Tag: Productivity
Personality Traits, Remote Work and Productivity
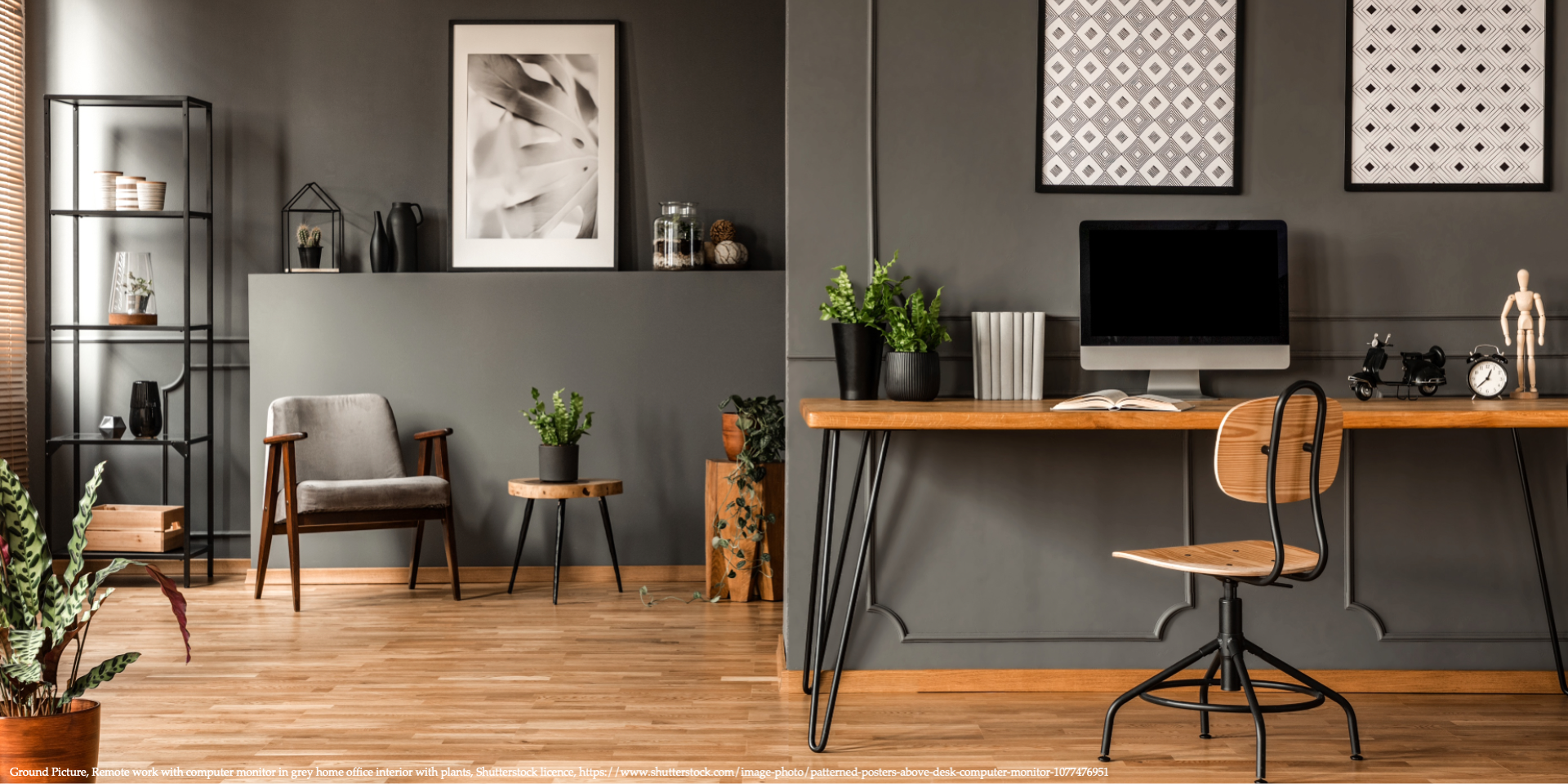
The Covid-19 pandemic generated a massive and sudden shift towards teleworking. Survey evidence suggests that remote work will stick in the post-pandemic period. The effects of remote work on workers’ productivity are however not well understood, some workers gaining in productivity whereas others experience the opposite. How can this large heterogeneity in workers productivity following the switch to teleworking be explained? In this brief, we discuss the importance of personality traits. We document strong links between personality, productivity, and willingness to work from home in the post-pandemic period. Our results suggest that a one-size-fits-all policy regarding remote work is unlikely to maximize firms’ productivity.
Introduction
The Covid-19 pandemic triggered a large and sudden exogenous shift towards working from home (WFH). Within a few months in Spring 2020, the share of remote workers increased from 8.2 percent to 35.2 percent in the US (Bick et al., 2020), and from 5 percent to more than 30 percent in the EU (Sostero et al., 2020). Surveys of business leaders suggest that WFH will stick in the post-pandemic period (e.g., Bartik et al., 2020).
The prevalence of teleworking will ultimately depend on its impact on workers’ productivity and well-being. This impact however remains ambiguous, some studies reporting an overall positive impact, some studies a negative one. Overall, the balance of these pros and cons can vary greatly across individuals. The existing literature emphasizes the importance of gender and occupation for workers’ productivity under WFH arrangements, but a large share of this heterogeneity remains unexplained.
In a recent paper (Gavoille and Hazans, 2022) we investigate the link between personality traits and workers’ productivity when working from home. Importance of non-cognitive skills, in particular personality traits, for individual labor market outcomes is well documented in the literature (e.g., Heckman et al., 2006; Heckman and Kautz, 2012). In the context of WFH, soft skills such as conscientiousness or emotional stability, are good candidates for explaining heterogeneity in relative productivity at the individual employee level.
The Latvian context provides an ideal setup for studying the effect of teleworking on productivity. First, Latvia has a large but unexploited potential for teleworking. Dingel and Neiman (2021) estimate that 35 percent of Latvian jobs could be done remotely, which is about the EU average. However, prior to the pandemic only 3 percent of the workforce was working remotely – one of the smallest figures in the EU. Second, the Latvian government declared a state of emergency in March 2020, which introduced compulsory WFH for all private and public sector employees, except for cases where on-site work is indispensable due to the nature of the work. This led to a six-fold increase in the share of remote workers within a couple of months. This stringent policy constitutes a massive exogenous shock in the worker-level adoption of WFH, well suited for studying.
Survey Design
To study the link between personality traits, teleworking, and productivity, we designed an original survey, implemented in May and June 2021 in Latvia. The target population was the set of employees who experienced work from home (only or mostly) during the pandemic. To reach this population, we used various channels: national news portals, social media (Facebook and Twitter) and radio advertisement. More than 2000 respondents participated in the survey, from which we obtained more than 1700 fully completed questionnaires.
Productivity and Remote Work
In addition to the standard individual characteristics such as age and the likes, we first collect information about respondents’ perception of their own relative productivity at the office and at home. More specifically, we ask “Where are you more productive?”. The five possible answers are “In office”, “In office (slightly)”, “No difference”, “At home (slightly)” and “At home” (plus a sixth answer: “Difficult to tell”). Table 1 provides a description of the answers. Roughly one third of the respondents reports a higher productivity at home, another third a higher productivity at the office, and one third do not report much of a difference. This measure of productivity is self-assessed, as it is the case with virtually any “Covid-19-era” paper on productivity. Note however that our question is not about absolute productivity as such, but relative productivity of teleworking in comparison with productivity at the office, which is arguably easier to self-assess.
Second, we ask “Talking about the job you worked at mostly remotely, and taking into account all difficulties and advantages, what would you choose post-pandemic: working from home or in office for the same remuneration (if you had the choice)?” The five possible answers are “Only from home”, “Mostly from home”, “Indifferent”, “Mostly in office”, “Only in office” (and a sixth option: “Difficult to tell”). The main aim of this question is to study who would like to keep working remotely in the post-pandemic period, irrespective of productivity concerns. Notably, the answers are much different than from the productivity question (see Table 1), which suggests the latter does not reflect preferences.
Finally, we ask respondents about the post-pandemic monthly wage premium required by the respondent to accept i) working at the office for individuals preferring to work from home; ii) working from home for individuals preferring to work at the office. Median values of these premia for workers with different preferences are reported in Table 1 (panel C). These values appear to be economically meaningful both in absolute terms and relative to the median net monthly wage in Latvia (which was 740 euro in 2021), reinforcing the reliability of the survey.
Table 1. Outcome variables
Source: reproduced from Gavoille and Hazans (2022).
Measuring Personality Traits
The survey contains a section aiming at evaluating the personality of the respondent through the lens of the so-called Five Factor Model of Personality. The psychometrics literature offers several standardized questionnaires allowing to build a measure for each of these five factors – Openness to Experience, Agreeableness, Extraversion, Emotional Stability and Conscientiousness. We rely on the Ten-Item-Personality-Inventory (TIPI) measure (Gosling et al., 2003). This test is composed by only ten questions, making it convenient for surveys, and it has been widely used, including in economics. As simple as this approach seems, the performance of this test has been shown to be only slightly below those with more sophisticated questionnaires, and to provide measures highly correlated with the existing alternative measures of personality traits.
Results
Overall, the results indicate that personality traits do matter for productivity at home vs. at the office. The personality trait most strongly related to all three outcome variables is Conscientiousness. Controlling for a battery of other factors, individuals with a higher level of conscientiousness are reporting a higher productivity when working from home as well as a higher willingness to keep working from home after the pandemic. This link is not only statistically significant but also economically meaningful: an individual with a level of conscientiousness in the 75th percentile is 8.4 percentage points more likely to report a higher productivity from home than a similar individual in the 25th percentile. Considering that the sample average is 31 percent, this difference is substantial.
Previous studies documented a positive correlation between Conscientiousness and key labor market outcomes such as wage, employment status and supervisor evaluation. A usual concern of employers is a possible negative selection of workers in teleworking. Observing that highly conscientious workers are more willing to work from home, where they are more productive, suggests that firms do not need to exert a very strict control on employees choosing to telework.
Openness to Experience shows a similar positive relationship with productivity. Extraversion on the other hand is only weakly negatively related to productivity. The relationship between this trait and willingness to work from home is however much stronger. These findings are intuitive: workers with a high Openness to Experience are more likely to cope easily with the important changes associated with switching to WFH. On the other hand, extravert individuals may find it more difficult to remain physically isolated from colleagues.
The literature studying the relationship between WFH and productivity suggests a conditional effect based on gender. In parallel, the literature investigating the role of personality traits on labor market outcomes also documents gender-specific patterns. As our work builds on these two strands of literature, we provide a heterogeneity analysis of the personality traits/productivity relationship conditional on gender.
