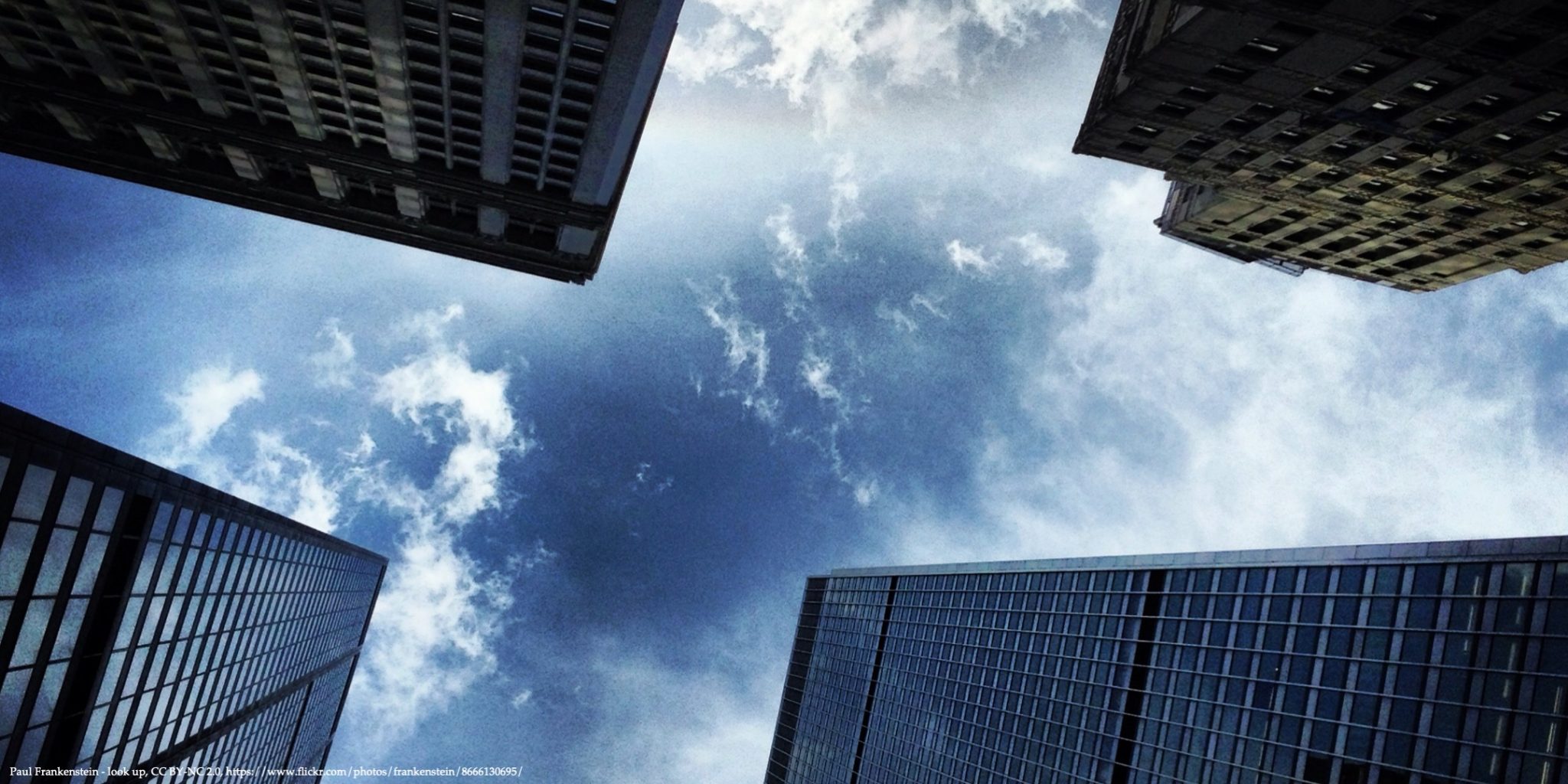
This brief is based on research that investigates the probability of employment among displaced and non-displaced households in a region bordering territory with an ongoing military conflict in Eastern Ukraine. According to the results, internally displaced persons (IDP) are more educated, younger and more active in their job search than locals. Nevertheless, displaced individuals, particularly males, have experienced heavy discrimination. After controlling for personal characteristics, the structure of the household, location, non-labour incomes and endogeneity of displacement, IDP males are 17% less likely to be formally employed two years after resettlement than locals.
Internally displaced persons in Ukraine
In 2014, 23 years after independence, Ukraine suddenly found itself among the top-ten of countries with the largest internally displaced population. During the period 2014–2016, 1.8 million persons registered as internally displaced. Potentially, about 1 million more reallocated to Russia and about 100,000 to other countries nearby, where they sought refugee or labour migrant status (Smal, 2016).
The Ministry of Social Policy of Ukraine (MSPU) has regularly published very general reports on displaced persons. According to these reports, at the end of February 2016, the internally displaced persons in Ukraine included 22,000 individuals from Crimea and over 1.7 million citizens from Eastern Ukraine. These are mostly individuals who registered as IDPs to qualify for financial assistance from the state and some non-monetary benefits. Among them, 60% are retired people, 23.1% are individuals of working age, 12.8% are children and 4.1% are people with disabilities (Smal and Poznyak, 2017). In fact, the MSPU registers not only displaced persons but also those who de facto live in the occupied territories and occasionally travel to territories controlled by the Ukrainian authorities to receive their pension or social benefits (so called ‘pension tourism’). On the other hand, some IDPs did not register either to avoid bureaucracy or because they were unable to prove their status due to lack of documents. Recent publications that are based on surveys portray a more balanced distribution: 15% are retired people, 58% are individuals of working age, 27% are children and 13% are people with disabilities (IOM and the Ukrainian Centre for Social Reforms, 2018).
Only limited information is available about IDPs’ labour market activity. According to the State Employment Service (SES), between March 2014 and January 2016, only 64,300 IDPs or 3.75% referred to the SES for assistance (Smal and Poznyak, 2017). On the one hand, this figure reflects the relatively low reliance of displaced Ukrainians on the SES services in their job search. On the other hand, the geographical variation in the share of SES applicants suggests that Ukraine’s IDPs who moved further from the war zone and their homes were more active in trying to find a job.
