Tag: Industrial organization
Land Market and a Pre-emptive Right in Farmland Sales
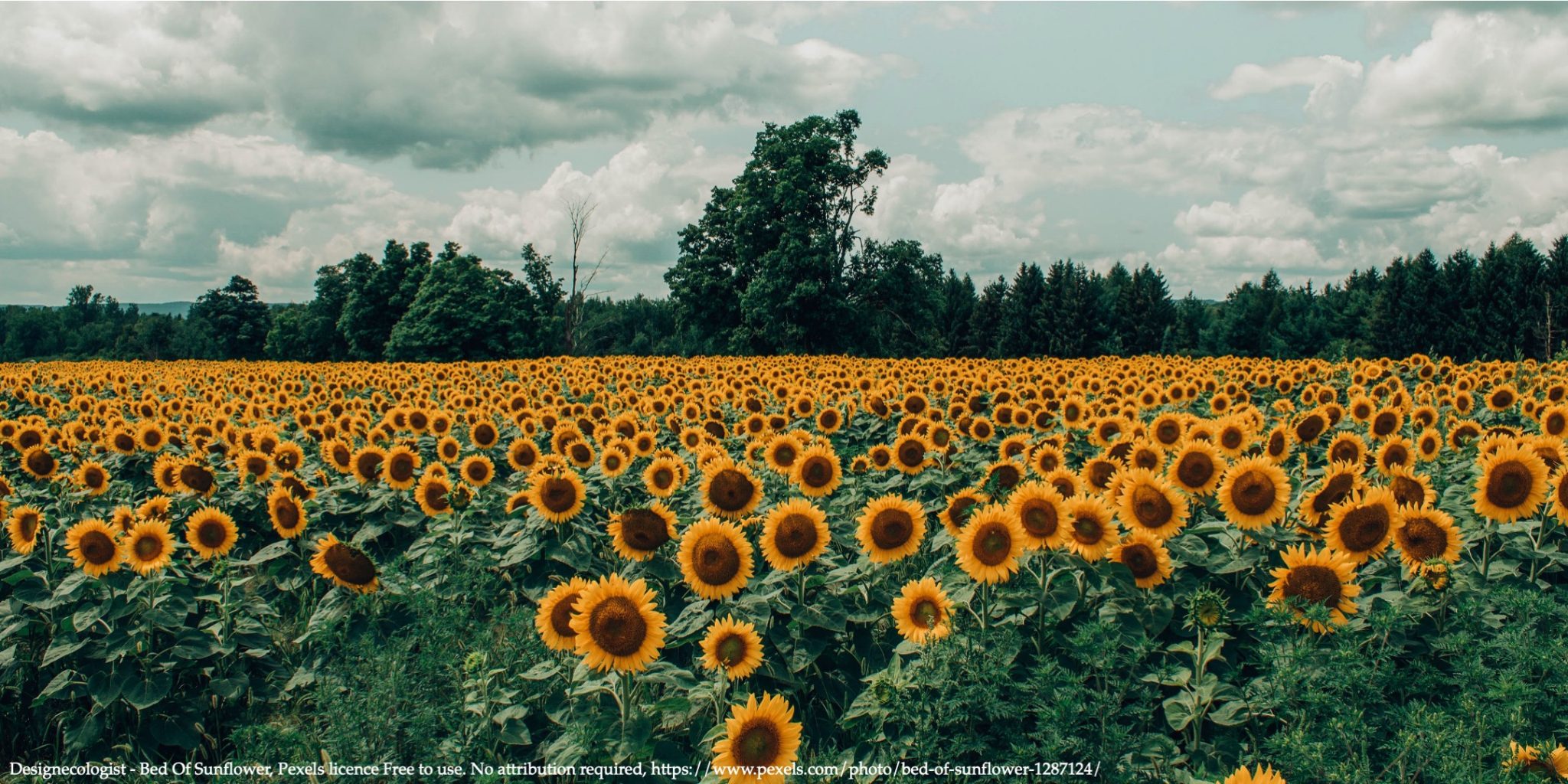
After more than 20 years of a land sales ban, Ukraine finally opened its farmland market on July 1st, 2021. A design of the land market contains a pre-emptive right to buy the land for the farmland tenants. In this study, we model the effect of this pre-emptive right. Following the approach of Walker (1999), we use a theoretical model with three players – landowner, potential buyer, and the tenant – to model outcomes of the land transactions with and without the pre-emptive right. To empirically estimate the effect of the pre-emptive right, we use farm-level data to derive farmers’ maximum willingness to pay and the minimum price that landowners are willing to accept. The introduction of the pre-emptive right decreases the land price and increases the tenant’s chances of winning as well as his surplus, at the cost of a potential buyer and the landowner. The introduction of the pre-emptive right also leads to inefficient distribution and deadweight losses to the economy.
Introduction
After more than 20 years of a land sales ban, Ukraine finally opened its farmland market on July 1st, 2021. The moratorium on the sales of agricultural land in Ukraine covered of 96% of the country’s farmland market (or 66% of its entire territory).
The critical element of the newly opened Ukrainian farmland market design is the pre-emption right (right of the first refusal, RoFR) that is granted to the current tenant of land plots. By applying their pre-emptive right, tenants can purchase the land at the highest price the landowner could get on the market. On top of that, this right is transferable, meaning that the tenant could sell the right to the interested party. In this brief, we model the consequences of the pre-emptive right introduction in Ukraine.