When disaggregating the analysis by gender, it appears that the relationship between personality traits and productivity is stronger for women than for men. Conscientiousness and (to a smaller extent) Openness to Experience have a strong positive relationship with relative productivity of teleworking for women, while Extraversion and Agreeableness feature economically meaningful negative relationships. Noteworthy, the effects of Agreeableness and Openness to Experience do not concern the probability to be more productive at the office but only the willingness to work from home after the pandemic. For men, only Conscientiousness is significant, with a much smaller magnitude than for women.
Conclusion
We document that personality traits matter for changes in productivity when switching to a WFH regime. In particular, individuals with high levels of Conscientiousness are much more likely to report a better productivity from home than from the office. Additionally, Openness to Experience and Extraversion also do play a role.
Taken together, these results suggest that a one-size-fits-all policy is unlikely to maximize neither firms’ productivity nor workers’ satisfaction. It also highlights that when estimating firm-level ability in switching to remote work, characteristics of individual workers should be considered. In particular, employers practicing remote work should invest in socialization measures to compensate the negative effect of teleworking on the wellbeing of more extravert workers. Finally, several surveys (e.g., Barrero et al., 2021) document that more than a third of workers in the US would start looking for a new job allowing (some) work from home if their current employer would impose a strict in-office policy. Our results support this finding but also indicate that the opposite also holds: some workers would strongly oppose to remaining in a WFH setup after the pandemic. Personality traits are important determinants of the value attached to working from home.
Acknowledgement
This research is funded by Iceland, Liechtenstein and Norway through the EEA Grants. Project Title: The Economic Integration of the Nordic-Baltic Region through Labour, Innovation, Investments and Trade (LIFT). Project contract with the Research Council of Lithuania (LMTLT) No is S-BMT-21-7 (LT08-2-LMT-K-01-070).
References
- Barrero, J. M., Bloom, N. and Steven, D. (2021). Why working from home will stick, NBER Working Paper 28731.
- Bartik, A., Cullen, Z., Glaeser, E., Luca, M. and Stanton, C. (2020). What jobs are being done at home during the COVID-19 crisis? Evidence from firm-level surveys, NBER Working Paper 27422.
- Bick, A. and Blandin, A. (2021). Real-time labor market estimates during the 2020 coronavirus outbreak.
- Dingel, J. and Neiman, B. (2021). How many jobs can be done at home?, Journal of Public Economics, 189, 104235.
- Gavoille, N. and Hazans, M. (2022). Personality traits, remote work and productivity, IZA Discussion Paper 15486.
- Gosling, S., Rentfrow, P. and Swann, W. (2003). A very brief measure of the Big-Five personality domains. Journal of Research in personality, 37(6), pp. 504-528.
- Heckman, J., Stixrud, J. and Urzua, S. (2006). The effects of cognitive and noncognitive abilities on labor market outcomes and social behavior, Journal of Labor economics, 24(3), pp. 411-482.
- Heckman, J. and Tim Kautz. (2012). Hard evidence on soft skills. Labour Economics, 19(4), pp. 451-464.
- Sostero, M., Milasi, S., Hurley, J., Fernandez-Macias, H. and Bisello, M. (2020). Teleworkability and the COVID-19 crisis: a new digital divide?, JRC Working Papers Series on Labour, Education and Technology, No. 2020/05.
Disclaimer: Opinions expressed in policy briefs and other publications are those of the authors; they do not necessarily reflect those of the FREE Network and its research institutes.
Remaining Challenges for Faster Growth in CESEE
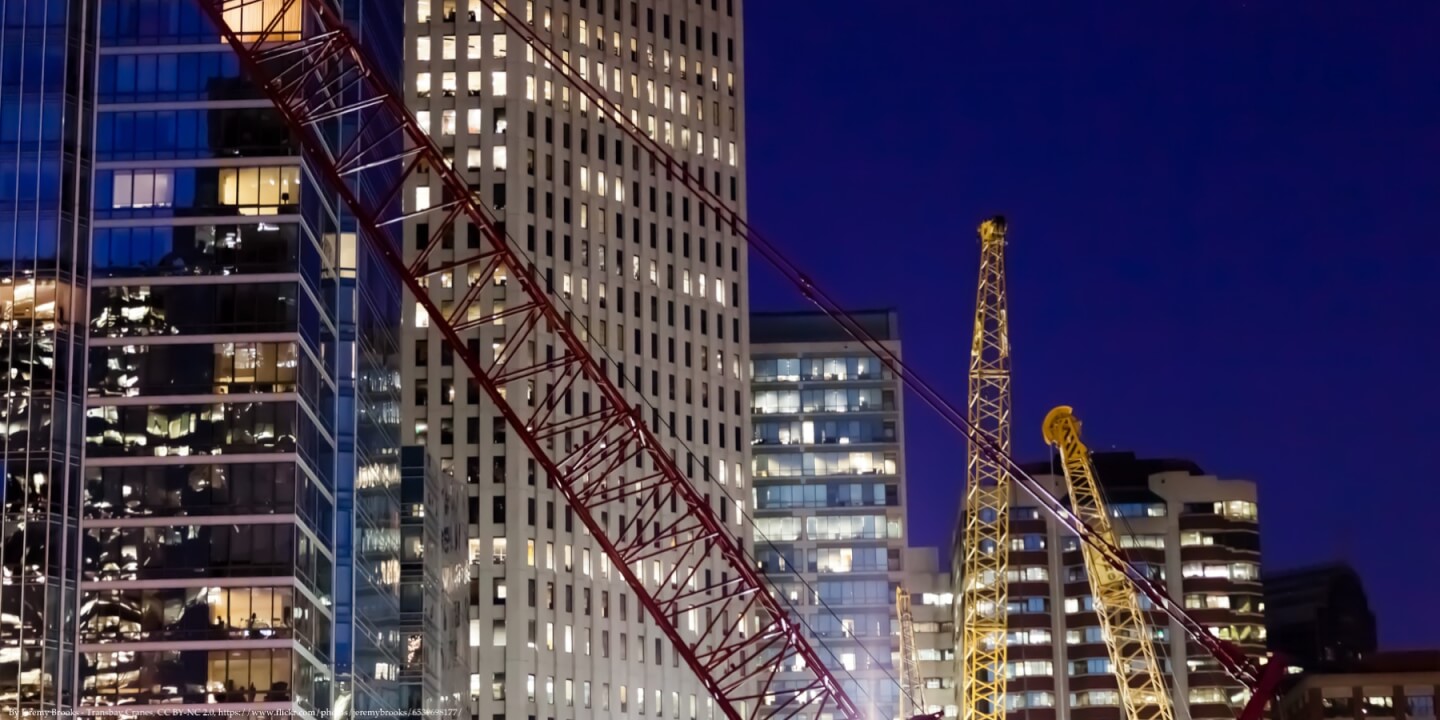
Between 1995 and 2016, per capita GDP levels in Europe have converged, as countries that had lower income levels in 1995 on average have seen faster growth rates between 1995 and 2016 (Figure 1).
Figure 1
Income differentials between CESEE and Germany have narrowed significantly during this time. If we look at CESEE as a whole, in 1995 GDP per capita of CESEE was only a third of Germany. By 2016 it has increased to almost half. If we look at individual countries, all countries in CESEE have seen faster GDP growth than in Germany, but there have been important cross-country differences. For example, growth has been relatively rapid in the EU New Member States and very slow in Ukraine.
Nevertheless, CESEE is still much poorer than Germany. The richest country in CESEE – Slovenia – has the income level per capita Germany had in 1990 (Figure 2). Poland is as rich as Germany was in the late 1970s. And Ukraine, which in early transition had similar level of income to Poland, is now as rich as Germany was in the early 1950s.
Figure 2
CESEE is poorer both because labor productivity is lower and a smaller share of the population works. GDP per capita is the product of GDP per worker and the employment to population rate:
In 2015, labor productivity in CESEE was still well below that in Germany and the Netherlands (Figure 3, x-axis). Employment rates were also lower, but those differences were less pronounced (Figure 3, y-axis).
Figure 3
Differences in employment rates are, however, more pronounced if we take into account that in CESEE a higher share of the population is of working age. The employment to population rate is the product of the employment to working age population [1] rate:
The share of the working age population in CESEE is relatively high (Figure 4), although it is now declining. The employment to working age ratios in CESEE are well below those in Germany (Figure 5); only the Baltics come close.
Figure 4
Figure 5
It will be challenging to further increase the employment to total population rate, given the impact of aging and the already relatively low level of unemployment. The decline of the working age population will accelerate in the next decade (Figure 6) as the baby-boom generation is retiring; in a number of countries the working age population is set to decline by more than 1 percent annually. [2] If the share of the working age population that works remains constant, the share of the employment to total population rate will fall sharply. At the same time, the unemployment rate in many countries is already close to pre-crisis lows (Figure 7). It will therefore be key to increase labor force participation rates, which in most countries are still below those of Germany, particularly those of women (Figure 8).
Figure 6
Figure 7
Figure 8
A higher capital stock may be even more important than raising the employment rate. There is a strong correlation between the level of capital stock per capita and GDP per capita (Figure 9, left panel). The relationship between the employment rate and GDP per capita is much weaker (Figure 9, right panel). Further convergence of CESEE will thus require capital deepening. As of 2015, the capital stock per capita in CESEE region is on average only a quarter of that in Germany.
Figure 9
Figure 10
Figure 11
Figure 12
Unfortunately, the growth of the capital stock per capita has slowed (Figure 10), which reflects the decline in investment rates. Investment rates are low compared with other emerging market countries (Figure 11). Saving rates are low too (Figure 12), which suggests that a rebound of investment could lead to a re-emergence of high current account deficits, unless savings increases as well. Yet it may be challenging to boost saving. With labor markets tightening, wages shares are likely to increase, which is likely to reduce corporate profits. Indeed, in a number of countries this is already happening (Figure 13). Household savings are difficult to influence. Boosting public savings would help, yet even though unemployment rates are falling, few countries plan a meaningful fiscal tightening (Figure 14).
Figure 13
Figure 14
TFP growth has slowed as well. TFP growth has recovered somewhat in recent years, but it is still much slower than in the pre-crisis years (Figure 15). The TFP slowdown might be a result of both the decrease of productivity in main trading partners and unfinished post-crisis adjustment.
The IMF’s CESEE Regional Economic Issues have identified several factors that might restrain productivity and investment. The May 2016 and November 2016 IMF CESEE Regional Economic Issues [3] analyzed several areas where reforms are needed in CESEE, and recommended to improve institutions to boost productivity. The May 2016 REI suggested the largest efficiency gains might come from increasing protection of property rights, upgrading legal systems and other government services. In this context, the November 2016 REI discussed the need to improve public investment management and tax administration. Given the large gaps in infrastructure and capital stock to Western Europe, improving the efficiency of public investment by improving its allocation and the implementation of frameworks and procedures could boost potential growth significantly. Regarding tax administration, reducing compliance gaps, would help improve tax collection, which could generate more fiscal revenues and allow for higher public investment.