Data
Our primary data were collected in June–August 2016 by REACH and provided by the Ukraine Food Security Cluster (UFSC) as a part of the needs assessment in Luhansk and Donetsk oblasts of Ukraine – two regions that were directly affected by the conflict. These two regions have hosted roughly 53% of all IDPs in Ukraine (Smal and Poznyak, 2017). We argue that households that did not move far from the place of conflict are most likely to be driven by conflict only, while long-distance movers may combine economic and forced displacement motives.
The data set offers information on 2500 households interviewed in 233 locations and is statistically representative of the average household in each oblast. It includes respondents currently living in their pre-conflict settlements (non-displaced, NDs) and respondents who report a different place of residence before the conflict (IDPs). The IDP group comprises individuals with registered and unregistered status and from both sides of the current contact line. The non-IDP group includes only households living on the territory controlled by the Ukrainian Government that did not move after the conflict had started.
Our sample covers 1,135 displaced households that came from 131 settlements. Most of the reallocations took place in early summer 2014 with the military escalation of the conflict in Eastern Ukraine. Thus, the average duration of displacement up to the moment of the interview was 637 days (or 21 months). This is a sufficiently long period for adaptation and job search. However, there is enough variation in this indicator – some families left as early as March–April 2014, while others were displaced in June 2016, just a few days before the interviews started.
Results
Simple comparison shows that heads of displaced households are on average almost four years younger than those of non-displaced households (Table 1). In terms of education, displaced households are found to be more educated than non-displaced households, as there are significantly more IDP household heads with tertiary education and significantly fewer individuals with only primary, secondary or vocational degrees. In particular, 37% of IDP household heads hold a university degree compared with 22% of household heads among the local population. This seems to suggest positive displacement selection. IDPs are slightly more likely to be headed by females and unmarried persons, although these differences are statistically insignificant. Displaced households include more children aged under five (0.35 vs. 0.22 children per non-displaced household) and 6 to 17 years (0.42 vs. 0.34, respectively) and fewer members aged over 60 years (0.58 vs 0.66, respectively). There is no difference in the number of working-age adults or disabled individuals per household among IDPs and non-IDPs. The average household size is statistically similar for the groups (2.74 vs. 2.65 persons per IDP and non-IDP household, respectively).
Table 1. Selected descriptive statistics
Internally displaced households | Non- displaced households | |
Household head employed | 0.43*** | 0.48*** |
Household head characteristics | ||
Age (years) | 48.10*** | 52.85*** |
Male | 0.49 | 0.52 |
Education | ||
vocational | 0.42*** | 0.49*** |
university | 0.37*** | 0.22*** |
Household characteristics | ||
Size (persons) | 2.74 | 2.65 |
Number of children 0-5 | 0.35*** | 0.21*** |
Number of children 6-17 | 0.42*** | 0.34*** |
Number of members 60+ | 0.58** | 0.66** |
IDP payments | 0.50*** | 0*** |
Humanitarian assistance | 0.78*** | 0.28*** |
There are further differences in the types of economic activity and occupations among IDPs and non-IDPs. Prior to the conflict, displaced respondents were more likely (than non-displaced persons) to be employed as managers or professionals and less likely to hold positions as factory or skilled agricultural workers. This result also speaks in favor of a positive displacement selection story.
As expected, the conflict has had a negative effect on human capital in the government controlled areas of Donetsk and Luhansk regions. We observe some deskilling at the time of the interviews, which is especially pronounced for IDPs. In particular, the share of managers among the IDPs had reduced from 12% to 5% and that of technicians from 15% to 12%, while the proportion of service and sales employees had increased from 10% to 13%, that of factory workers from 11% to 15% and that of skilled agricultural workers from 2% to 6%.
Considering the economic activity in the current location, we can note that on average the heads of displaced households are 5% less likely to be employed than those of non-displaced households (43% vs. 48%, respectively). In both groups, a large share of respondents report difficulties in their job search, but IDPs are 13% more likely to experience this problem. They report changing their pre-conflict occupation three times more often than non-IDPs (37% vs. 11%).
Government and non-government assistance may also drive the differences in employment. Economic theory states that individuals are less likely to work if they have some backup in the form of non-labour earnings. Financial support and humanitarian assistance are widely used to smooth a displacement shock. At the same time, improperly designed assistance schemes may reduce the stimulus to search for a job.
IDPs are 9% less likely to include earnings in their household’s top three main sources of income than the non-displaced population (46% vs. 55%, respectively), meaning that they rely more on various social payments and pensions. In addition, displaced households may be slightly more reluctant to search for a job due to displacement assistance from the government (received by 50% of IDPs compared with 0% for non-IDP households), although the amounts are quite modest. According to the existing legislation, IDPs can receive regular monthly state payments and one-time state payments. Regular monthly payments can be received by any IDP and cannot exceed UAH 3,000 (~$111) for an ordinary household, UAH 3,400 for a household with disabled people and UAH 5,000 (~$185) for a household with more than 2 children. Eligibility and the size of the one-time payment are determined by the local government. In the data set, 95% of IDPs receive less than UAH 3,000 while the 2016 average monthly wage was UAH 6,000 in Donetsk and UAH 4,600 in Luhansk regions.
In addition, IDPs are three times more likely to receive humanitarian assistance (78% vs. 28% among displaced and non-displaced persons, respectively). This support includes mostly food and winterisation items but also cash (26% among displaced vs. 12% among non-displaced assistance receivers). On the other hand, to cover reallocation and adaptation costs, some IDPs use their financial reserves, and as a result they are by 10 p.p. more likely to report no or already depleted savings. This may increase their stimulus to engage in a more active job search.
After taking into account the observed and unobserved differences between the groups as well as controlling for the location fixed effect, we find that the difference in the probability of employment between displaced and non-displaced persons increases from a casually observed slit of 5% to a chasm of 17.3%. This result suggests that IDPs are [negatively] discriminated despite being younger, more educated, skilled and more ‘able’ in the labour market. Specifically, 7 out of 17 p.p. (41% of the gap) are due to the variation in observed household head characteristics and family composition, while unobserved displacement-related features (such as attitude towards change, activism, mental and physical ability to reallocate) account for 5 p.p. (29%) of the gap. Controlling for particularities of a current location does not substantially affect the estimated differences.
Figure 1. Main results
We re-estimate these regressions using an employment indicator that includes both formal and informal employment (as defined by the respondents), accounting for occasional and irregular employment, including subsistence agricultural work. Since informal work is more common among IDPs, this definition of employment leads to a reduction in the average casually observed gap from 5% to 3%. However, after controlling for all the factors, we obtain the same result – a 17.8% difference between displaced and non-displaced households.