Farmland Market in Ukraine
The moratorium on farmland sales that was in place for the last 20 years created a substantial distortion on the farmland market. It led to the situation where large companies predominantly cultivate the rented land, with the average share of leased land in the land bank for corporate farms in Ukraine approaching 99% (Graubner et al., 2021). Another noticeable trait of the farmland market in Ukraine is significant inequality in Ukrainian farms’ land banks. Based on the statistical forms 50AG, 29AG, and 2farm, our calculations show that the GINI index for the allocation of cultivated land across farms in Ukraine is 86%, indicating an extreme degree of inequality. As we can see from Table 1 – the top 10% of farms operate on 75% of all cultivated farmland in Ukraine. On the other side of the spectrum, 49% of the smallest farms in Ukraine operate on only 2% of the cultivated farmland and rent only 0,3% of all rented farmland.
Table 1. Ukrainian farmland market structure
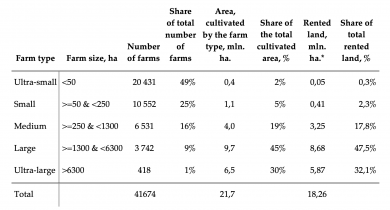
Source – own calculations based on the statistical forms 50AG, 29AG, 2farm for the year 2016.
Therefore, in our analysis, we break a sample of Ukrainian farms into five categories with respect to their size.
Framework
To model the effect of the pre-emptive right, we will use the approach proposed by Walker (1999) using farm-level data. Thus, this study compares two scenarios – with the pre-emptive right (right of the first refusal, RoFR) and without the pre-emptive right in place. We assume that there are only three sides to each transaction – the seller (landowner), the prospective buyer, and the tenant, to whom the pre-emptive right is granted. Throughout this brief, we assume that there are no transaction costs involved.
Scenario 1. No Pre-emptive Right
In the no-RoFR scenario, the prospective buyer offers the landowner a price that the seller is willing to accept. The seller now has two options: either accept and get the offered price or reach the tenant and propose to outbid this offer. The option of reaching a tenant is more attractive since, in a worst-case scenario, if the tenant’s valuation – i.e., the maximum price the tenant is willing to pay for the land plot – is lower than the offered price, the tenant would simply not respond to this offer, and the landlord still gets the offered price.
On the other hand, if the tenant’s valuation is higher than the offered price, he has a strong incentive to make the counteroffer and start a bidding process. Both the tenant and the prospective buyer are incentivized to make a counteroffer up until the point where the offer’s value reaches their respective valuation. Thus, the smallest valuation between those of the tenant and prospective buyer would be the final transaction price.
Scenario 2. A Tenant Has the Pre-emptive Right
In this scenario, the tenant does not need to increase the price in his counteroffer if the third-party buyer’s offer is lower than the tenant’s valuation. The tenant could execute his pre-emptive right and buy the plot at the third-party buyer’s proposed price. Therefore, the outside buyer will change his approach to the initial offer. If the offer he makes is “too low”, he loses the chance of buying this plot since the tenant would exercise his pre-emptive right. If the offer is “too high,” he misses the profit he would make by making a lower offer.
In such circumstances, the transaction price will be given by the third-party buyer’s offer that maximizes his expected profit. The latter, in turn, depends on the probability of the tenant exercising his preemptive right, the third-party buyer’s own valuation, and the price he offers to the landlord. The probability of the tenant exercising the offer is the probability that the tenant’s valuation exceeds the offered price. It depends on the tenant’s farm size category and on the offer itself and can be calculated based on the distribution of valuations.
Empirical Approach
Our empirical analysis considers a (hypothetical) situation of a third-party buyer coming to the landowner, whose land is rented to another farmer, with the offer to buy a one-hectare plot. We assume that the offer exceeds the landowner’s minimum price that a landowner is willing to accept (WTA). The landowner’s WTA is proxied by the current rental price the landlord gets multiplied by the capitalization rate, set to 20 for all three sides of the transaction. The farmers’ valuations are estimated based on their net profit per hectare. We use the farm-level data to compute the average net profit per hectare needed for valuations estimation and the average rental price per hectare for the WTA estimation. This data was collected by the State Statistics Service of Ukraine through statistical questionnaires called 50AG, 29AG, and 2farm for the year 2016 and covers 39,297 farms. The descriptive statistics of the data are presented in table 2.
Table 2. Descriptive statistics
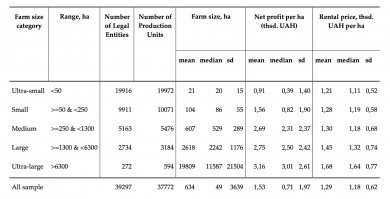
Source: own calculations based on the statistical forms 50AG, 29AG, 2farm for the year 2016.