Figure 15
In short, further catch-up is possible but challenging. Labor force participation could be further increased, which would also help to offset declining share of working age population. A slowdown or even reversal of net emigration would also contribute. The capital stock is relatively low, and higher investment is needed especially in infrastructure, but raising the saving rate will be a challenge. Since the crisis the TFP has slowed considerably, and re-igniting TFP growth will be crucial for boosting growth. For all this, improving the quality of institutions and legal frameworks will help.
Bas Bakker is the IMF’s Senior Resident Representative for Central and Eastern Europe; Marta Korczak and Krzysztof Krogulski are economists in the IMF’s regional office for Central and Eastern Europe in Warsaw. The views expressed in this paper are those of the authors and do not necessarily represent those of the IMF or IMF policy. Comments by [Jorg Decressin] on an earlier version are gratefully acknowledged.
[1] The working age population is the population ages between 15 and 64.
[2] In many countries, demographics pressures have been exacerbated by the net emigration. A reduction in emigration, or even reversal, would also help. See IMF Staff Discussion Note “Emigration and Its Economic Impact on Eastern Europe” available at https://www.imf.org/external/pubs/ft/sdn/2016/sdn1607.pdf
[3] In many countries, demographics pressures have been exacerbated by the net emigration. A reduction in emigration, or even reversal, would also help. See IMF Staff Discussion Note “Emigration and Its Economic Impact on Eastern Europe” available at https://www.imf.org/external/pubs/ft/sdn/2016/sdn1607.pdf
On Economics of Innovation Subsidies in Russia

Following the general agreement that innovation is a source of economic growth, the Russian government has provided various stimuli to foster domestic innovation. One of the mechanisms of innovation policy is research subsidies. This policy brief starts off with a discussion of the theoretical predictions and empirical evidence, which relates the economic incentives of research subsides to innovation and growth. We then address the potential adverse effects of focusing innovation subsidies mainly on large public companies in Russia. Finally, we attempt to establish a link between the innovation rate and market competition within Russian industries.
Overview
According to data from the Russian Statistical Agency, the R&D intensity – measured by R&D expenditure as percent of sales – increases with company size. Companies with 50 to 500 employees spend 1% of their sales on R&D, while the R&D intensity varies from 2 to 5% of sales for larger businesses (see Figure 1). The size non-neutrality of R&D in Russia contradicts the findings in the theoretical and empirical literature, which hold for companies in the developed countries (Cohen, 2010). An explanation may be the excessive government support to public companies in Russia, and in particular, to larger public corporations. A positive consequence of such policies is that public corporations come ahead of private companies, not only in R&D intensity, but also in innovation rates (see Figures 2–3).
However, government support towards innovation does not necessarily have a positive impact on overall economic activity. The purpose of this brief is to discuss the unwanted effects of the government policy in the form of research subsidies, both in theory and in an application to public companies and corporations in Russia. We base our analysis on the outcomes of the 2014–2017 micro surveys by the Analytical Center under the Government of the Russian Federation.
The role of government
Fighting under-provision of innovation
According to the seminal paradigm of the endogenous growth models with technological change, companies are engaged in quality competition, and their innovations are explained by a rational decision to raise profits through expanding the markets for existing products or entering markets for new products (Schumpeter, 1942; Romer, 1990; Grossman and Helpman, 1991; Kletter and Kortum, 2004). The innovation becomes one of the causes of economic growth, which is proved in empirical applications for developed countries, such as the U.S., Japan and the Netherlands (Akcigit and Kerr, 2010; Lentz and Mortensen, 2008; Grossman, 1990).
Figure 1. Innovation rate and R&D intensity by company size (number of employees)
Source: Indicators of Innovation in the Russian Federation: 2017. Tables 2.4, 2.16, Data for 2015. Innovative rate is % of companies involved in innovative activity.
However, the technological change is closely linked to knowledge disclosure, which means that new products become vulnerable to imitation, and that the non-rival character of knowledge causes an under-provision of innovation on the market (Arrow, 1962). The argument supports the cause for government policies through the system of intellectual property rights on the legal side, and research subsidies as an economic mechanism (Rockett, 2010; Hall and Lerner, 2010). Research subsidies are expected to have a positive effect on innovation rate, as is empirically shown for the U.S. in Acemoglu et al. (2016) and Wilson (2009). However, the impact on economic growth is ambiguous (Acemoglu et al., 2013; Grossman, 1990).
Figure 2. Innovation rate and R&D intensity by ownership
Source: Indicators of Innovation in the Russian Federation: 2017. Tables 2.6, 2.17, Data for 2015, public corporations are different from organizations by regional/federal government.
Figure 3. Share of public funds in R&D financing, % of company budget
Notes: Indicators of Innovation in the Russian Federation: 2017. Table 1.13; Innovation Development Programmes of Russian State-Owned Companies, Fig.4.
Unwanted effects of subsidies
Two concerns are associated with subsidization of innovation. First, while research subsidies may stimulate innovation among the targeted companies, the growth effect is likely to be heterogeneous across companies in the industry or economy, leading to a neutral or even negative overall effect. For instance, the increased innovation rate in subsidized large incumbents may curb entry of new (and more productive) firms, so the net outcome is deceleration of growth in the economy (Acemoglu et al., 2013). Research subsidies may even cause a shrinking of the high-tech sectors: if skilled labor moves from manufacturing to research labs, manufacturing may experience a shortage of labor, resulting in the net effect being a decrease in production (Grossman, 1990).
Another extreme of subsidizing entrants, in view of antitrust policies, occurs when former entrants change their market status to incumbents: now they face lower profits relative to newer entrants and hence, become less incentivized in their economic activity (Segal and Whinston, 2007).
Second, innovation policy (for instance, in the form of subsidies) may sometimes not even increase the innovation rate. Indeed, incumbents have no incentives to innovate in order to keep their market power or to prevent entry of higher quality firms in industries with non-perfect competition (Rockett, 2010; Qian, 2007).
Both mechanisms are likely to hold for Russian industries, where the protection of large public corporations has led to low competition, various forms of distortions on the market and hence, weak incentives to innovate.
Potential adverse effects in Russia
Large companies are likely to attract public attention owing to their obvious advantages in spreading fixed costs of innovations (Cohen,
2010). Russia is no exception to the phenomenon, so public corporations, which are commonly of a large size, received government subsidies. However, the subsidy is primarily used for acquiring new technologies and perfecting design, rather than conducting R&D (See Figure 4 with comparison available for communications and IT industry). The fact points to a possibility of a small effect of innovations on growth of public companies. Only if the research subsidy is spent on delegating the R&D research to specialized firms, with a subsequent acquiring of the resulting technology, the existing policy of supporting public corporations may induce their growth and/or growth of the corresponding industry.
Figure 4. Structure of spending the research subsidy in communications and IT in 2013, %
Notes: Indicators of Innovation in the Russian Federation: 2017. Table 1.134 Innovation Development Programmes of Russian State-Owned Companies, Fig.3.
In an attempt to formally assess the effect of innovation subsidies on company growth, we focus on the time profiles of the common proxies for company size: sales, profits and employment (Akcigit et al., 2017; Akcigit and Kerr, 2010; Acemoglu et al., 2013). The macroeconomic literature predicts that innovation becomes one of the channels for an increase of each of the three variables through a rise in quality. Motivated by this literature, the micro-data analysis “On the Interaction of the Elements of the Innovation Infrastructure”, conducted by the Analytical Center under the Government of the Russian Federation (2014), asked companies to assess their changes in sales, profits and employment in response to the innovation subsidy. As a result, the outcomes of the above analysis allow for a comparative assessment of the impact of the government’s innovation subsidy for public and private companies.
In particular, the results point to higher growth across private companies owing to research subsidies: the percent of private companies with new employees is higher than that of public companies. Similarly, the percentage of private companies that increased market share or raised profits/export due to subsidies exceed those of the public companies (see Figure 5). Here, we interpret new hires as employment growth and increase of market share as a potential indicator of sales growth.
Figure 5. Economic activity owing to research subsidies, % of companies
Source: Analytical Center under the Government of the Russian Federation, 2014. Fig.22
The innovation activity in private Russian companies lead to a higher prevalence of new products in comparison with public companies. The fact goes in line with a more important role of research and development in the innovative activity of private Russian companies (see Figure 4).
Finally, we attempt to establish a link between the innovation rate and market competition at the level of Russian industries. For this purpose, we use the results of the annual surveys “An assessment of the competitiveness in Russia”, conducted in 2015–2017 by the Analytical Center across 650–1500 companies from 84 Russian regions. The respondents were asked if they implemented R&D as a strategy for raising their competitiveness. We use the percentage of firms doing R&D as a proxy for the innovation rate. Competition in the industry was evaluated by respondents on a five-point scale (no competition, weak, median, high and very high), and we combine the prevalence of the two top categories as a proxy for competition in the industry.
Figure 6. Competition and R&D in Russian industries, % of firms
Source: Analytical Center under the Government of the Russian Federation, 2017, pp.8, 18.
The results show that innovative activity in the form of R&D or product modification is observed in industries with relatively high competition in Russia – for instance, in machinery and electric/electronic equipment (Figure 6). At the same time, industries where competition is not as high (e.g. woodworking, construction) show absence of either type of innovation. The findings go in line with the economic theory about market competition being a prerequisite for the rational choice of companies about innovation. Moreover, if the purpose of government subsidies is to foster innovation, the effective allocation of subsidies would imply the focus on Russian industries with high competition – here various forms of innovation do play a role in the company strategy on the market.
Conclusion
Our analysis outlines the theoretical foundations for the potential adverse effects of innovation policies in the form of research subsidies. The unwanted outcomes may relate to heterogeneity of companies and absence of the association between innovation activity and growth on non-competitive markets.
We offer the empirical evidence, which points to the undesired effects of subsidizing public companies in Russia. For instance, compared to the overall Russian sector of communications and IT, the innovative activity in public corporations has a weaker association with research and development. Additionally, compared to private companies, the innovations may result in smaller prevalence of increased exports, profits or new hires, as well as in a less frequent development of new products by public companies in Russia.
References
- Acemoglu, D., Akcigit, U., Bloom, N., Kerr, W. R., 2013. “Innovation, reallocation and growth”, National Bureau of Economic Research Working paper, No. 18993.
- Acemoglu, D., Akcigit, U., Hanley, D., Kerr, W. (2016). Transition to clean technology. Journal of Political Economy, Volume 124(1), pages 52-104.
