Tag: Russia
Short-Run and Long-Run Effects of Sizeable Child Subsidy: Evidence from Russia
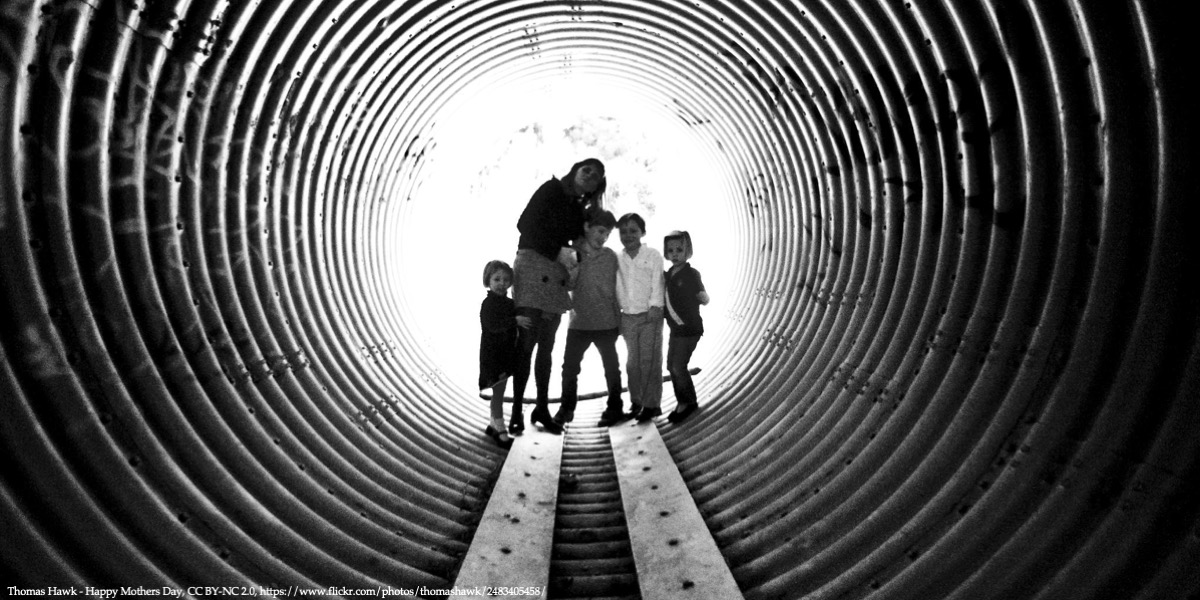
How to design the optimal pro-natalist policy is an important open question for policymakers around the world. Our paper utilizes a large-scale natural experiment aimed to increase fertility in Russia. Motivated by a decade-long decrease in fertility and population, the Russian government introduced a sequence of sizable child subsidies (called Maternity Capitals) in 2007 and 2012. We find that the Maternity Capital resulted in a significant increase in fertility both in the short run and in the long run. The subsidy is conditional and can be used mainly to buy housing. We find that fertility grew faster in regions with a shortage of housing and with a higher ratio of subsidy to housing prices. We also find that the subsidy has a substantial general equilibrium effect. It affected the housing market and family stability. Finally, we show that this government intervention comes at substantial costs.
In all European and Northern American countries the fertility is below the replacement level (United Nations, 2017). Following this concern, most of the developed countries have implemented various large scale and expensive pro-natalist policies. Yet, the effectiveness of these policies is unclear, and the design of the optimal pro-natalist policy remains a challenge.
There are several important open research questions on the evaluation of these programs. The first is whether these programs can induce fertility in the short-run and/or in the long-run horizon. Indeed, very few of these expensive and large-scale policies are proved to be an effective tool to increase fertility (Adda et al, 2017). The next set of questions deals with further evaluation of the programs: What are the characteristics of families that are affected by this policy? How costly is the policy, i.e. how much is the government paying per one birth that is induced by the policy? Finally, what are the non-fertility related effects of these policies? While most of the studies that analyze the effect of pro-natalist policies concentrate on fertility and mothers’ labor market outcomes, these, usually large-scale, policies may have important general equilibrium and multiplier effects that may affect economies both in the short run and long run (Acemoglu, 2010).