Conclusion
Policy makers and international donors should not be misled by the seemingly comparable probability of employment among IDPs and non-IDPs based on simple statistics. The average 0–5% difference in unconditional employment rates conceals the actual 17% gap in the likelihood of having a job. The contribution of unobserved displacement-related factors in hiding the true gap is large, especially for males seeking formal employment. Without adjusting for it, we would underestimate the real difference in employment probability by one-third to one-half.
Our study produces firm evidence that displaced individuals in Ukraine, particularly males, have been discriminated against in terms of employment. Our results further suggest that male heads of displaced households experience more discrimination in the formal labour market, while the situation is the opposite for females, who are more likely to face unequal treatment in the informal sector. Policy makers and volunteers should take this difference into account in the adaptation of male- and female-headed households.
Humanitarian assistance to displaced individuals was found to have no negative effect on their employment, which suggests that it is provided in an effective manner. Thus, this tool can be used to mitigate the discrimination.
References
- IOM and the Ukrainian Centre for Social Reforms. (2018). ’National Monitoring System Report on the Situation of Internally Displaced Persons.’
- Smal, V. and O. Poznyak. (2017) ‘Internally displaced persons: social and economic integration in hosting communities’, PLEDDG Project.
- Smal, V. 2016. ’Внутрішньо Переміщені Особи: Соціальна та економічна інтеграція в приймаючих громадах.’
- Vakhitova, H. and P. Iavorskyi, “Employment of Displaced and Non-displaced Households in Luhansk and Donetsk Oblasts”, Europe-Asia studies, (forthcoming).
Disclaimer: Opinions expressed in policy briefs and other publications are those of the authors; they do not necessarily reflect those of the FREE Network and its research institutes.
Conflict, Minorities and Well-Being
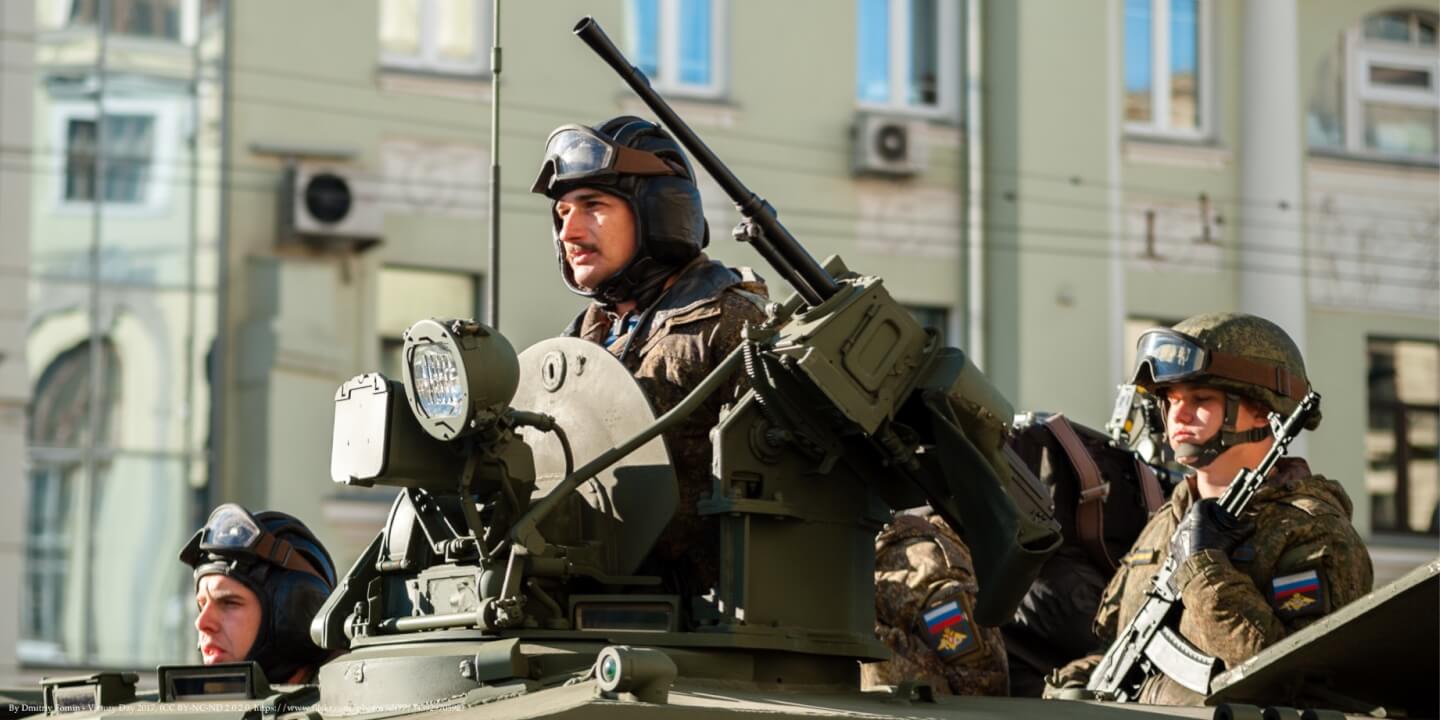
We assess the effect of the Russo-Georgian conflict of 2008 and the Ukrainian-Russian conflict of 2014 on the well-being of minorities in Russia. Using the Russian Longitudinal Monitoring Survey (RLMS), we find that the well-being of Georgians in Russia suffered negatively from the 2008 Russo-Georgian conflict. In comparison, we find no general effect of the Ukrainian-Russian conflict of 2014 on the Ukrainian nationals’ happiness. However, the life satisfaction of Ukrainians who reside in the southern regions of Russia in close proximity to Ukraine is negatively affected. We also show that the negative effect of conflict is short-lived with no long-term legacy. Additionally, we analyze the spillover effect of conflict on other minorities in Russia. We find that while the well-being of non-Slavic and migrant minorities who have recently moved to Russia is negatively affected, there is no effect on local minorities who have been living in Russia for at least ten years.
Militarized conflict affects a myriad of socioeconomic outcomes, such as the level of GDP (Bove et al. 2016), household welfare (Justino 2011), generalized trust and trust in central institutions (Grosjean 2014), social capital (Guriev and Melnikov 2016), and election turnout (Coupe and Obrizan 2016). Importantly, conflict has also been found to directly affect individual well-being (Frey 2012, Welsch 2008).