We construct a set of potential buyers for each farm that operates on rented land based on the 10-km threshold distance between the tenant and third-party buyer. We end up with a sample of 764760 pairs of tenants and potential third-party buyers. We drop all pairs where third-party buyers cannot make an offer landlord is willing to accept. Therefore, only a sample of 291506 observations of tenant – prospective buyer pairs is used for the analysis. Importantly, for large and ultra-large farms, the share of observations that would attempt a transaction is 70% and 69% correspondingly. On the lower side of the size spectrum, this share is noticeably lower. For the group of small third-party buyers, the buyer would attempt the transaction only in 42% of cases. The most excluded from the farmland sales market category are ultra-small farms as they would only attempt the transaction in 25% of all cases.
Results
Our findings suggest that the effect of the pre-emptive right on the land price is twofold. On the one hand, in 55% of cases – the RoFR price is higher than the (modelled auction) price in the absence of a preemptive right. However, the median price differences in these cases are just 0,7% of the auction price. At the same time, for the cases where the auction price is higher than the price with the RoFR, it exceeds the RoFR price, on average, by 83%, with a median value of 66%. As a result, if we compare the expected prices, the expected prices under the RoFR are significantly lower than the auction prices. There are also differences between different farm size categories of the third-party buyer – the larger the buyer is, the higher the transaction price would be regardless of the RoFR. In the scenario without the RoFR, the average transaction price for ultra-small farms would be $1259 per hectare. While for the ultra-large farm as the third-party buyer, the transaction price would be $1647. With the pre-emptive right granted to the tenant, the transaction prices would be $977 and $1313 correspondingly.
The pre-emptive right also increases the probability of the tenant acquiring the land. The most noticeable effect is for ultra-small and small farms – if an outside buyer attempts the transaction, their chances of purchasing the land increase from 12% to 28% and from 23% to 45%, respectively. The probability increase for the larger tenants persists, but percentage-wise it is smaller – their probability of purchasing the land due to the granted pre-emptive right increases from 42-45% to 65-66%.
The pre-emptive right also redistributes the surplus from the transaction. Measuring the surplus as the difference between the valuation and the buyer’s actual purchase price, we can conclude that the third party’s surplus decreased due to the RoFR introduction. The tenant’s surplus, on the other hand, increases. In the case of RoFR introduction, the percentage increase in the tenant’s surplus is larger for the ultra-small and small farmers, from 5% to 13% and from 10% to 23% of the tenant’s valuation, respectively. For larger farms, albeit the surplus’ increase is larger in absolute terms, percentage-wise, it is smaller than for their smaller counterparts. Their average surplus increased from 18-20% to 37-38% of the tenant’s valuation. For the third-party buyers, the percentage-wise decrease is more or less the same, regardless of their farm size. Their surpluses, on average, shrink by 23-27% depending on the size of the farm.
We also estimated the effect of the pre-emptive right on the joint surplus of the landlord and the tenant. The effect of the pre-emptive right on their joint surplus is positive regardless of the size category of the tenant. The largest increase of the joint surplus, percentage-wise, is observed for the small-sized farms as a tenant. In this case, the average joint surplus increased by 5%, translating into an $87 increase in the joint surplus. In absolute terms, the highest increase is for medium-sized farms as a tenant – $108 increase in the surplus or 4.5% of their original joint surplus.
The pre-emptive right also leads to inefficient allocations when the land is acquired by a lower valuation party, resulting in deadweight losses. Inefficient allocation is observed in 19% of all observations. The deadweight losses generated by the introduction of the ROFR are statistically significant (with the t-value equal to 195) and average 233 USD per hectare.
Conclusions
In this brief, we suggest a theoretical and analytical approach to calculate the impact of the pre-emptive right in farmland sales. Our analysis offers a range of important findings. First, small and medium-sized farms are almost entirely excluded from the farmland market. While more than two-thirds of the medium, large or ultra-large farms can afford to buy a nearby parcel, based on their profitability – for ultra-small farms, which have a land bank of under 50 hectares – this share is equal to just 25%. The introduction of the pre-emptive right granted to the current tenant may exaggerate this problem. The reason is that most of the rented land is already controlled by large and ultra-large companies. At the same time, the pre-emptive right increases the tenant’s probability of winning and its surplus at the expense of the landowner and outside buyer.
On the other hand, the pre-emptive right increases the joint surplus of the tenant and the landowner. Therefore, if the pre-emptive right would be a voluntaristic clause in the contract, rather than a right granted to all tenants by the government, it creates an incentive to include the pre-emptive right in the rental agreement with the price of this right negotiated between the landlord and the tenant.
Summing up, the pre-emptive right, as a policy instrument, has its costs. It leads to inefficient distribution and deadweight losses. In view of this, as much as the recent farm market reform in Ukraine is a clear step towards a market economy, the design of the land market should be taken with a grain of salt.
References
- Graubner, Marten, Igor Ostapchuk and Taras Gagalyuk, 2021. “Agroholdings and land rental markets: a spatial competition perspective”, European Review of Agricultural Economics, 48(1), 158-206
- Walker, David, 1999. “Rethinking rights of first refusal“, Stanford Journal of Law, Business & Finance, 5, 1-58.
Disclaimer: Opinions expressed in policy briefs and other publications are those of the authors; they do not necessarily reflect those of the FREE Network and its research institutes.