- Akcigit, U., Kerr, W. R., 2010. “Growth through heterogeneous innovations” National Bureau of Economic Research Working Paper, No. 16443.
- Analytical Center under the Government of the Russian Federation, 2014. “On the Interaction of the Elements of the Innovation Infrastructure”, Analytical report, in Russian.
- Analytical Center under the Government of the Russian Federation, 2015-2017. “An Assessment of the Competitiveness in Russia”, Analytical reports, in Russian.
- Arrow, K., 1962. “Economic welfare and the allocation of resources for invention”, In The Rate and Direction of Inventive Activity: Economic and Ssocial Factors, Princeton University Press, pages 609-626.
- Cohen, W. M., 2010. “Fifty years of empirical studies of innovative activity and performance”, Handbook of the Economics of Innovation, Volume 1, pages 129-213.
- Grossman, G. M., Helpman, E., 1991. “Quality ladders in the theory of growth”, The Review of Economic Studies, Volume 58(1), pages 43-61.
- Grossman, G.M., 1990. ”Explaining Japan’s innovation and trade”, BOJ Monetary and Economic Studies, Volume 8(2), pages 75-100.
- Hall, B. H., Lerner, J., 2010. “The financing of R&D and innovation”, Handbook of the Economics of Innovation, Volume 1, pages 609-639.
- Indicators of Innovation in the Russian Federation: 2017. N. Gorodnikova, L. Gokhberg, K. Ditkovskiy et al.; National Research University Higher School of Economics, in Russian.
- Innovation Development Programmes of Russian State-Owned Companies: Interim Results and Priorities, 2015. M. Gershman, T. Zinina, M. Romanov et al.; L. Gokhberg, A. Klepach, P. Rudnik et al. (eds.), National Research University Higher School of Economics, in Russian.
- Klette, T. J., Kortum, S., 2004. “Innovating firms and aggregate innovation”, Journal of Political Economy, Volume 112(5), pages 986-1018.
- Lentz, R., Mortensen, D.T., 2008. “An empirical model of growth through product innovation”, Econometrica, Volume 76(6), pages 1317–1373.
- Qian, Y., 2007. “Do national patent laws stimulate domestic innovation in a global patenting environment? A cross-country analysis of pharmaceutical patent protection, 1978–2002”, The Review of Economics and Statistics, Volume 89(3), pages 436-453.
- Rockett, K., 2010. “Property rights and invention”, Handbook of the Economics of Innovation, Volume 1, pages 315-380.
- Romer, P. M. (1990). Endogenous technological change. Journal of political Economy, 98(5, Part 2), S71-S102.
- Segal, I., Whinston, M.D., 2007. “Antitrust in innovative industries”, American Economic Review, Volume 97(5), pages 1703-1730.
- Schumpeter, J., 1942. “Creative destruction”, Capitalism, Socialism and Democracy, pages 82-83.
- Wilson, D. J., 2009. Beggar thy neighbor? The in-state, out-of-state, and aggregate effects of R&D tax credits. The Review of Economics and Statistics, Volume 91(2), pages 431-436.
Higher Competition in the Domestic Market – A Way to Boost Aggregate Productivity

Competition is a good thing not only because of lower prices and larger variety. Higher competition in the domestic market also shifts necessary labour and capital resources from less productive domestic-oriented firms to export-oriented productivity champions. Such firms will make better use of production factors and generate larger output. Thus, simply increasing the level of competition in the domestic market can boost the aggregate productivity of a country.
The aggregate productivity of a country can be boosted even without changing the productivity of individual enterprises. This can be achieved by improving the allocation of resources – the redistribution of labour and capital towards more productive firms. These firms will make better use of production factors and generate larger output. But how can one affect the allocation of resources? Economic theory says that allocation depends on the productivity of individual firms: more productive enterprises attract more labour and capital. However, there exists another factor behind allocation: distortions.
Distortions affect the allocation of resources
A model developed by Hsieh and Klenow (2009) – one of the most popular frameworks to study the allocation of resources – has a very important and realistic feature: it acknowledges that firms are not treated equally. Some firms may face lower supply of banking loans ending with higher capital costs. Other firms could confront with trade unions and higher wages. Tax rates may also differ across firms. These are all examples of distortions. Firms facing larger distortions are forced to underuse respective production factor, while firms that enjoy more favourable conditions tend to overuse capital and labour, generating more output.
While it is virtually impossible to imagine an economy without any distortions (the one where all firms face the same taxes, costs of labour, capital etc.), not all distortions damage the allocation of resources. Only distortions to productive firms create misallocation of resources by shifting labour and capital towards unproductive firms. Thus, removal of such distortions can improve the efficiency of allocation and raise the aggregate output of the country.
According to Hsieh and Klenow (2009) the distortions faced by every individual firm can be quantified from the balance sheets and profit/loss data. For example, observing lower-than-usual ratio of capital to intermediate inputs (comparing with other enterprises in a narrowly defined industry) indicates a capital distortion, possibly related with limited access to banking loans. Similarly, lower-than-usual share of wages in total production costs implies high labour distortions. Finally, the size of the distortion can be detected as a case of abnormally low share of intermediate inputs in total output, and signals about the restrictions to total output (e.g. due to higher taxes for large enterprises).
Misallocation of resources is small in Latvia
In my recent research (see Benkovskis, 2015), I use anonymised firm-level dataset for 2007–2013 and apply the Hsieh and Klenow (2009) model to study the allocation of resources in Latvia – a unique example of a small and open economy facing extreme structural shifts during the financial crisis. According to my estimates, the negative contribution of misallocation to aggregate productivity was close to 27% in 2013 (see Figure 1). In other words, it suggests that actual aggregate productivity could be boosted by 27% if all distortions were removed!
This may seem large but in fact 27% is a comparatively low figure. Hsieh and Klenow (2009) argue that full liberalisation would boost aggregate manufacturing productivity by 86–115% in China, 100–128% in India, and 30–43% in the US. Dias et al. (2015) show that removing distortions would lead to a 30% gain in output of Portugal in 2011. Thus, misallocation of resources is relatively small in Latvia. Even more important: the misallocation of resources decreased after the crisis in Latvia (contrary to the case of Portugal), adding more than 10 percentage points to aggregate productivity growth between 2010 and 2013.
Figure 1. Contribution from misallocation of resources to aggregate total factor productivity, %
Source: Benkovskis (2015). Note: shows the contribution of misallocation comparing with the counterfactual case of no distortions.
The finding that allocation of resources improved after the crisis is interesting per se, but uncovering the reasons behind the improvement is even more important. Figure 1 provides a decomposition, which shows that labour distortions are minor in Latvia due to high flexibility of labour market (in line with recent findings by Braukša and Fadejeva, 2016). The capital distortions, while being minor in 2007–2008, increased afterwards, pointing to some credit supply constraints faced by the highly productive enterprises after the financial crisis. However, by far largest contribution comes from the misallocation of intermediate inputs – the turnover of the most productive firms face some constraints. And it was the ease of constraints to turnover for the most productive firms that determined the improvements in aggregate productivity since 2010.
The level of competition matters for misallocation
My research stresses the importance of the competition level on the market, since higher competition serves as a natural constraint for the firm to increase its turnover. What if the most productive Latvia’s firms systematically come up against higher competition? I found that indeed this is the case. First, recent results by Fadejeva and Krasnopjorovs (2015) show that Latvia’s domestic market has lower competition level comparing with external markets. Second, it is widely acknowledged that exporters tend to be more productive comparing with domestically oriented firms (see e.g. Bertou et al., 2015, who report positive export premiums for EU countries, while Benkovskis and Tkačevs, 2015, find higher productivity of exporters in Latvia). Thus, Latvia’s productive export-oriented firms are subject to higher competition and cannot enlarge their turnover as easy as other entities. This shifts labour and capital towards small and less productive firms working solely on domestic market, creating the misallocation of resources.
The domestic competition factor can also explain the improving allocation of resources after 2010. The study by Fadejeva and Krasnopojorovs (2015) reveals that the competition gap between domestic and foreign markets narrowed after the financial crisis (see Table 1). Namely, life was too easy on the local Latvia’s market during the boom time, allowing unproductive firms to survive and drain away resources from more productive firms. But conditions became tougher after the crisis (although the competition level still remained lower than abroad). We can view this as a “cleansing effect of the crisis”: some of the least productive domestic oriented firms went bankrupt (or decreased their turnover), freeing the necessary capital and labour resources for productive exporters.
Table 1: Change in the competitive pressure on main product in domestic and foreign markets compared to the situation before 2008, %
Domestic market | Foreign market | |||
2008–2009 | 2010–2013 | 2008–2009 | 2010–2013 | |
Strong decrease | 2.9 | 2.2 | 0.9 | 1.0 |
Moderate decrease | 11.8 | 3.8 | 7.6 | 5.9 |
Unchanged | 33.8 | 24.7 | 45.7 | 51.5 |
Moderate increase | 30.0 | 28.1 | 25.2 | 19.7 |
Strong increase | 18.7 | 38.5 | 11.2 | 8.8 |
Does not apply | 2.8 | 2.8 | 9.4 | 13.1 |
Source: Fadejeva and Krasnopjorovs (2015), Table A.102. Notes: based on the sample of 557 Latvia’s firms; results are weighted to represent firm population.
Conclusion
This research has an important policy conclusions applicable to any country that seeks to increase aggregate productivity. The competition level in the domestic market is important not only for consumers, who enjoy lower prices and higher variety. Higher competition in the domestic market also shifts necessary resources from less productive domestic-oriented firms to export-oriented productivity champions.
References
- Benkovskis, Konstantins; 2015. “Misallocation of resources in Latvia: did anything change during the crisis?”, Latvijas Banka Working Paper No.5/2015.
- Benkovskis, Konstantins; and Olegs Tkacevs, 2015. “Everything you always wanted to know about Latvia’s service exporters (but were afraid to ask)”, Latvijas Banka Working Paper No.6/2015.
- Berthou, Antoine; Emmanuel Dhyne; Matteo Bugamelli; Ana-Maria Cazacu; Calin-Vlad Demian; Peter Harasztosi; Tibor Lalinsky; Jaanika Meriküll ; Filippo Oropallo; and Ana Cristina Soares, 2015. “Assessing European Firms’ Exports and Productivity Distributions: The CompNet Trade Module”, ECB Working Paper, No. 1788.
- Braukša, Ieva; and Ludmila Fadejeva, 2016. “Internal labour market mobility in 2005–2014 in Latvia: the micro data approach”, Baltic Journal of Economics, 16(2), 152–174.
- Dias, Daniel A.; Carlos Robalo Marques; and Christine Richmond, 2015. “Misallocation and Productivity in the Lead Up to the Eurozone Crisis“, International Finance Discussion Papers 1146.