However, previous research studying individual well-being in transition countries largely abstracts from heightened political instability and conflict proneness, while this has been particularly pertinent in transition countries. Examples of transition countries facing various types of conflicts are abound, such as Yugoslavia, Ukraine, Tajikistan, Russia, Armenia, Azerbaijan, Moldova, and so on. Therefore, it is imperative to explore how conflict shapes well-being in transition countries.
In a new paper (Gokmen and Yakovlev, forthcoming), we add to our understanding of well-being in transition in relation to conflict. We focus on the effect of Russo-Georgian conflict of 2008 and the Ukrainian-Russian conflict of 2014 on the well-being of minorities in Russia. The results suggest that the well-being of Georgians in Russia suffered negatively from the 2008 Russo-Georgian conflict. However, we find no general effect of the Ukrainian-Russian conflict of 2014 on the Ukrainian nationals’ happiness, while the life satisfaction of Ukrainians who reside in the southern regions of Russia in close proximity to Ukraine is negatively affected. Additionally, we analyze the spillover effect of conflict on other minorities in Russia. We find that while the well-being of non-slavic and migrant minorities who have recently moved to Russia is negatively affected, there is no effect on local minorities who have been living in Russia for at least ten years.
Data and Results
We employ the Russian Longitudinal Monitoring Survey (RLMS) which contains data on small neighborhoods where respondents live. Starting from 1992, the RLMS provides nationally-representative annual surveys that cover more than 4000 households with 10000 to 22000 individual respondents. The RLMS surveys comprise a broad set of questions, including a variety of individual demographic characteristics, health status, and well-being. Our study utilizes rounds 9 through 24 of the RLMS from 2000 to 2015.
In this survey, we identify minorities with the question of “What nationality do you consider yourself?” Accordingly, anybody who answers this question with a non-Russian nationality is assigned to that minority group.
We employ three measures of well-being. Our main outcome variable is “life satisfaction.” The life satisfaction question is as follows: “To what extent are you satisfied with your life in general at the present time?”, and evaluated on a 1-5 scale from not at all satisfied to fully satisfied. Additionally, we use “job satisfaction” and “health evaluation” as outcomes of well-being.
Our results suggest that our primary indicator of well-being, life satisfaction, for Georgian nationals has gone down in the Russo-Georgian conflict year of 2008 compared to the Russian majority (see Figure 1). The magnitude of the drop in life satisfaction is about 39 percent of the mean life satisfaction. Our estimates for the other two well-being indicators, job satisfaction and health evaluation, also indicate a dip in the conflict year of 2008. Lastly, our estimates show that the negative impact of the conflict does not last long. Although there is a reduction in the well-being of Georgians both on impact in 2008 and in the immediate aftermath in 2009, the rest of the period until 2015 is no different from the pre-2008 period.
Figure 1. Life Satisfaction of Georgian Nationals in Russia
Source: Authors’ own construction based on RLMS data and diff-in-diff estimates.
Furthermore, when we investigate the effect of the Ukrainian-Russian conflict of 2014, we find no negative effect on the life satisfaction of Ukrainians. One explanation for why the happiness of Ukrainians in Russia does not seem to be negatively affected in 2014 is that the degree of integration of Ukrainians into the Russian society is much stronger than the degree of integration of Georgians. On the other hand, our heterogeneity analysis reveals that in the southern parts of Russia closer to the Ukrainian border, where there are more Ukrainians who have ties to Ukraine, Ukrainian nationals are differentially more negatively affected by the 2014 conflict. The differential reduction in the happiness of Ukrainians is about 19 percent of the mean life satisfaction.
Moreover, we also look into whether there is any spillover effects of the Russo-Georgian and the Ukrainian-Russian conflicts on the well-being of other minorities. We first carry out a simple exercise on non-Slavic minorities of Russia. We pick the sample of non-Slavic ex-USSR nationals that are similar to Georgians in their somatic characteristics, such as hair color and complexion. This group of people include the nationals of Azerbaijan, Kazakhstan, Uzbekistan, Kyrgyzstan, Turkmenistan and Tajikistan. We treat this group as “the countries with predominantly non-Slavic population” as their predominant populations are somatically different from the majority Russians, and thus, might either have been subject to discrimination or might have feared a minority backlash to themselves during the times of conflict. This conjecture finds some support below in Figure 2 in terms of violence against minorities. We observe in Figure 2 that hate crimes and murders based on nationality and race peak in 2008.
Our estimates also support the above hypothesis and propose that there is some negative effect of the 2008 conflict on non-slavic minorities’ happiness as well as their job satisfaction, whereas 2014 conflict has no effect.
Figure 2. Hate Murders in Russia over Time
Source: Sova Center
Next, we investigate the spillover effects of conflict on Migrant Minorities. Migrant minorities are minorities who have been living in their residents in Russia for less than 10 years. We conjecture that these minorities, as opposed to the minorities who have been in place for a long time, could be more susceptible to any internal or external conflict between Russia and some other minority group for fear that they themselves could also be affected. Whereas other types of longer-term resident minorities, which we call Local Minorities, are probably less vulnerable since they have had more time to establish their networks, job security, and most likely also have Russian citizenship. Our estimates back up the above conjecture and demonstrate that migrant minorities suffer negatively from the spillover effects of the 2008 conflict onto their well-being captured by any of the three measures, and not from the 2014 conflict, whereas there is no negative impact on local minorities.
Conclusion
In this paper, instead of focusing on the direct impact of conflict on happiness in war-torn areas, we contribute to the discussion on conflict and well-being by scrutinizing the well-being of people whose country of origin experiences conflict, but they themselves are not in the war zone. Additionally, we show that some other minority groups also suffer from such negative spillovers of conflict. Being aware of such negative indirect effects of conflict on well-being is essential for policy makers, politicians and researchers. Most policy analyses ignore such indirect costs of conflict, and this study highlights the bleak fact that the cost of conflict on well-being is probably larger than it has been previously estimated.
References
- Bove, V.; L. Elia; and R. P. Smith, 2016. “On the heterogeneous consequences of civil war,” Oxford Economic Papers.
- Coupe, T.; and M. Obrizan, 2016. “Violence and political outcomes in Ukraine: Evidence from Sloviansk and Kramatorsk”, Journal of Comparative Economics, 44, 201-212.