Energy Storage: Opportunities and Challenges
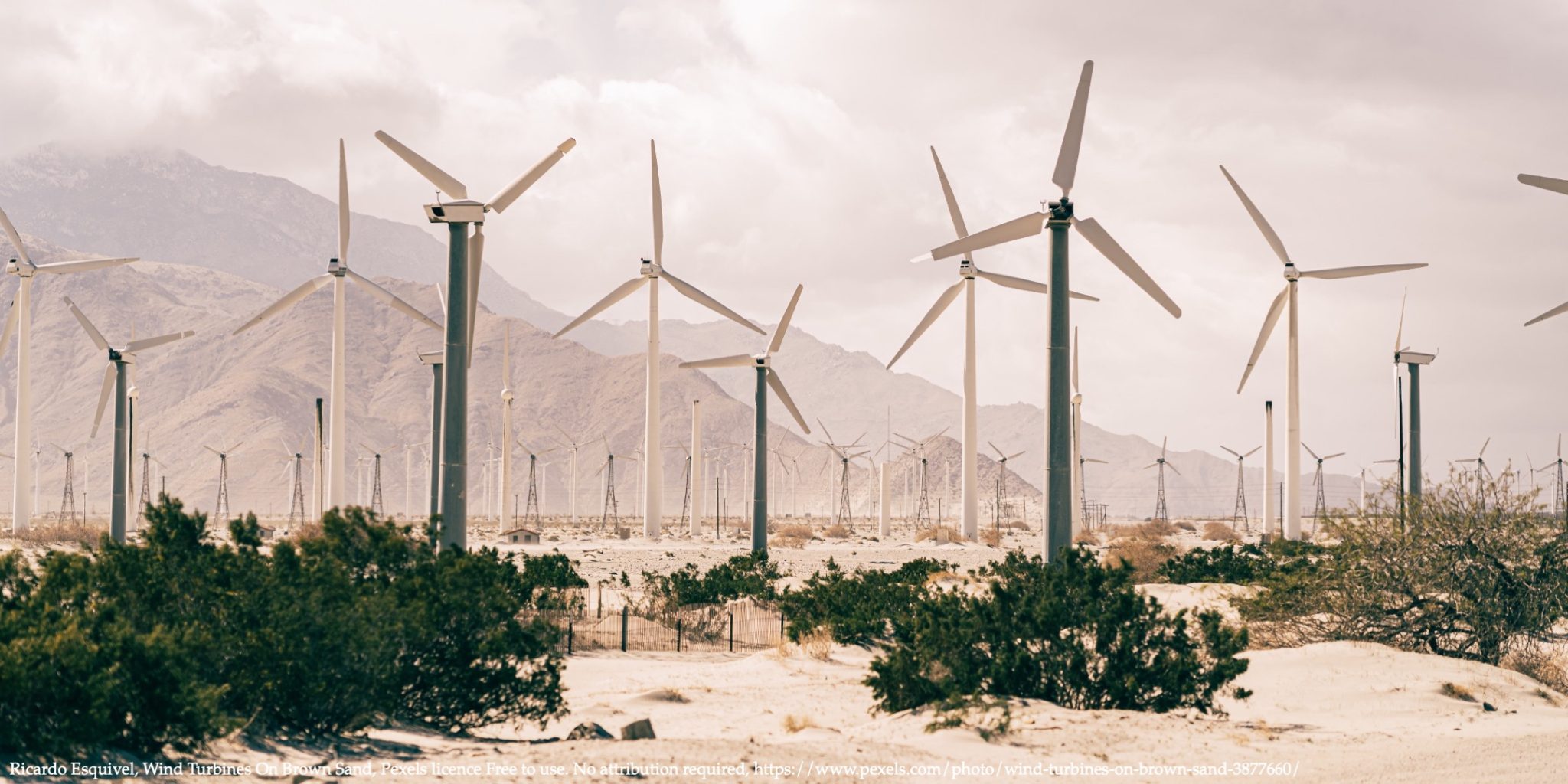
As the dramatic consequences of climate change are starting to unfold, addressing the intermittency of low-carbon energy sources, such as solar and wind, is crucial. The obvious solution to intermittency is energy storage. However, its constraints and implications are far from trivial. Developing and facilitating energy storage is associated with technological difficulties as well as economic and regulatory problems that need to be addressed to spur investments and foster competition. With these issues in mind, the annual Energy Talk, organized by the Stockholm Institute of Transition Economics, invited three experts to discuss the challenges and opportunities of energy storage.
Introduction
The intermittency of renewable energy sources poses one of the main challenges in the race against climate change. As the balance between electricity supply and demand must be maintained at all times, a critical step in decarbonizing the global energy sector is to enhance energy storage capacity to compensate for intermittent renewables.
Storage systems create opportunities for new entrants as well as established players in the wind and solar industry. But they also present challenges, particularly in terms of investment and economic impact.
Transitioning towards renewables, adopting green technologies, and developing energy storage can be particularly difficult for emerging economies. Some countries may be forced to clean a carbon-intensive power sector at the expense of economic progress.
The 2021 edition of Energy Talk – an annual seminar organized by the Stockholm Institute of Transition Economics – invited three international experts to discuss the challenges and opportunities of energy storage from a variety of academic and regulatory perspectives. This brief summarizes the main points of the discussion.