- Fadejeva, Ludmila; and Olegs Krasnopjorovs, 2015. “Labour Market Adjustment during 2008–2013 in Latvia: Firm Level Evidence”, Latvijas Banka Working Paper, No. 2/2015.
- Hsieh, Chang-Tai; and Peter J. Klenow, 2009. “Misallocation and manufacturing TFP in China and India“, The Quarterly Journal of Economics, 124(4), 1403–1448.
The Anatomy of Recession in Belarus

After impressive growth in the 2000s, Belarus’ economy has since the currency crisis of 2011 stalled. Structural issues – dominance of the state sector and directed lending practices – have made growth anemic. Recession for Belarus’ main trading partner and the decline of oil prices has aggravated the long-run problems. We perform growth diagnostics to separate the effects of total factor productivity (TFP) growth from capital accumulation over the recession. We show that, as in the 2000s, capital accumulation had the largest positive effect on growth in Belarus, but TFP gains were very low, or even negative in the years of recession.
During the 2000s, Belarus experienced extraordinarily high growth rates, despite a lack of economic reforms and low performance in the EBRD transition indicators. In Kruk and Bornukova (2014) we show that the growth was extensive in its nature, and mainly driven by capital accumulation. The total factor productivity (TFP) contribution to growth was low. After the currency crisis of 2011 in Belarus, however, growth rates have stagnated. Despite a high investment rate (which declined dramatically only after 2015) the growth rates were below 2 per cent per annum, which is a non-satisfactory performance for a developing economy (see Figure 1). In 2015, Belarus entered its first recession in the last 20 years with GDP declining by 3.9 per cent, and the recession has continued in 2016.
Figure 1. GDP Growth Rates and Investment Rates in Belarus (%), 2005-2015.
In the 2000s, the Belarusian government relied on directed-lending programs, and subsidized the interest rates for state-owned enterprises’ (SOE) loans. After the currency crisis of 2011, which many blamed on the loose monetary policies connected to directed-lending programs, the government switched to a so-called modernization policy that underlined the need to invest in new equipment and introduce new technologies. So far this policy have not bear fruits in terms of economic growth, but did it increase efficiency?
Growth Decomposition 2011-2015
Using the standard capital services approach modified for the Belarusian data in Kruk and Bornukova (2014), we decompose Belarusian economic growth in 2011-2015 into the growth of factors (capital and labor) and growth of TFP. We find that the lack of growth in TFP explains the lack of GDP growth and GDP decline over these years.
Figure 2. Gross Value Added Growth Decomposition in Belarus, 2006-2015.
Source: Author’s calculations based on Belstat data. Note: K stands for capital, L for labor, TFP for total factor productivity, and CU for capacity utilization.
A noteworthy fact about the Belarusian growth decomposition is that the direction of growth rate of capital and TFP has been persistently opposite in 2012-2015. Presumably, accelerated capital accumulation vs. stagnating/lowering TFP could be explained by initially insufficient levels of it (i.e. less than steady state). However, this explanation seems to be improper for the Belarusian path. According to our assessments, a capital stock has passed its steady state level at the turn of 2013-2014. Despite this, capital kept growing rapidly, while productivity contracted. An alternative explanation – a growth of the capital stock was secured by specific directed instruments; this artificial capital accumulation caused an endogenous contraction of TFP, as confirmed by the data.
Indeed, a TFP decline could accompany capital accumulation due to expanding allocation and technical inefficiencies. This explains the meltdown of economic growth in Belarus by 2013-2014 and its transition to the negative spectrum later on. In late 2014-2015, this was supplemented by exogenous negative shocks affecting TFP – deteriorating terms of trade and a shrinking energy subsidy from Russia – which caused a rapid dip into recession, which should be classified as structural adjustment.
In 2015-2016, lack of TFP growth and excessive capital accumulation caused further adjustments: firms reduced capital investments radically and contracted capacity utilization. These mechanisms amplified structural recession by a cyclical component.
Sectoral dimension: manufacturing
Out of all the manufacturing industries, only one – manufacturing of electrical, electronic and optical equipment – had positive TFP growth in 2011-2015. On average, manufacturing has lost 4.1% of TFP over this period, with the highest TFP losses in the industries that have always been hallmark for Belarus: manufacturing of machinery (-7.6%) and transport equipment and vehicles (-8.8%). The wood-processing industry has notoriously obtained huge financial aid during the modernization campaign (over 1 billion USD – but Belta (2015) lost 5.6% of TFP over 2011-2015.
We also find that the capital market continues to be distorted by the government interventions, leading to inefficient allocations in the sense that investment is not going to the most efficient industries. On the contrary, there is a negative relationship between the capital growth rate and the TFP growth rate in manufacturing industries. The labor market, which faces less government intervention, functions more efficiently. Labor growth is higher in the industries with higher initial labor productivity.
International comparisons
While comparing the TFPs of Belarusian industries to each other makes little sense (like comparing apples and oranges), comparing them to the TFPs of corresponding industries in other countries might shed some light on the comparative efficiency and competitiveness of the Belarusian economy. Table 1 lists the industries and sectors of the Belarusian economy that are the most and least competitive in a relative TFP sense.
Table 1. TFP winners and losers in Belarus
2014 TFP relative to | ||
Czech Republic | Sweden | |
Winners | ||
Petroleum products | 1.98 | – |
Transport services/communications | 1.67 | 0.70 |
Trade and repair | 1.37 | 1.77 |
Financial activities | 1.33 | – |
Chemicals manufacturing | 1.17 | – |
Losers | ||
Transport vehicles | 0.72 | – |
Machinery and equipment | 0.70 | 0.34 |
Textiles | 0.68 | 0.26 |
Woodworking | 0.56 | – |
Electricity, gas and water | 0.41 | 0.22 |
Agriculture | 0.40 | – |
Source: Author’s calculations.
The majority of the industries in the “winners” category are non-tradable (services like communications, finance, trade and repair). Coincidentally, trade, transport and finance also have relatively high shares of private ownership. Another group of winners are rent industries (petroleum benefitting from cheap Russian oil; and chemical industry built on potassium salts extraction).
As for the most of the manufacturing industries, where the government dominates, and where extensive financing was available at subsidized rates, TFP levels are relatively low. While the TFP performance of the manufacturing of transport vehicles, machinery and other equipment was also reported as low in 2010 (Kruk and Bornukova, 2014), the woodworking industry reached high levels of inefficiency after 2010, when the “modernization” program of this industry received a huge influx of capital.
The relative levels of TFP are good predictors of the future exports performance: higher-TFP industries are more competitive in the international markets. The current low relative TFP of the manufacturing sectors suggests that manufacturing exports will not recover in the coming years.
Conclusion
As in the 2000s, Belarus relies on capital accumulation to generate economic growth. In recent years, however, more investments have not generated growth and rather led to losses in TFP, aggravated by external factors. The current recession in Belarus is mainly a structural adjustment, driven by distortive policies of capital accumulation and allocation; and only partially driven by external shocks.
Lack of TFP growth leads to loss of international competitiveness, causing a collapse of exports. Deep structural reforms are necessary to revive growth and recuperate the lost export potential.
References
- Belta (2015) http://eng.belta.by/president/view/bellesbumprom-group-to-increase-exports-to-1.4-1.5bn-by-late-2017-2860-2014/
- Kruk, Dzmitry; and Kateryna Bornukova, 2014. “Belarusian Economic Growth Decomposition”, BEROC working paper series, WP no. 24
What Does Ukraine’s Orange Revolution Tell Us About the Impact of Political Turnover on Economic Performance?
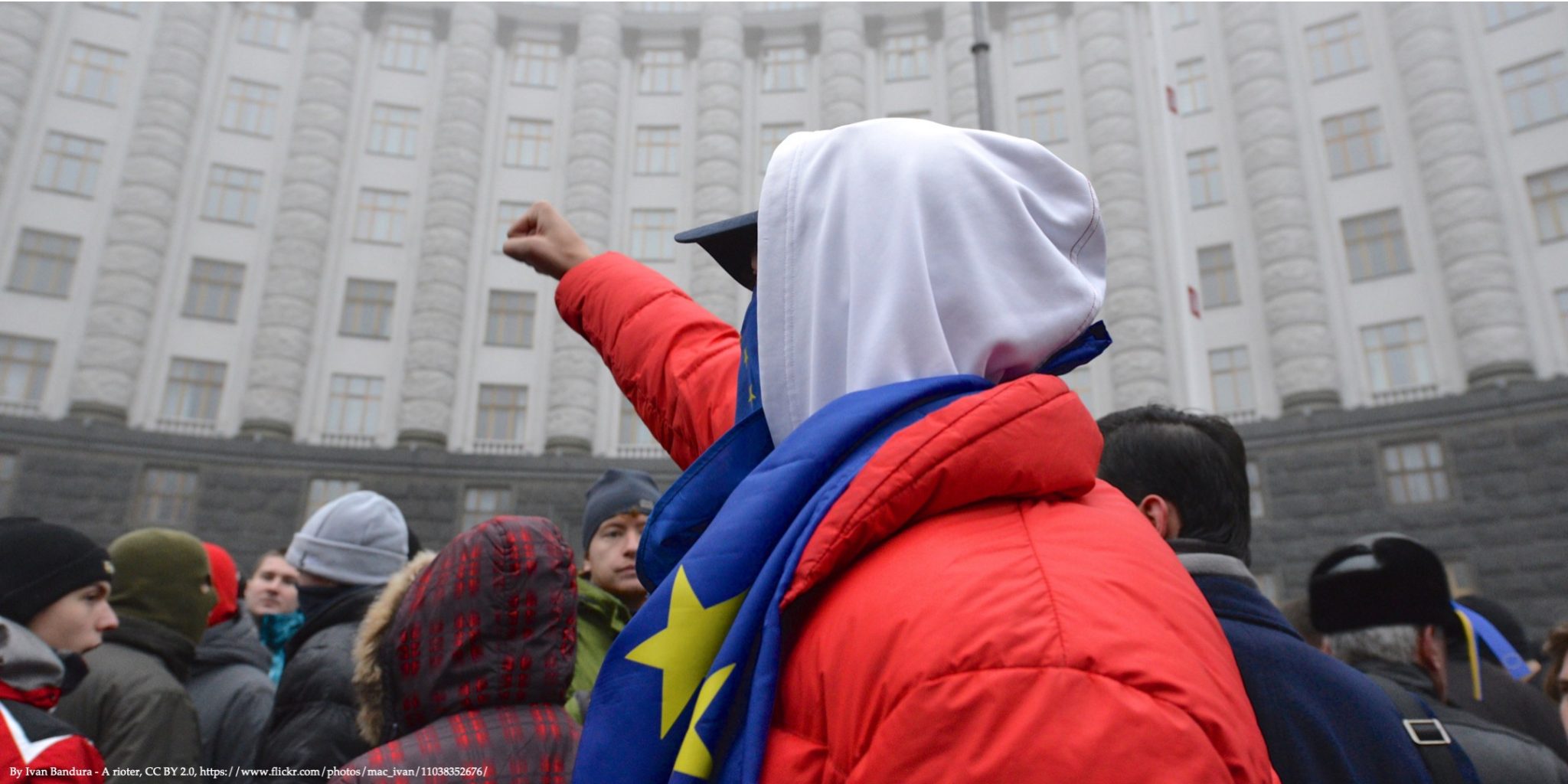
Political turnover is a normal, even desirable, feature of competitive politics, yet turnover in a context of weak institutions can create policy uncertainty, disrupt political connections, and threaten the security of property rights. What is the impact of political turnover on economic performance in such an environment? We examine the behavior of over 7,000 enterprises before and after Ukraine’s Orange Revolution—a moment of largely unanticipated political turnover in a country with profoundly weak institutions. We find that the productivity of firms in regions that supported Viktor Yushchenko increased after the Orange Revolution, relative to that of firms in regions that supported Viktor Yanukovych. Our results illustrate that the efficiency consequences of turnover can be large when institutions are weak.