- Frey, B. S., 2012. “Well-being and war”, International Review of Economics, 59, 363-375.
- Gokmen, Gunes; and Evgeny Yakovlev, forthcoming. “War and Well-Being in Transition: Evidence from Two Natural Experiments”, Journal of Comparative Economics.
- Grosjean, P., 2014. “Conflict and social and political preferences: Evidence from World War II and civil conflict in 35 European countries” Comparative Economic Studies, 56, 424-451.
- Guriev, S.; and N. Melnikov, 2016. “War, inflation, and social capital,” American Economic Review: Papers & Proceedings, 106, 230-35.
- Justino, P., 2011. “The impact of armed civil conflict on household welfare and policy,” IDS Working Papers.
- Welsch, H., 2008. “The social costs of civil conflict: Evidence from surveys of happiness” Kyklos, 61, 320-340.
Disclaimer: Opinions expressed in policy briefs and other publications are those of the authors; they do not necessarily reflect those of the FREE Network and its research institutes.
What Does Ukraine’s Orange Revolution Tell Us About the Impact of Political Turnover on Economic Performance?
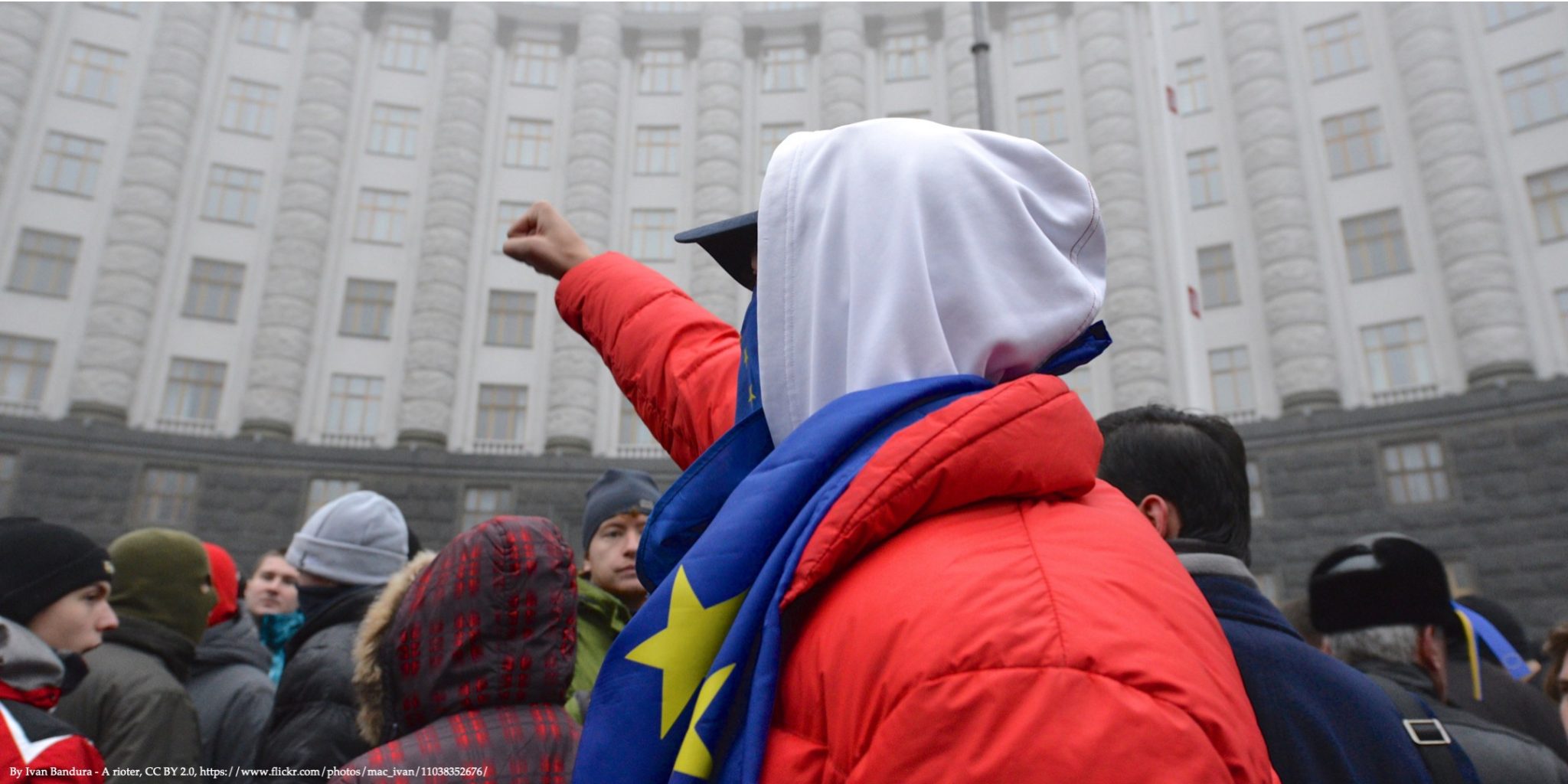
Political turnover is a normal, even desirable, feature of competitive politics, yet turnover in a context of weak institutions can create policy uncertainty, disrupt political connections, and threaten the security of property rights. What is the impact of political turnover on economic performance in such an environment? We examine the behavior of over 7,000 enterprises before and after Ukraine’s Orange Revolution—a moment of largely unanticipated political turnover in a country with profoundly weak institutions. We find that the productivity of firms in regions that supported Viktor Yushchenko increased after the Orange Revolution, relative to that of firms in regions that supported Viktor Yanukovych. Our results illustrate that the efficiency consequences of turnover can be large when institutions are weak.
Introduction
Politics in much of the world is a winner-take-all contest. When Viktor Yanukovych fled Kyiv in February 2014, for example, he was joined by a close group of associates overwhelmingly drawn from the country’s Russian-speaking East, including Yanukovych’s home region of Donetsk. The governors who ran Ukraine’s regions under Yanukovych fared no better. Oleksandr Turchynov, who served as acting president from February to June of that year, did what all Ukrainian presidents do: he fired the existing governors and replaced them with figures friendly to the new regime.
What is the impact of such political turnover on economic performance? In principle, replacement of political elites can have profound consequences for enterprise owners and managers, who rely on the support of patrons in government for government contracts, direct and indirect subsidies, the security of property rights, and permits to do business. In a system without effective checks and balances, economic policy can also swing widely as power passes from one group to another. Yet little is known about the impact of such changes on firm productivity, a major driver of economic welfare.