A TSO’s Perspective
Niclas Damsgaard, the Chief strategist at Svenska kraftnät, gave a brief overview of the situation from a transmission system operator’s (TSO’s) viewpoint. He highlighted several reasons for a faster, larger-scale, and more variable development of energy storage. For starters, the green transition implies that we are moving towards a power system that requires the supply of electricity to follow the demand to a much larger extent. The fact that the availability of renewable energy is not constant over time makes it crucial to save power when the need for electricity is low and discharge it when demand is high. However, the development and facilitation of energy storage will not happen overnight, and substantial measures on the demand side are also needed to ensure a more dynamic energy system. Indeed, Damsgaard emphasized that demand flexibility constitutes a necessary element in the current decarbonization process. However, with the long-run electrification of the economy (particularly driven by the transition of the transport industry), extensive energy storage will be a necessary complement to demand flexibility.
It is worth mentioning that such electrification is likely to create not only adaptation challenges but also opportunities for the energy systems. For example, the current dramatic decrease in battery costs (around 90% between 2010 and 2020) is, to a significant extent, associated with an increased adoption of electric vehicles.
However, even such a drastic decline in prices may still fall short of fully facilitating the new realities of the fast-changing energy sector. One of the new challenges is the possibility to store energy for extended periods of time, for example, to benefit from the differences in energy demand across months or seasons. Lithium-ion batteries, the dominant battery technology today, work well to store for a few hours or days, but not for longer storage, as such batteries self-discharge over time. Hence, to ensure sufficient long-term storage, more batteries would be needed and the associated cost would be too high, despite the above-mentioned price decrease. Alternative technological solutions may be necessary to resolve this problem.
Energy Storage and Market Structure
As emphasized above, energy storage facilitates the integration of renewables into the power market, reduces the overall cost of generating electricity, and limits carbon-based backup capacities required for the security of supply, creating massive gains for society. However, because the technological costs are still high, it is unclear whether the current economic environment will induce efficient storage. In particular, does the market provide optimal incentives for investment, or is there a need for regulations to ensure this?
Natalia Fabra, Professor of Economics and Head of EnergyEcoLab at Universidad Carlos III de Madrid, shared insights from her (and co-author’s) recent paper that addresses these questions. The paper studies how firms’ incentives to operate and invest in energy storage change when firms in storage and/or production have market power.
Fabra argued that storage pricing depends on how decisions about the storage investment and generation are allocated between the regulator and the firms operating in the storage and generation markets. Comparing different market structures, she showed as market power increases, the aggregate welfare and the consumer surplus decline. Still, even at the highest level of market concentration, an integrated storage-generation monopolist firm, society and consumers are better off than without energy storage.
Fabra’s model also predicts that market power is likely to result in inefficient storage investment.
If the storage market is competitive, firms maximize profits by storing energy when the prices are low and releasing when the prices are high. The free entry condition implies that there are investments in storage capacity as long as the marginal benefit of storage investment is higher than the marginal cost of adding an additional unit of storage. But this precisely reflects the societal gains from storage; so, the competitive market will replicate the regulator solution, and there are no investment distortions.
If there is market power in either generation or storage markets, or both, the investment is no longer efficient. Under market power in generation and perfectly competitive storage, power generating firms will have the incentive to supply less electricity when demand is high and thereby increase the price. As a result, the induced price volatility will inflate arbitrage profits for competitive storage firms, potentially leading to overinvestment.
If the model features a monopolist storage firm interacting with a perfectly competitive power generation market, the effect is reversed. The firm internalizes the price it either buys or sells energy, so profit maximization makes it buy and sell less energy than it would in a competitive market, in the exact same manner as the classical monopolist/monopsonist does. This underutilization of storage leads to underinvestment.
If the model considers a vertically integrated (VI) generation-storage firm with market power in both sectors, the incentives to invest are further weakened: the above-mentioned storage monopolist distortion is exacerbated as storage undermines profits from generation.
Using data on the Spanish electricity market, the study also demonstrated that investments in renewables and storage have a complementary relationship. While storage increases renewables’ profitability by reducing the energy wasted when the availability is excess, renewables increase arbitrage profits due to increased volatility in the price.
In summary, Fabra’s presentation highlighted that the benefits of storage depend significantly on the market power and the ownership structure of storage. Typically, market power in production leads to higher volatility in prices across demand levels; in turn, storage monopolist creates productive inefficiencies, two situations that ultimately translate into higher prices for consumers and a sub-optimal level of investment.
Governments aiming to facilitate the incentives to invest in the energy storage sector should therefore carefully consider the economic and regulatory context of their respective countries, while keeping in mind that an imperfect storage market is better than none at all.
The Russian Context
The last part of the event was devoted to the green transition and the energy storage issue in Eastern Europe, with a specific focus on Russia.