Introduction
Politics in much of the world is a winner-take-all contest. When Viktor Yanukovych fled Kyiv in February 2014, for example, he was joined by a close group of associates overwhelmingly drawn from the country’s Russian-speaking East, including Yanukovych’s home region of Donetsk. The governors who ran Ukraine’s regions under Yanukovych fared no better. Oleksandr Turchynov, who served as acting president from February to June of that year, did what all Ukrainian presidents do: he fired the existing governors and replaced them with figures friendly to the new regime.
What is the impact of such political turnover on economic performance? In principle, replacement of political elites can have profound consequences for enterprise owners and managers, who rely on the support of patrons in government for government contracts, direct and indirect subsidies, the security of property rights, and permits to do business. In a system without effective checks and balances, economic policy can also swing widely as power passes from one group to another. Yet little is known about the impact of such changes on firm productivity, a major driver of economic welfare.
We examine the impact of political turnover on productivity and other aspects of firm performance in “The Productivity Consequences of Political Turnover: Firm-Level Evidence from Ukraine’s Orange Revolution” (Earle and Gehlbach, 2015). Our main finding is that the productivity of firms in regions that supported Yushchenko, the eventual winner of the 2004 presidential election, increased after the Orange Revolution, relative to that of firms in regions that supported Yanukovych, the chosen successor of incumbent President Leonid Kuchma. These results demonstrate that political turnover in a context of weak institutions can have major efficiency consequences as measured by differences in firm productivity.
Ukraine in 2004
Three factors make Ukraine in 2004 an appropriate setting for identifying the effect of political turnover on economic performance. First, Ukraine under Kuchma was a paradigmatic case of “patronal presidentialism,” in which the president “wields not only the powers formally invested in the office but also the ability to selectively direct vast sources of material wealth and power outside of formal institutional channels” (Hale 2005, p. 138). Who won the presidential contest had enormous implications for economic activity.
Second, economic and political power was regionally concentrated in Ukraine’s Russian-speaking East—Yanukovych himself was closely affiliated with oligarchs in Donetsk—while the political opposition represented by Yushchenko had its base in the ethnically Ukrainian and less industrialized West. Voting in Ukraine’s 2004 presidential election reflected this regional divide.
Third, few gave Yushchenko much chance of winning the presidency until the presidential campaign was well underway. In the end, it took not only a highly contested election, but also sustained street protests to wrest power from the existing elite.
Together, these considerations imply not only that political turnover in Ukraine could have an impact on firm performance, but also that any such effect could be observed by comparing the performance of enterprises in regions supportive of the two candidates before and after Yushchenko’s unexpected election victory.
The Orange Revolution and Firm Performance
To analyze the impact of political turnover, we use data on over 7,000 manufacturing enterprises that we track over many years, both before and after the Orange Revolution. We compare the evolution of productivity across firms in regions by vote in the 2004 election that was won by Yushchenko, while controlling for any shocks to particular industries in any year, for constant differences across firms in the level or trend of their productivity, and for regional differences in industrial structure. This design avoids many of the other influences on firm-level productivity that might have coincided with the Orange Revolution.
Our primary finding is that the productivity of firms in regions that supported Yushchenko in 2004 increased after Yushchenko took power, relative to the productivity of firms in regions that supported Yanukovych (and, implicitly, his patron Kuchma, whom Yushchenko succeeded as president). This effect is most pronounced among firms that had the most to gain or lose from presidential turnover: firms in sectors that rely on government contracts; private enterprises, given Ukraine’s weak property rights; and large enterprises. Other measures of economic performance suggest that these results are driven by favorable treatment of particular firms, either before or after the Orange Revolution, rather than by broad changes in economic policy.
Conclusion
Political turnover is often desirable. Nonetheless, our results suggest that the distributional consequences can be profound when institutions are weak, that is, when access to those in power is the primary guarantee of market access, contract enforcement, and property-rights protection. Oscillation of privilege from one region or sector to another is inefficient, as firms initiate or postpone restructuring based on who is in power. The optimal solution, of course, is not to restrict turnover, but to make turnover safe for economic activity. This requires that institutions be reformed to guarantee equal treatment for all economic actors—a difficult process that has proceeded with fits and starts in post-Yanukovych Ukraine.
References
- Earle, John S.; and Scott Gehlbach, 2015. “The Productivity Consequences of Political Turnover,” American Journal of Political Science, 59(3), 708–723.
- Hale, Henry E, 2005. “Regime Cycles: Democracy, Autocracy, and Revolution in Post-Soviet Eurasia,” World Politics, 58(1), 133–65.
Why Do Scientists Move? The Mobility of Scientists
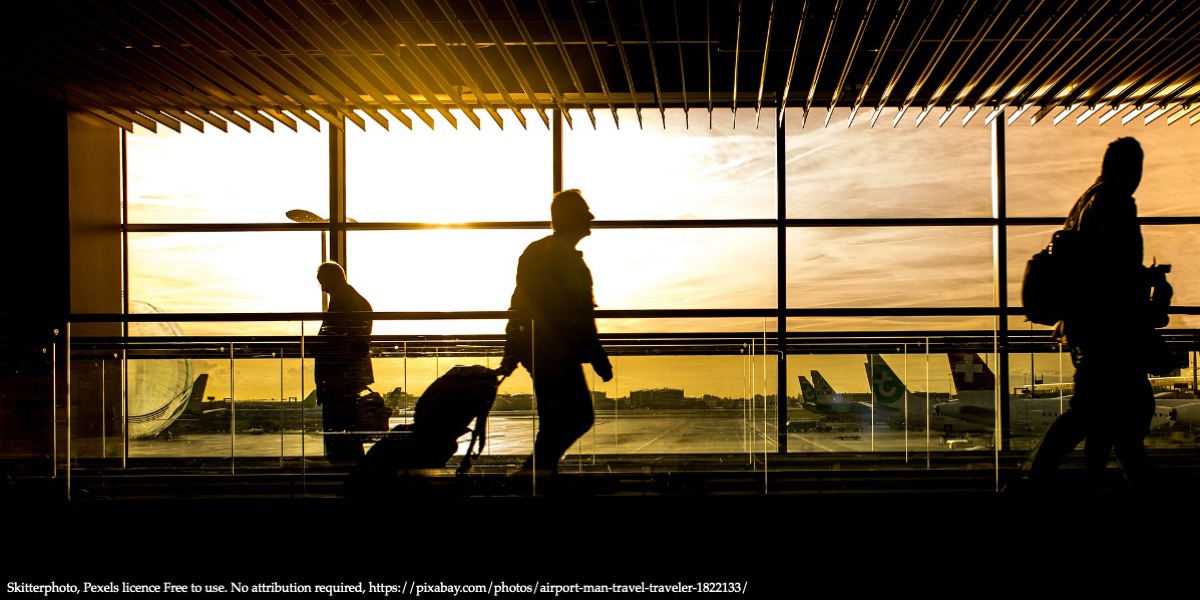
This policy brief provides an overview of new evidence on the determinants of the mobility of scientists – high human capital workers who generate new ideas and expand the frontier of knowledge. New evidence from a large dataset of elite US life scientists shows that professional factors, including individual productivity and the quality of a scientist’s peer environment, matter for mobility. Strikingly, family structure also plays a significant role, with the likelihood of moving to decrease when a scientist’s children are in high school (14-17 years old). This suggests that even “star” scientists take into account more personal, family factors in their mobility decisions, likely due to the costs associated with disrupting their children’s social networks.
Workers often face an important decision during their career: should I move to a new city for a new job? Relocation is a complex decision that can involve numerous professional and personal factors, and some of these factors can constrain moves while others facilitate them. Relocating can, on one hand, mean significant transition costs in terms of uprooting one’s family and navigating a new city and workplace; on the other hand, it can open up new career opportunities and provide environments where one’s skills are put to better use.
Why should we care about whether and why workers move? Economic theory suggests that mobility is one channel through which worker productivity can be increased by improving the employer-employee “match”. Moreover, particularly for highly-skilled workers, the theory suggests that the mobility of workers can impact the productivity of their peers; if the human capital of the mobile worker “spills over” to their peers, then the peers left behind would experience a decline in productivity and those at the new destination would get a boost.
In light of this, understanding the mobility of scientists – high human capital workers who are generating new ideas and expanding the frontier of knowledge – is of particular importance when considering the potential role that mobility can play in increasing productivity and innovation, which are central to models of economic growth.
The Determinants of Mobility
While there is a growing literature trying to document how the mobility of scientists can impact their own productivity and the productivity of their peers (see e.g. Agrawal, McHale, & Oettl, 2014), a significant challenge is finding plausibly exogenous variation in both the timing and location choices of movers. In order to fully understand the impacts of mobility, we first need to know more about the determinant of mobility: why and when in their careers scientists move.
Several studies have examined the determinants of the mobility of scientists and inventors, but the literature has been hampered by lack of data that allow researchers to observe the relevant factors that may matter for mobility. These studies have tended to focus on professional factors for moves; especially how individual productivity measures, like a scientist’s number of publications, citations and patents, predict moves. Importantly, there has been less attention in this literature on the constraints to mobility, including more personal factors, like the role of children and family, and the quality of one’s peer environment.
The findings from these studies on the role of individual productivity for mobility is mixed with evidence pointing to a positive relationship (Zucker, Darby and Torero, 2002; Lenzi, 2009), and some evidence showing negative (Hoisl, 2007) and/or no effects (Crespi et al, 2007). One key professional factor that has remained underexplored in these studies is the quality of the peer environment, or how one’s colleagues can influence the choice of moving.