We examine the impact of political turnover on productivity and other aspects of firm performance in “The Productivity Consequences of Political Turnover: Firm-Level Evidence from Ukraine’s Orange Revolution” (Earle and Gehlbach, 2015). Our main finding is that the productivity of firms in regions that supported Yushchenko, the eventual winner of the 2004 presidential election, increased after the Orange Revolution, relative to that of firms in regions that supported Yanukovych, the chosen successor of incumbent President Leonid Kuchma. These results demonstrate that political turnover in a context of weak institutions can have major efficiency consequences as measured by differences in firm productivity.
Ukraine in 2004
Three factors make Ukraine in 2004 an appropriate setting for identifying the effect of political turnover on economic performance. First, Ukraine under Kuchma was a paradigmatic case of “patronal presidentialism,” in which the president “wields not only the powers formally invested in the office but also the ability to selectively direct vast sources of material wealth and power outside of formal institutional channels” (Hale 2005, p. 138). Who won the presidential contest had enormous implications for economic activity.
Second, economic and political power was regionally concentrated in Ukraine’s Russian-speaking East—Yanukovych himself was closely affiliated with oligarchs in Donetsk—while the political opposition represented by Yushchenko had its base in the ethnically Ukrainian and less industrialized West. Voting in Ukraine’s 2004 presidential election reflected this regional divide.
Third, few gave Yushchenko much chance of winning the presidency until the presidential campaign was well underway. In the end, it took not only a highly contested election, but also sustained street protests to wrest power from the existing elite.
Together, these considerations imply not only that political turnover in Ukraine could have an impact on firm performance, but also that any such effect could be observed by comparing the performance of enterprises in regions supportive of the two candidates before and after Yushchenko’s unexpected election victory.
The Orange Revolution and Firm Performance
To analyze the impact of political turnover, we use data on over 7,000 manufacturing enterprises that we track over many years, both before and after the Orange Revolution. We compare the evolution of productivity across firms in regions by vote in the 2004 election that was won by Yushchenko, while controlling for any shocks to particular industries in any year, for constant differences across firms in the level or trend of their productivity, and for regional differences in industrial structure. This design avoids many of the other influences on firm-level productivity that might have coincided with the Orange Revolution.
Our primary finding is that the productivity of firms in regions that supported Yushchenko in 2004 increased after Yushchenko took power, relative to the productivity of firms in regions that supported Yanukovych (and, implicitly, his patron Kuchma, whom Yushchenko succeeded as president). This effect is most pronounced among firms that had the most to gain or lose from presidential turnover: firms in sectors that rely on government contracts; private enterprises, given Ukraine’s weak property rights; and large enterprises. Other measures of economic performance suggest that these results are driven by favorable treatment of particular firms, either before or after the Orange Revolution, rather than by broad changes in economic policy.
Conclusion
Political turnover is often desirable. Nonetheless, our results suggest that the distributional consequences can be profound when institutions are weak, that is, when access to those in power is the primary guarantee of market access, contract enforcement, and property-rights protection. Oscillation of privilege from one region or sector to another is inefficient, as firms initiate or postpone restructuring based on who is in power. The optimal solution, of course, is not to restrict turnover, but to make turnover safe for economic activity. This requires that institutions be reformed to guarantee equal treatment for all economic actors—a difficult process that has proceeded with fits and starts in post-Yanukovych Ukraine.
References
- Earle, John S.; and Scott Gehlbach, 2015. “The Productivity Consequences of Political Turnover,” American Journal of Political Science, 59(3), 708–723.
- Hale, Henry E, 2005. “Regime Cycles: Democracy, Autocracy, and Revolution in Post-Soviet Eurasia,” World Politics, 58(1), 133–65.
And the Lights Went Out – Measuring the Economic Situation in Eastern Ukraine

This policy brief assesses the economic situation in the war-affected East of Ukraine. Given that official statistics are not available, we use changes in nighttime light intensity, measured by satellites, to estimate to what extent the war has destroyed the economy, and whether any recovery can be observed since the Minsk II agreement.
This FREE Policy Brief is simultaneously published as a column at VoxUkraine.org/en.
Correct measurement of economic performance is difficult enough in peaceful times and in scenarios, in which reliable economic indicators are available. However, when the necessary data is missing or when its reliability is far from clear, assessing the degree of economic activity – even in the most crude of forms – becomes a significant challenge. And yet, such situations are very frequent, apply to many countries and regions and become most evident at times of military conflicts when data collection is far from a top priority. In the context of the Ukrainian conflict an example of indirectly estimating changes in economic performance can be found in Talavera & Gorodnichenko (2016) who focus on measures of the degree of price integration in the so called Luhansk and Donetsk National Republics (LNR/DNR). In addition, there are various articles using anecdotal evidence to illustrate the economic losses in the East of Ukraine. For example, BBC, 2015 mentions an estimate by the Ukrainian Ministry of Economy that by mid 2015, 50% to 80% of jobs were lost in the so-called Luhansk and Donetsk National Republics, compared to the pre-war situation. Knowing the economic situation in the East is important both to assess the economic viability of the so-called Luhansk and Donetsk National Republics (LNR/DNR) as well as to assess the likely humanitarian situation in the East.
An alternative indirect way to examine the intensity of economic activity is to use measures based on satellite nighttime light intensity images. Nighttime light intensity is closely related to electricity consumption, which often has been used as an indicator of economic activity (e.g. Arora and Lieskovsky, 2014). Nighttime light intensity has been used to assess economic activity in sub-Saharan Africa (Henderson et al., 2012), the impact of the crisis in Syria (Li and Li, 2014) or to study how elected politicians favour their own regions worldwide (Hodler and Raschky, 2014). Henderson et al. (2012) find that among low- and middle-income countries, a one percent change in light roughly corresponds to a one percent change in income. [1]
In this note we use nighttime light intensity to measure economic activity in Eastern Ukraine since the outbreak of the war in the East of Ukraine in April 2014.[2] As a reference point we use the nighttime light intensity in March 2014, prior to the outbreak of violence in the East of Ukraine, and we focus on Ukraine’s capital Kyiv and a number of big and small cities in Eastern Ukraine, which we know have been heavily affected by the conflict. In table 1, we compare the light intensity at several points in time (May 2014; August 2014; January 2015; March 2015; March 2016) to the light intensity in March 2014 in these selected cities.