Alexey Khokhlov, Head of the Electric Power Sector at the Energy Center of Moscow School of Management, SKOLKOVO, gave context to Russia’s energy storage issues and prospects. While making up for 3% of global GDP, Russia stands for 10% of the worldwide energy production, which arguably makes it one of the major actors in the global power sector (Global and Russian Energy Outlook, 2016). The country has a unified power system (UPS) interconnected by seven regional facilities constituting 880 powerplants. The system is highly centralized and covers nearly the whole country except for more remote regions in the northeast of Russia, which rely on independent energy systems. The energy production of the UPS is strongly dominated by thermal (59.27%) followed by nuclear (20.60%), hydro (19.81%), wind (0.19%), and solar energy (0.13%). The corresponding ranking in capacity is similar to that of production, except the share of hydro-storage is almost twice as high as nuclear. The percentage of solar and wind of the total energy balance is insignificant
Despite the deterring factors mentioned above, Khokhlov described how the Russian energy sector is transitioning, though at a slow pace, from the traditional centralized carbon-based system towards renewables and distributed energy resources (DER). Specifically, the production of renewables has increased 12-fold over the last five years. The government is exploring the possibilities of expanding as well as integrating already existing (originally industrial) microgrids that generate, store, and load energy, independent from the main grid. These types of small-scaled facilities typically employ a mix of energy sources, although the ones currently installed in Russia are dominated by natural gas. A primary reason for utilizing such localized systems would be for Russia to improve the energy system efficiency. Conventional power systems require extra energy to transmit power across distances. Microgrids, along with other DER’s, do not only offer better opportunities to expand the production of renewables, but their ability to operate autonomously can also help mitigate the pressure on the main grid, reducing the risk for black-outs and raising the feasibility to meet large-scale electrification in the future.
Although decarbonization does not currently seem to be on the top of Russia’s priority list, their plans to decentralize the energy sector on top of the changes in global demand for fossil fuels opens up possibilities to establish a low-carbon energy sector with storage technologies. Russia is currently exploring different technological solutions to the latter. In particular, in 2021, Russia plans to unveil a state-of-the-art solid-mass gravity storage system in Novosibirisk. Other recently commissioned solutions include photovoltaic and hybrid powerplants with integrated energy storage.
Conclusion
There is no doubt that decarbonization of the global energy system, and the role of energy storage, are key in mitigating climate change. However, the webinar highlighted that the challenges of implementing and investing in storage are both vast and heterogenous. Adequate regulation and, potentially, further government involvement is needed to correctly shape incentives for the market participants and get the industry going.
On behalf of the Stockholm Institute of Transition Economics, we would like to thank Niclas Damsgaard, Natalia Fabra, and Alexey Khokhlov for participating in this year’s Energy Talk. The material presented at the webinar can be found here.
Disclaimer: Opinions expressed in policy briefs and other publications are those of the authors; they do not necessarily reflect those of the FREE Network and its research institutes.
Optimal Recommendation System with Competing Sellers
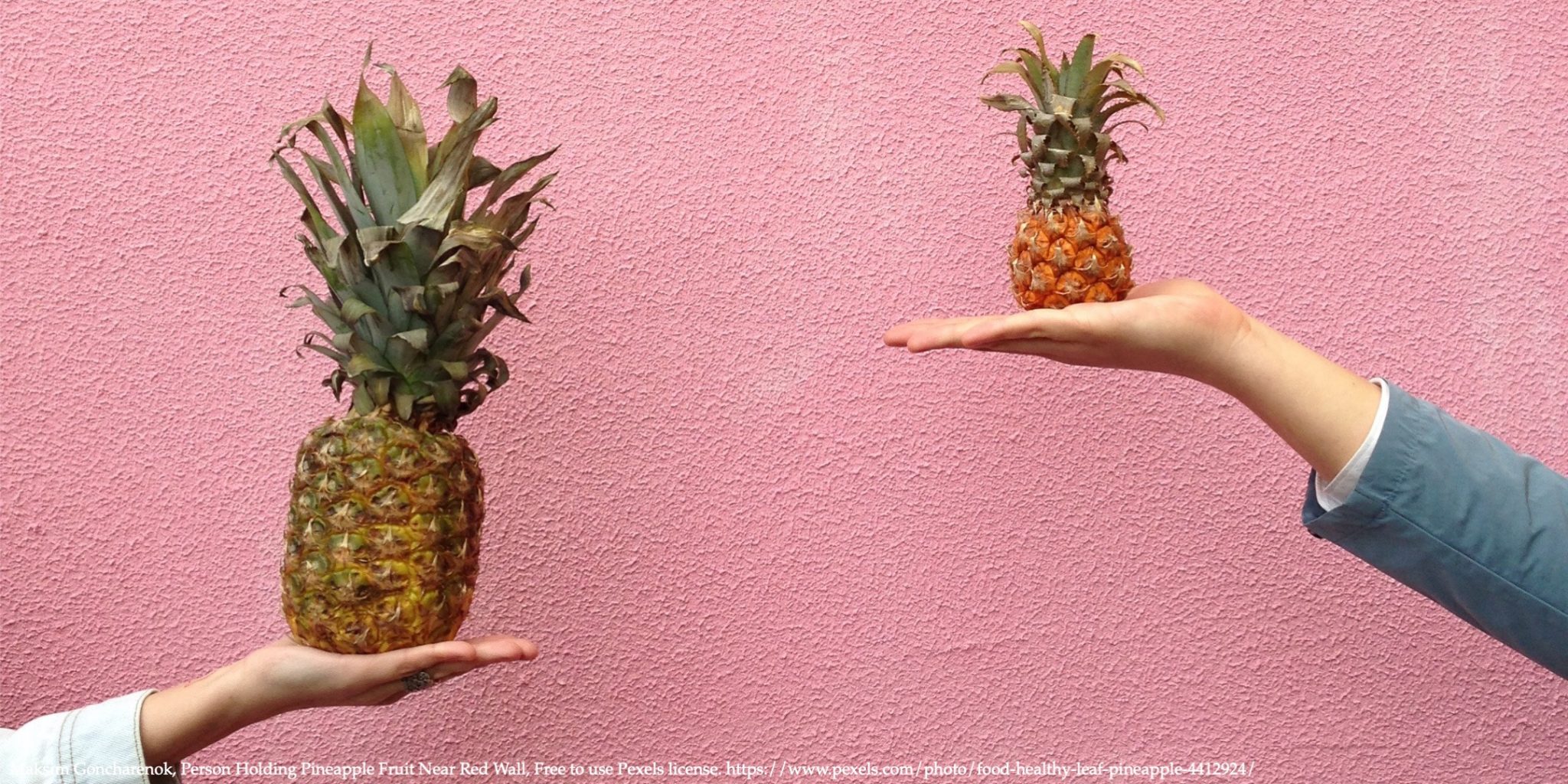
Many e-commerce platforms that connect buyers and sellers employ recommendation systems to help customers find products and services. Such platforms seek to maximize their profits which mainly comes from a commission on sales made via the platform. This may create incentives for platforms to use a recommendation strategy that suppresses competition among sellers and keeps prices and the resulting commission high. At the same time, the huge success of platforms such as Amazon suggests that they also care about customer satisfaction. Thus, the platform has an incentive to recommend goods that are cheaper and a better match for customer’s tastes. This requires not only sufficient competition between sellers but also that sellers act to improve the fit of their product to customer needs. Since these actions are typically costly, a high commission may disincentivize sellers to undertake them, thereby negatively affecting customers. Therefore, in designing the recommendation system and deciding on commissions, the platform should carefully balance the pro-competitive customer care and anti-competitive incentives to keep high prices and profits.
Introduction