Moreover, very few studies have been able to examine non-professional factors such as the role of family and children. There is some evidence on family factors and inventor mobility from Sweden, where detailed data is widely available but within country mobility is relatively low (Ejermo and Ahlin, 2014). There is also some evidence from the sociology of science literature showing that children influence the scientific performance and mobility of scientists. Using data from the U.S. Census, Shauman and Xie (1996) find that children tend to constrain mobility; for women, children negatively impact mobility regardless of the age of the children, while for men, it is older, high school-age children that tend to constrain mobility. However, given that the study uses Census data, individual productivity measures are limited, which are needed to compare the effects for similarly accomplished scientists.
Evidence from Elite Life Scientists in the US
In Azoulay, Ganguli and Graff Zivin (2016) we examine the determinants of mobility of elite life scientists in the U.S. and for the first time, provide evidence on both professional and personal determinants of mobility. We use a unique panel dataset we compiled from the career histories of 10,004 elite life scientists to understand why and when scientists make decisions to move to new locations. We are able to observe the transitions scientists make between institutions, and focus on moves that are at least 50 miles apart (based on distance between the zip codes of the institutions) to increase the likelihood that a transition leads the scientists to change their place of residence.
The dataset includes individual productivity measures of publication counts and the U.S. National Institutes of Health (NIH) funding data. We also measure the quality of the peer environment at the scientists’ origin and destination locations using publication and funding counts of peers. We define peers as both collaborating and non-collaborating peers (those who are close in “idea space”), and those who are geographically close (less than 50 miles apart) and those who are distant (more than 50 miles apart). Finally, to examine the personal factors, for each scientist in our sample, we hand-collected information on their children, including each child’s year of birth.
Through regression analysis, we find that individual productivity is a positive predictor of moves, which is consistent with several other studies (Zucker, Darby and Torero, 2002; Coupé, Smeets & Warzynski, 2006; Lenzi, 2009; Ganguli, 2015). We also provide new evidence on additional professional factors that influence the propensity to move. For example, we find that obtaining recent NIH funding deters moves, perhaps as a result of transaction costs associated with transferring federally funded research between institutions. We also find that a scientists’ peer environment is a significant predictor of mobility, as scientists are less likely to move when the quality of the peer environment near their home institution is high and more likely to move when the quality of the peer environment at distant institutions is high.
Figure 1. Age of Children and Mobility: Age of Oldest Child
Figure 2. Age of Children and Mobility: Age of Youngest Child
Our most striking result is the role that family structure plays for mobility. We find a significant decrease in mobility when scientists’ children are of high school age. The likelihood of moving increases just before their oldest child enters high school, and again when their youngest child is beyond high school age. Figure 1 shows a notable spike in distant moves just before the oldest child in the household enters high school (11-14 years old), while Figure 2 shows a similar spike after the youngest child completes high school (18-20 years old). In both figures, the relationship between age of children and local (less than 50 miles) moves does not show a similar spike. This relationship between mobility and age of children persists in regressions that allow us to control for productivity measures and potential confounders.
Conclusion
This brief has discussed theory and evidence related to scientific mobility. New evidence from a large dataset of elite life scientists shows that while professional factors do matter for mobility, we also find that even “star” scientists take into account more personal, family factors in their mobility decisions, likely due to potential disruptions to the social networks of their children.
Given that there is still little evidence about what drives relocation decisions, it is important for further analysis of these issues, and our study raises several more questions for researchers to examine, many of which have important policy implications. For example, what is it about recent NIH grants that deter mobility – the terms of the grant contracts or costs of moving personnel and equipment? Regarding the family factors, we were unable to look at differences among female and male scientists, but an important question for further research is whether the age of children and other factors appear to affect women’s and men’s relocation decisions differently.
References
- Agrawal, A., McHale, J., & Oettl, A. (2014). Why stars matter, National Bureau of Economic Research Working Paper No. w20012.
- Ahlin L. and Ejermo O. (2015). The patent productivity effects of mobility for a panel of Swedish inventors, DRUID, 2015.
- Azoulay, P., Ganguli, I. and Graff Zivin, J.S. (2016). The Mobility of Elite Life Scientists: Professional and Personal Determinants, National Bureau of Economic Research Working Paper No. w21995.
- Coupé, T., Smeets, V., & Warzynski, F. (2006). Incentives, sorting and productivity along the career: Evidence from a sample of top economists. Journal of Law, Economics, and Organization, 22(1), 137-167.
- Ganguli, I. (2015). “Who Leaves and Who Stays? Evidence on Immigrant Selection from the Collapse of Soviet Science” in Aldo Geuna (ed), Global Mobility of Research Scientists: The Economics of Who Goes Where and Why, Elsevier.
- Hoisl, K. (2007). Tracing mobile inventors—the causality between inventor mobility and inventor productivity. Research Policy, 36(5), 619-636.
- Lenzi, C. (2009). Patterns and determinants of skilled workers’ mobility: evidence from a survey of Italian inventors. Economics of Innovation and New Technology, 18(2), 161-179.
- Shauman, K. A., & Xie, Y. (1996). Geographic mobility of scientists: Sex differences and family constraints. Demography, 33(4), 455-468.
- Zucker, L. G., Darby, M. R., & Torero, M. (2002). Labor Mobility from Academe to Commerce. Journal of Labor Economics, 20(3), 629-660.
Disclaimer: Opinions expressed in policy briefs and other publications are those of the authors; they do not necessarily reflect those of the FREE Network and its research institutes.
Electoral Competition and Political Selection
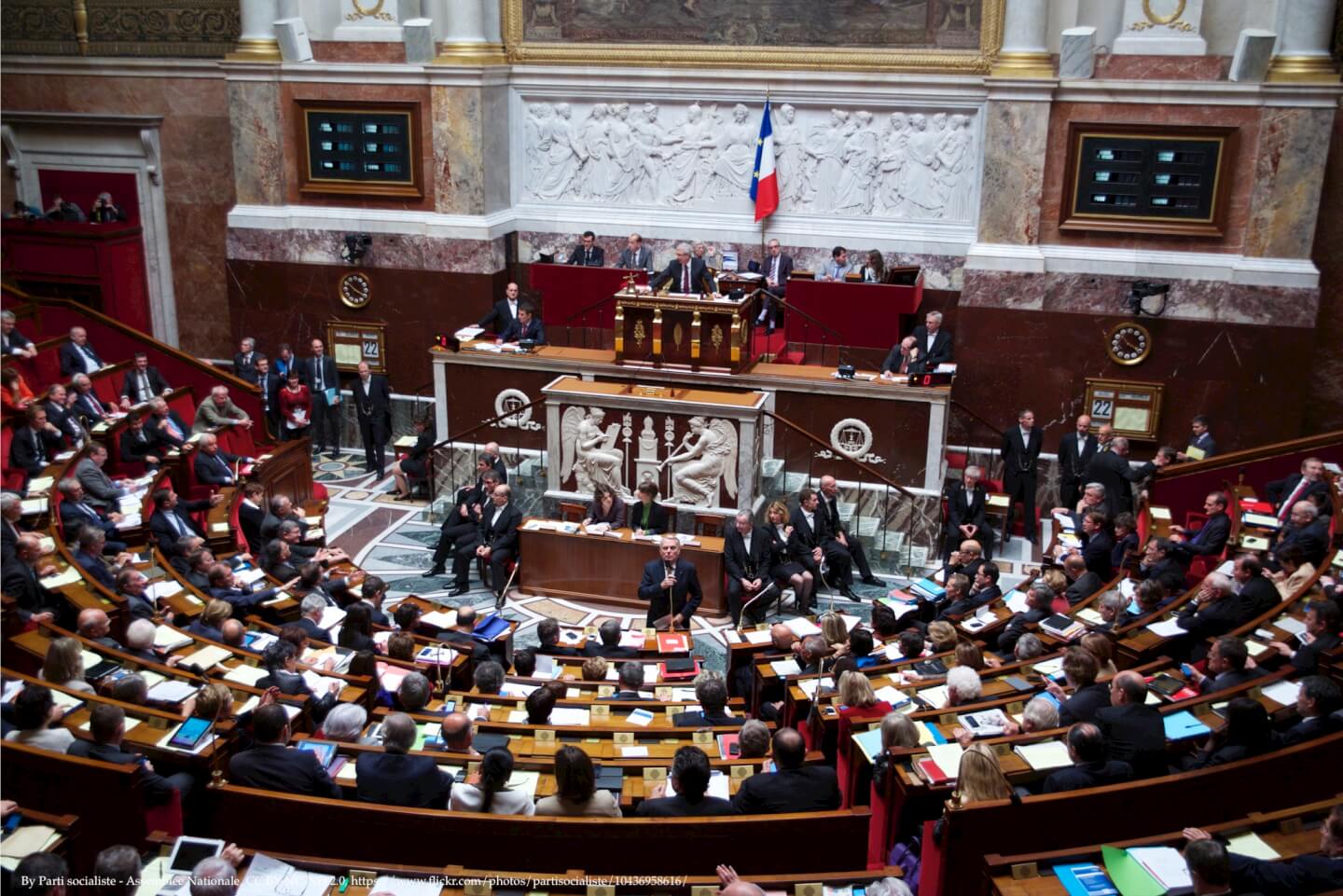
Research in political economy has recently rediscovered that individuals, and not only institutions, matter for economic outcomes: not all politicians are of the same quality. Competition in the political market may have another virtue than its traditional disciplining role: it may favor the selection of good politicians. In a recent paper, Marijn Verschelde and I study the (time-varying) relationship between political competition and the quality of French deputies.
Note: this column draws heavily on a paper written with Marijn Verschelde, which is available here. The figures are taken from it.
How to design the political selection mechanism in order to favor the emergence of “good” politicians? The traditional public-choice literature emphasized the role of institutions in shaping the behavior of politicians, but have paid little attention, if any, to the individual decision maker. From this point of view, it is purely illusive to seek for “morally superior agents who will use their powers in some public interest” (Buchanan, 1989): all politicians are purely self-interested agents who have to be incentivized to act in accordance with voters’ preferences.
However, a recent stream of empirical papers provides evidence that individual politicians matter for economic outcomes (Besley et al., 2011; Jones and Olken, 2005). In other words, as suggested by common sense, they are not all cut from the same cloth. This emphasizes the need not only to shape institutions in order to discipline incumbent politicians, but also to select “good” decision makers.