Figure 1. Nighttime images of Kyiv (a), Donetsk (b), and Luhansk (c) in March 2014, 2015, and 2016
(a) Kyiv | ||
March 2014 | March 2015 | March 2016 |
![]() |
![]() |
![]() |
(b) Donetsk | ||
March 2014 | March 2015 | March 2016 |
![]() |
![]() |
![]() |
(c) Luhansk | ||
March 2014 | March 2015 | March 2016 |
![]() |
![]() |
![]() |
Notes: Radiance was linearly scaled from 0 to 10 nW/cm2/sr, where black pixels represent 0 and white represent 10 or more nW/cm2/sr. Administrative boundaries for cities: © OpenStreetMap contributors, CC BY-SA.
Figure 1 presents sample images of nighttime illumination for Kyiv, Donetsk and Luhansk in March 2014, 2015 and 2016. We can see that between March 2014 and 2015, in the case of Donetsk and Luhansk, both the surface area lit as well as the measured light intensity significantly decreased, while there is very little change in the case of Kyiv. A similar picture emerges in other cities that were not directly affected by the war, such as, for example Zaporizhia, Dnipropetrovsk and Kharkiv (see Table 1). While, as in Kyiv, there are ups and downs in terms of measured nighttime light intensity, by and large, the level of economic activity remains fairly similar over time.
Table 1. Change in nighttime light intensity across time for selected cities in Ukraine
Notes: The numbers in the table are ratios of light intensity, comparing a given point in time to March 15, 2014. Hence, number 1 suggests no change, numbers above 1 suggest improvements, and numbers below 1 suggest decreases in economic activity.
The situation is clearly different in Donetsk and Luhansk, the two major occupied towns. Nighttime light intensity in Donetsk is about half of the level it was before the outbreak of violence in the East of Ukraine. Luhansk fares even worse – light intensity as measured in March 2015 and 2016 is roughly a third of the initial level (Table 1).
Ilovaisk and Debaltseve, two cities where major battles took place and which are now under control of the so-called DNR/LNR, clearly have suffered a lot and are still far from recovering. Illovaisk is at about a third of its original level of light intensity, while Debaltseve is at less than a tenth (!) of the level in 2014. It is thus clear that economic recovery in these areas takes a long time, and that this is also true for the government controlled areas. This is illustrated by the fact that cities such as Sloviansk and to a lesser extent Kramatorsk are also still far away from their pre-conflict level of light intensity.
Conclusion
The above analysis of changes in nighttime light intensity data leads to two important conclusions. First, the impact of the war in Eastern Ukraine on the level of economic activity in the area is sizeable and varies considerably across towns. Levels of nighttime light intensity are at 30 to 50% of their pre-war level in the big cities and at only a tenth of their pre-war level in some smaller cities. Using the Henderson et al. (2012) one to one ratio of changes in nighttime light intensity and economic development, this suggest the economic activity in the Donbas region has similarly dropped in economic terms to 30 to 50% of the pre-war level for the big cities and to only a tenth of the pre-war level for some smaller cities. [3]
Second, there has been no sign of economic recovery in the region since the Minsk I and II agreements. Even though military activity in the Donbas region has decreased compared to the period April 2014-February 2015, the economy – at least as measured by the intensity of lights – has not been improving and the economic situation of the Donbas population remains very far from what it used to be before the war.
______________________________________________________
[1] ‘The elasticity of growth of lights emanating into space with respect to income growth is close to one (p. 1025)’
[2] We use version 1 nighttime monthly data from the Visible Infrared Imaging Radiometer Suite (VIIRS) Day/Night Band (DNB) generated by the Earth Observation Group at NOAA National Geophysical Data Center and made publically available for download.
[3] Given the specificity of light intensity measures, we focus on changes between periods rather than levels because light intensity is computed as the sum of radiance over a selected area, and hence the level of intensity depends on the scale of the area. For comparisons over time, we always use the same geographic area. It is important to remember that these changes are proxies only since changes in light intensity can be sensitive to weather conditions over time. Thus, to be able to make informative judgement on the basis of these data, we focus on the broad picture that emerges from the data, rather than on specific values.
References
- Arora, Vipin and Jozef Lieskovsky (2014), “Electricity Use as an Indicator of U.S. Economic Activity”, U.S. Energy Information Administration Working Paper.
- BBC (2015) – Ukrainian Service, ‘ One year after the referendum DNR/LNR: Economic Losses’, May 12 2015.
- Henderson, J. Vernon , Adam Storeygard, and David N. Weil (2012), Measuring Economic Growth from Outer Space, American Economic Review 2012, 102: 994–1028
- Hodler, Roland, and Paul A. Raschky (2014), Regional Favouritism. Quarterly Journal of Economics 129: 995-1033.
- Talavera, Oleksandr and Yuriy Gorodnichenko (2016), How’s DNR Economy Doing, VoxUkraine April 7, 2016
- Xi Li & Deren Li (2014) Can night-time light images play a role in evaluating the Syrian Crisis?, International Journal of Remote Sensing, 35: 6648-6661.
Effects of Trade Wars on Belarus

The trade wars following the 2014 events in Ukraine affected not only the directly involved participants, but also countries like Belarus that were affected through international trade linkages. According to my estimations based on a model outlined in Ossa (2014), these trade wars led to an increase in the trade flow through Belarus and thereby an increase of its tariff revenue. At the same time, because of a ban on imports in the sectors of meat and dairy products, the tariff revenue of Russia declined. As a member of the Eurasian Customs Union (EACU), Belarus can only claim a fixed portion of its total tariff revenue. Since the decline in the tariff revenue of Russia led to a decline in the total tariff revenue of the EACU, there was a decrease in the after-redistribution tariff revenue of Belarus. As a result, Belarusian welfare decreased. To avoid further welfare declines, Belarus should argue for a modification of the redistribution schedule. Alternatively, Belarus could increase its welfare during trade wars by shifting from being a part of the EACU to only being a part of the CIS Free Trade Area (FTA). If Belarus was only part of the CIS FTA, the optimal tariffs during trade wars should be higher than the optimal tariffs without trade wars. The optimal response to the increased trade flow through Belarus is higher tariffs.