When we search for a product on an e-commerce platform, such as Amazon or AliExpress, the default search outcome often contains a list of recommended products sold by vendors that are selected by the platform. The order of these sellers is, of course, not random – the platform’s decision on which sellers to recommend is strategic and there could be different forces driving such a strategy. For example, since the platform charges commission on sales, it may have an incentive to recommend the most expensive seller among those who sell similar products. At the same time, such a recommendation strategy, and high(er) prices in general, may negatively affect customer satisfaction from the marketplace and lead to a loss of its customer base. This is not in the best interest of the platform, especially if it wants to achieve long-term sustainability and growth.
The behavior of sellers adds a further layer to these considerations. Indeed, sellers are likely to adjust their pricing behavior and competitive strategies in response to a platform recommendation system.
These considerations give rise to two questions: First, how should an e-commerce platform design its recommendation system, or in other words, how does it optimally choose which sellers to recommend, which commission rate to set, etc.? Second, how does the presence of this system affect the competition and prices?
Further, a seller’s strategy may depend not only on the presence of recommendations but also on the commission rate set by the platform. Sellers usually have an option to perform costly actions in order to improve the match of their product to customers’ needs. For example, sellers may disclose more information on the characteristics of a good they are selling: spend time and money on detailed descriptions of their goods, or provide high-resolution photos. Though these actions are usually left at sellers’ discretion, they may substantially increase a customer’s satisfaction by improving the match between the purchased product and customer’s preferences.
In turn, a better fit may create a more loyal customer base for the seller, giving her more market power and increased profits. However, if the platform sets a high commission rate, sellers will have less incentive to undertake such costly actions (since the platform eats up a large share of the return to this action). This raises the questions – what is the optimal commission rate chosen by the platform, and how does the optimal commission rate affect sellers’ incentives to disclose information about their goods?
Another issue that arises here concerns the optimal precision of the recommendation system, that is, its ability to pin down customers’ tastes correctly. When the e-commerce platform deals with heterogeneous buyers, it should assess buyer’s preferences prior to making a recommendation. Although almost all research in Computer Science regarding recommendation systems focuses on how to make the precision as high as possible, I show that the highest level of precision may not be optimal from the platform’s perspective. Intuitively, this is because highly precise recommendation systems differentiate customers effectively, which in turn could give sellers local monopoly power and translate into higher prices. At the same time, an inaccurate recommendation system cannot distinguish customers with different preferences and views, which intensifies the competition by allowing sellers to compete for all customers.
In Fedchenko (2020), I address the abovementioned and other related issues on recommendation systems of e-commerce platforms. This brief summarizes the main findings of the study.
Model Description and Findings
In my model, I consider a platform that is designing a recommendation system. That is, for each seller, the platform chooses what share of customers end up receiving a recommendation to buy from this seller. This choice depends on the seller’s price, the quality of the good (if disclosed by the seller), and the buyers’ tastes. The platform also sets the commission rate it charges the sellers. I focus only on direct recommendations (i.e., the platform gives each buyer a unique recommendation). Although, in reality, platforms usually provide users with a ranking of alternatives, I assume that buyers always choose the top-ranked alternative which is equivalent to a single recommendation.
The model also assumes that a platform seeks to maximize the weighted sum of its profit (driven by commissions) and aggregate consumer surplus (motivated by the platform’s willingness to build a steady customer base). The (exogenous) weight assigned to the aggregate consumer surplus is referred to as the platform’s degree of consumer orientation (DCO). DCO is a measure of how much the platform cares about customer satisfaction and it plays an important role in determining the platform’s optimal recommendation strategy. In turn, customers have higher satisfaction if they buy a good that better fits their tastes, has higher quality, and is sold at a lower price.