Several determinants have been shown to affect political selection: the wage of politicians (Besley, 2004; Messner and Polborn, 2004), the transparency of politics (Mattozzi and Merlo, 2007), the institutional flexibility (Acemoglu et al., 2010), and reservation quotas (Besley et al., 2005; Besley et al., 2013). In addition to a well-known disciplining effect (for instance Stigler, 1972, and Becker, 1983), electoral competition is also likely to enhance this selection process as well (Galasso and Nannicini, 2011): competition pushes parties to select candidates of good quality in order to seduce neutral voters, who, contrary to partisans, are sensitive to the quality of the candidates. As recruiting experts are difficult, hence costly, parties will allocate them in the most contested districts only.
In a recent paper, Marijn Verschelde and I investigate the relationship between quality and competition in the case of the French National Assembly. Measuring the quality of politicians is not straightforward. A wide theoretical definition of quality is a combination of competence and motivation. Such a broad definition is challenging to operationalize in empirical work. Some papers approximate quality by variables like level of schooling and experience of politicians (Baltrunaite et al., 2014; Besley et al., 2011; Kotakorpi and Poutvaara, 2011), while others use their absenteeism rate or vote attendance (Galasso and Nannicini, 2011; Gagliarducci and Nannicini, 2013). We innovate by proposing a measure of quality based on productivity, i.e., on what deputies actually do. We gathered from the Assemblée Nationale archive all the information that has been systematically collected over the years for each deputy and for each year from 1958 to 2012: (i) propositions of law, (ii) oral questions, (iii) reports, and (iv) debates in which the deputy has been involved in. From these four aspects of parliamentary work, we compute a composite indicator of productivity (bounded between 0 and 1, higher values indicating higher performance). To measure political competition, we use a measure based on a Herfindahl index of the vote shares at the first round (bounded between 0 and 1, larger values indicating stiffer competition).
Figure 1. Estimated Productivity Conditional on Competition
We document a strong effect of competition on deputies’ productivity, in accordance with the theoretical framework. Figure 1 displays the estimated level of productivity as a function of the degree of competition, controlling for personal (age, schooling, etc.) and political (party, committee, and the likes) variables. Everything else equal, a deputy elected in the a priori most contested district is estimated to reach a productivity close to 0.8, while if she is elected in a safe district her productivity is estimated to be at most 0.6. This means that the productivity of deputies can vary by up to 30% depending on the degree of contestability. This relation holds even after minimizing problems of potential reversed causality and controlling for reelection incentives, indicating that we actually observe a selection effect.
Considering the time span of our dataset, it is interesting to observe how the positive relationship between electoral competition and political selection evolved over time. The most noticeable evolution over the second part of the XXth century in France is certainly the ideological convergence after the 80’s, marked with the reconciliation of the Socialist Party with the market and the rise of the Pensée unique (“one track thinking”, Knapp and Wright, 2001). Such an evolution should have decreased ideological voting and produced more competence-based elections, hence increasing the effect of competition on quality.
Figure 2. Effect of Competition over Time
However, we uncover a clear inverse-U shaped evolution. Figure 2 plots the effect of competition conditional on the year: the effect of electoral competition sharply increased till the 1980’s, but it has continuously decreased since then. How to explain this pattern? A possible explanation is the increased voters volatility, i.e., a higher unpredictability in voters’ behavior. If at first sight this phenomenon indicates an increase in the share of neutral voters, this is however not necessarily the case. Instead of moving from partisanship to neutrality, it is possible that supporters of a party A at election t turn into supporters of party B at election t+1. Partisans disappointed by the behavior of their party while in office might provide an unconditional support to the competing party at the next election. This is consistent with the fact that not any party succeeded to win two national elections in a row since the 80’s.
Conclusion
Our analysis point out a clear positive relationship between electoral competition and the quality of the French deputies, measured by a composite indicator of productivity. Overall, deputies elected in contested districts tend to perform better than others. From a public policy perspective, this result suggests that reforms enhancing political competition (for instance by redistricting jurisdiction in a proper way or by ensuring a fair access to media to competing parties) should be supported. However, we observe that the impact of competition increased till the 80’s, but continuously decreases since then. This opens the door for a vast research agenda, as it indicate that drivers of an efficient selection mechanism are not necessarily stable over time.
▪
References
- Acemoglu, D., Egorov, G., Sonin, K., 2010. Political selection and persistence of bad government. Quarterly Journal of Economics 125 (4), 1511-1575.
- Becker, G.S., 1983. A theory of competition among pressure groups for political influence. Quarterly Journal of Economics 97, 371-400.
- Baltrunaite, A., Bello, P., Casarico, A., Profeta, P., 2014. Gender quotas and the quality of politicians. Journal of Public Economics 118, 62–74.
- Besley, T., Montalvo, J., Reynal-Querol, M., 2011. Do educated leaders matter? Economic Journal 121, 205-227.
- Besley, T., Pande, R., Rao, V., 2005. Political selection and the quality of government: Evidence from south india. PEPP/8, Suntory and Toyota International Centres for Economics and Related Disciplines, London School of Economics and Political Science, London, UK.
- Besley, T., Folke, O., Persson, T., Rickne, J., 2013. Gender quotas and the crisis of the mediocre man: Theory and evidence from sweden. IFN Working Paper 985.
- Buchanan, J., 1989. Constitutional Economics, Explorations into Constutional Economics. College Station, TX: Texas A & M University Press.
- Gagliarducci, S., Nannicini, T., 2013. Do better paid politicians perform better? disentangling incentives from selection. Journal of the European Economic Association 11 (2), 369-398.
- Galasso, V., Nannicini, T., 2011. Competing on good politicians. American Political Science Review 105 (1), 79-99.
- Gavoille, N., Verschelde, M., 2015. Electoral competition and political selection: An analysis of the productivity of French politicians, 1958-2012. Condorcet Center working paper series.
- Jones, B., Olken, B., 2005. Do leaders matter? National leadership and growth since World War II. Quarterly Journal of Economics 120 (3), 835-864.
- Knapp, A., Wright, V., 2001. The governmentand politics of France. Routledge.
- Kotakorpi, K., Poutvaara, P., 2011. Pay for politicians and candidate selection: An empirical analysis. Journal of Public Economics 95, 877–885.
- Messner, M., Polborn, M., 2004. Paying politicians. Journal of Public Economics 88, 2423-2445.
- Mattozzi, A., Merlo, A., 2007. The transparency of politics and the quality of politicians. American Economic Review 97 (2), 311-315.
- Stigler, G., 1972. Economic competition and political competition. Public Choice 13, 91–106.
Does Service-Sector Liberalization Increase Productivity in the Manufacturing Sector?
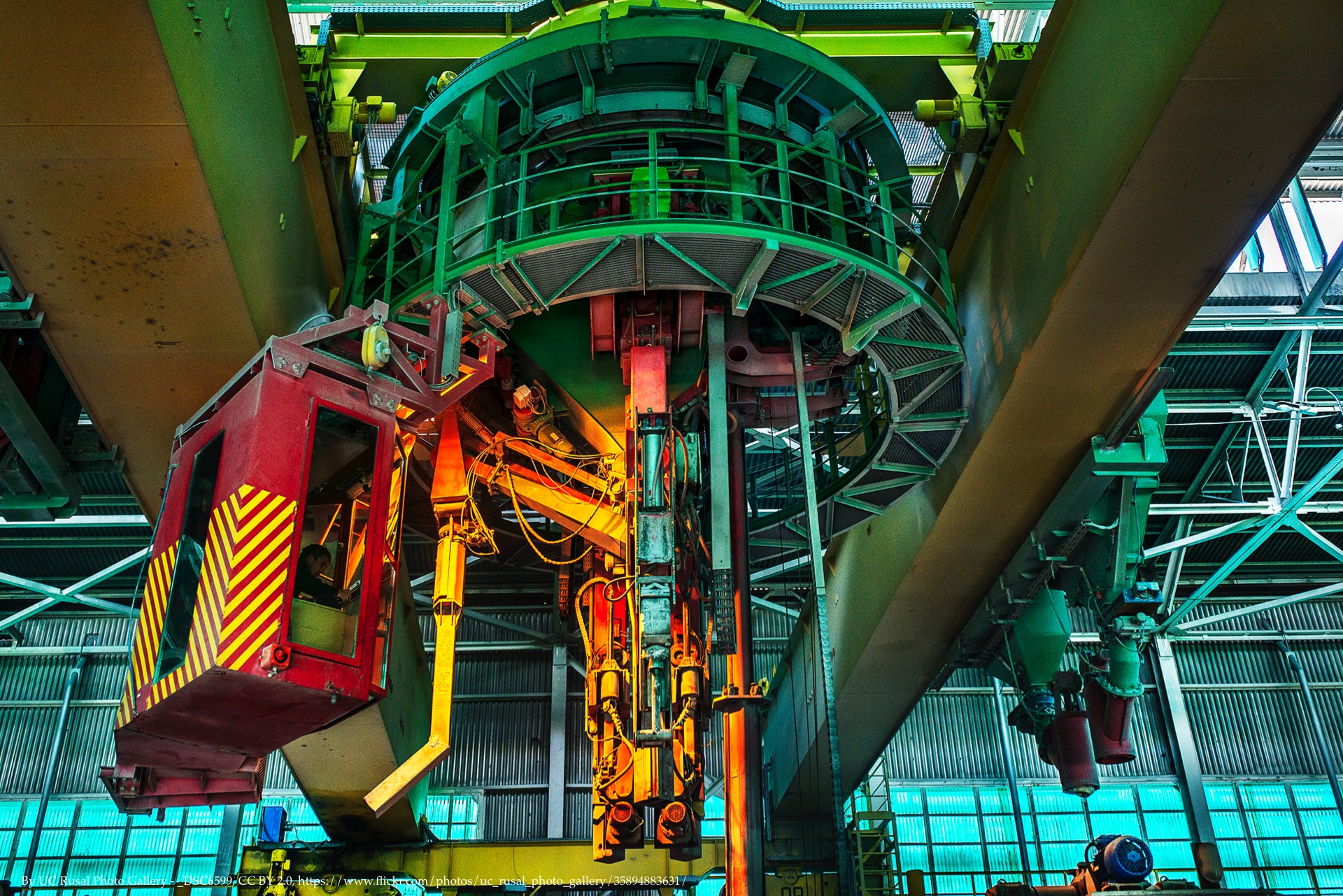
Authors: Oleksandr Shepotylo and Volodymyr Vakhitov, KEI.
This policy brief summarizes the results of recent research on the effect of service-sector liberalization in Ukraine, 2001-2007, on productivity in the manufacturing sector. We use a sample of manufacturing firms and construct a firm-specific index of service-sector liberalization. We find that the manufacturing firms which more intensively use liberalized services, on average, have gained 9 percent in total factor productivity (TFP). The service liberalization is associated with increased foreign presence which also has a positive and significant effect on TFP. The effect is stronger for domestic and small firms.