Following the political protests in 2014, Ukraine terminated its membership in the CIS Free Trade Area (FTA) and moved towards becoming a part of the EU. The political protests evolved into an armed conflict and a partial loss of Ukrainian territory. These events led to Western countries introducing sanctions against some Russian citizens and enterprises. In response, Russia introduced a ban on imports from EU countries, Australia, Norway, and USA in the sectors of meat products, dairy products, and vegetables, fruits and nut products. In addition, both Ukraine and Russia increased the tariffs on imports from each other in the above-mentioned sectors.
Clearly, the trade wars affected directly involved participants such as the EU countries, Russia, and Ukraine. At the same time, countries like Belarus that were not directly involved in the trade wars, were also affected because of international trade linkages. It is important to understand the influence of trade wars on none-participating countries. To address this question, a framework with many countries and international trade linkages will be utilized and I will in this policy brief present some of my key findings.
Framework and Data
To evaluate the effects of the trade wars, I use the methodology outlined in Ossa (2014). This framework is based on the monopolistic competition market structure that was introduced into international trade by Krugman (1979, 1981). The framework in Ossa (2014) allows for many countries and sectors, and for a prediction of the outcome if one or several countries changes their tariffs. Perroni and Whallye (2000) and Caliendo and Parro (2012) present alternative frameworks with many countries that can also be used to estimate the welfare effects of tariff changes. The important advantage of the framework introduced in Ossa (2014) is that only data on trade flows, domestic production, and tariffs are needed to evaluate the outcomes of a change in tariffs, though the model itself contains other variables like transportation costs, the number of firms, and productivities.
It should also be pointed out that the framework in Ossa (2014) is not an example of a CGE model as it does not contain features such as investment, savings, and taxes. Since the framework in Ossa (2014) is simpler than CGE models, the effects of a tariff change can more easily be tracked and interpreted. On the other hand, this framework does not take into account spillover effects of tariff changes on for example capital formation and trade in assets.
The data on trade flows and domestic production come from the seventh version of the Global Trade Analysis Project database (GTAP 7). The data on tariffs come from the Trade Analysis Information System Data Base (TRAINS). The estimation of the model is done for 47 countries/regions and the sectors of meat and dairy products.
Results
According to my estimations, because of the ban on imports by Russia, the trade flow through Belarus increased. Belarusian imports of meat products are estimated to have increased by 28%, and imports of dairy products by 47%. Such increases in imports mean an increase in the tariff revenue of Belarus. It should be pointed out, however, that the model only tracks the effects of the ban on imports in the sectors of meat and dairy products. An alternative way would be to construct an econometric model that takes into account different factors influencing the trade between the countries. The effects of the decrease in the price of oil and the introduced ban on imports, which happened close in time, could then have been evaluated.
The estimated model further predicts that, because of the ban on imports, the tariff revenue collected by Russia in these two sectors has decreased by 53%. This means that since Belarus can only claim a fixed portion (4.55%) of the total tariff revenue of the EACU, its after-redistribution tariff revenue collected in the meat and dairy product sectors declined by 44.86%, in spite of its increase in before-redistribution tariff revenue by 35%. The decline in Belarus’ after-redistribution tariff revenue is thus estimated to have led to a decrease in welfare by 0.03%. To prevent such a decrease in the future, Belarus should argue for an increase in its share of the total tariff revenue of the EACU.
Furthermore, in addition to the decrease in the tariff revenue, the estimated model predicts that the real wage in Russia decreased by 0.39%, and its welfare by 0.49%.
The introduced ban on imports also affected the European countries that used to export to Russia. The model predicts that the welfare of Latvia declined by 0.38% and that the welfare of Lithuania declined by 0.27%. A substantial portion of the decline in welfare of these countries can be explained by a decrease in their terms of trade. The introduced ban on imports by Russia led to a decline in prices in the countries that exported meat and dairy products to Russia. Lower prices led to a decrease in the proceeds from exports collected by EU countries, and lower proceeds from exports buy less import, implying a decrease in their welfare.
In spite of the increase in tariffs between Russia and Ukraine, the model predicts an increase in the welfare of Ukraine by 0.23% following the formation of the EU-Ukraine Deep and Comprehensive Free Trade Area (DCFTA). An increase in real wages by 0.34% is the main factor contributing to this welfare increase. This is because it is associated with a redirection of Ukrainian exports from Russia towards the EU. The predicted increase in real wages in Ukraine have not materialized so far, presumably because of the ongoing military conflict and because time is needed to redirect the trade flows in response to the changes in the tariffs.
While bearing in mind that the analysis is only based on the sectors of meat and dairy products, Belarus could have increased its welfare during the trade wars if it had shifted from EACU status back to CIS FTA status with tariffs set at before-EACU levels. In this case, Belarus would not have needed to share its tariff revenue with other countries, and would then have increased its tariff revenue by 47.93% instead of the now predicted decline by 44.86%. Similarly, the welfare during trade wars could then have increased by 0.05%, instead of the now predicted decline by 0.03%. Another advantage of moving to CIS FTA status during trade wars is that the real wage could have increased by 0.04% instead of the 0.003% in the case of continued EACU status. Belarus could further have benefitted from moving to CIS FTA status by choosing optimal tariffs. This study suggests that the optimal tariffs of Belarus under CIS FTA status with trade wars are higher than the optimal tariffs under CIS FTA status without trade wars. Higher tariffs is the optimal response to the increased trade flows through Belarus resulting from trade wars.
Conclusion
Although it is optimal to move to CIS FTA status during trade wars, it is optimal to move back to EACU status after the trade wars are over. Therefore, such a policy should be adopted with caution, since the shift back to EACU status will likely not be possible. If it is expected that the trade wars will continue for a long period of time, or if the other members of the EACU will often deviate from the common tariffs, a transition to CIS FTA should be adopted. At the same time, asking for an increase in its share of total tariff revenue of EACU is a feasible strategy for Belarus to follow.
While estimating the effect of a transition from EACU status to CIS FTA status for Belarus during trade wars, the evaluation was done using two sectors affected by counter-sanctions. To evaluate the full welfare effect of this transition, its effect on the other sectors of Belarus should also be estimated, which is a question for the further research.
Is War Good for a Country’s Political Institutions?

Author: Tom Coupe, KSE.
Recent research suggests that experiencing war violence might make people more likely to turn out during elections. Using data from the conflict in Eastern Ukraine, we show, however, that people who were injured or had close friends or relatives killed or injured were less likely to turn out at the 2014 parliamentary elections. We also show that the impact of violence on turn out and political views depends on the type of violence one experienced.