Recommendation System Affects Competition
My model demonstrates that the presence of a recommendation system that charges sellers commission on sales (i.e. makes the platform have a stake in sellers’ profits) “softens” competition, and, in turn, increases prices. This effect is stronger the more a platform cares about its profits relative to customer satisfaction. The force that drives this result has already been touched upon in the introduction: if the platform has a stake in sellers’ profits, it will occasionally recommend sellers with higher prices. However, since the platform also cares about consumer surplus (which decreases if the price goes up) these high-priced recommendations will not go to all buyers, and therefore, the overall price level will not become too high. Still, the sellers are encouraged to set higher prices in this scenario, as compared to the hypothetical case in which customers know about the sellers without the platform.
Optimal Commission vs. Information Disclosure
The relationship between the commission rate and the seller’s decision on how much information to disclose is nontrivially affected by the DCO. If the DCO is high, then a higher commission rate causes sellers to disclose less information about their goods in equilibrium. If the DCO is low, the relationship is reversed: a higher commission rate increases the amount of disclosed information. This result stems from the interplay between two counteracting forces. On one hand, an increase in the commission rate decreases a seller’s return to providing disclosure, and hence, discourages sellers from making the effort to disclose. On the other hand, a higher commission rate increases the platform’s stake in the sellers’ profits and, as a result, softens competition, increases sellers’ prices and profits, and thus makes it more worthwhile for sellers to provide disclosure of their goods.
An interesting implication of this result is that for a high DCO, the optimal commission rate for a platform should be as small as possible (just enough for the platform to cover the operational cost).
Optimal Precision
Next, I show that a lower precision (i.e., ability of the recommendation system to pin down buyers’ tastes) weakens the effect of the presence of a recommendation system on competition. This happens since more imprecise recommendations effectively increase the share of “undecisive” customers and, thereby, the appeal to capture that market share. As a result, the competition for those customers will intensify.
Imprecision also affects the amount of product information sellers choose to disclose in equilibrium. However, the direction of this effect depends on the cost of disclosure: if the cost is low, a more precise recommendation system may increase the amount of disclosed information, while the result is reversed if the cost is high. The reason for that is as follows: The platform has two sources of information to infer whether a particular seller fits a certain buyer – the buyer’s preferences and the seller’s information on the quality of the product (if disclosed). If the buyer’s taste is measured imprecisely, while the seller’s information is more precise, it is optimal for the platform to focus on the latter when designing a recommendation system. This, in turn, would motivate sellers to disclose more information about their products. In the case of low disclosure costs, this positive effect on disclosure more than offsets the direct negative effect of imprecision brought about by harsher competition and lower profits. In the case of high costs, the direct effect dominates.
I also show that some imprecision, in fact, can be optimal for the platform. Perfect precision softens the competition and results in increased prices for consumers. This negative effect on consumer satisfaction outweighs the benefits of a perfect match between seller and buyer. So, consumers prefer a certain degree of imprecision over perfect precision, which in turn, makes the platform unwilling to implement perfect precision. In other words, it is optimal to “sacrifice” some customers (i.e., not recommending them the best fitting alternative) in order to intensify the competition among sellers and, eventually, benefit all customers through lower prices.
Conclusion
The presence of a recommendation system on an e-commerce platform that charges sellers commissions on sales may cause softer competition and lead to higher prices and profits of sellers, as well as increased earnings for the platform. At the same time, it can sometimes be optimal for a platform to set a low commission rate since it would guarantee that sellers disclose more information about their goods which would improve the match between customers’ tastes and the goods they buy. If customer satisfaction is important for a platform, the indirect positive effect on customer satisfaction of a low commission rate, via sellers’ decisions, may outweigh the direct negative effect on the platform’s and sellers’ profits. Similarly, a recommendation system with some degree of imprecision can be beneficial for customers since it does not allow sellers to get local monopoly power. So, increasing the precision in the measurement of customers’ tastes – which seems to be the focus of many ongoing computer science studies devoted to recommendation systems, – may not actually be in the best interest of a platform.
In the modern era of digitalization, the use of e-commerce platforms is on the rise. Moreover, the ongoing COVID-19 pandemic has increased the use of such platforms even further. Understanding the implications of the strategies used by these platforms, such as recommendation systems, on prices, competition, and societal welfare is, thus, a necessary component for developing efficient regulation principles.
References
- Li, J. Chen, and S. Raghunathan. Advertising Role of Recommender Systems in Electronic Marketplaces: A Boon or a Bane for Competing Sellers? 2016.
- Li, J. Chen, and S. Raghunathan. Recommender System Rethink: Implications for an Electronic Marketplace with Competing Manufacturer. Information Systems Research, 29(4):1003–1023, 2018.
- Kremer, Y. Mansour, and M. Perry. Implementing the “wisdom of the crowd”. Journal of Political Economy, 122(5):988–1012, 2014.
- D. Fedchenko. “Optimal recommendation system for e-commerce: theoretical insights”, 2020, mimeo
Disclaimer: Opinions expressed in policy briefs and other publications are those of the authors; they do not necessarily reflect those of the FREE Network and its research institutes.