In our paper we utilize a natural experiment aimed to increase fertility in Russia to address these questions.
Motivated by a decade-long decrease in fertility and depopulation, the Russian government introduced a sizable conditional child subsidy (called Maternity Capital). The program was implemented in two waves. The first wave, the Federal Maternity Capital program, was enacted in 2007. Starting from 2007, a family that already has at least one child, and gives birth to another, becomes eligible for a one-time subsidy. Its size is approximately 10,000 dollars, which exceeds the country’s average 18-month wage and exceeds the country’s minimum wage over a 10-year period. The recipients of the subsidy can use it only on three options: on housing, the child’s education, and the mother’s pension. Four years later, at the end of 2011, Russian regional governments introduced their own regional maternity programs that give additional – on the top of the federal subsidy – money to families with new-born children.
In our paper, we document that the Maternity Capital program results in a significant increase in fertility rates both in the short run (by 10%) and in the long run (by more than 20%). This effect can be seen from both within-country analysis and from comparing the long-term growth of fertility rates in Russia with Eastern and Central European countries that face similar economic conditions and had similar pre-reform fertility trends. Like Russia, Eastern European countries experienced a drop in fertility rates right after the collapse of the Soviet Union and had similar trends in fertility up until 2007. Our results show that while having similar trends in fertility before 2007, afterward Russia significantly surpassed all the countries from this comparison group.
Figure 1 illustrates the effect of the Maternity Capital on birth rates. The top two panels show monthly birth rates (simple counts and de-seasoned); the bottom panels show total fertility rates in Russia versus Eastern European countries, and versus the European Union and the US.
Figure 1. Total Fertility Rate, Russia, Eastern European countries, USA and EU.
Source: Sorvachev and Yakovlev (2019), and http://www.fertilitydata.org/.
The effects of the policy are not limited to fertility. This policy affects family stability: it results in a reduction in the share of single mothers and in the share of non-married mothers.
Also, the policy affects the housing market. Out of three options (education, housing and pension), 88% of families use Federal Maternity Capital money to buy housing. We find that the supply of new housing and housing prices increased significantly as a result of the program. Confirming a close connection between the housing market and fertility, we find that in regions where the subsidy has a higher value for the housing market, the program has a larger effect: the effect of maternity capital was stronger, both in the short run and long run, in regions with a shortage of housing, and in regions with a higher ratio of subsidy to price of apartments (i.e. those regions where the real price of subsidy as measured in square meters of housing is higher).
Figure 2 below shows the effect of Federal Maternity Capital on birth rates in different regions. It shows no effect on fertility in Moscow, small effect in Saint-Petersburg; whereas the sizable effect of maternity capital in other Russian regions.
Figure 2. Effect of Federal Maternity capital, by regions
Source: Sorvachev and Yakovlev (2019), and http://www.gks.ru/.
These results suggest that cost-benefit analysis of such policies should go beyond the short-run and long-run effects on fertility. Ignoring general equilibrium issues may result in substantial bias in the evaluation of both short-run and long-run costs and benefits of the program.
While there are many benefits of the program, we show that this government intervention comes at substantial costs: the government’s willingness to pay for an additional birth induced by the program equals approximately 50,000 dollars.[1]
For more detailed evaluation of the results see Evgeny Yakovlev and Ilia Sorvachev, 2019, “Short-Run and Long-Run Effects of Sizable Child Subsidy: Evidence from Russia”, NES working Paper # 254, 2019.
References
- Acemoglu, Daron 2010 “Theory, General Equilibrium, Political Economy and Empirics in Development Economics”, Journal of Economic Perspectives, 24(3), pp. 17-32. 2010
- Adda, Jérôme, Christian Dustmann and Katrien Stevens 2017. “The Career Costs of Children”. Journal of Political Economy, 125, 2, 293-337.
- Ilia Sorvachev and Evgeny Yakovlev, 2019, “Short-Run and Long-Run Effects of Sizable Child Subsidy: Evidence from Russia”, NES working Paper #254 and LSE IGA Research Working Paper Series 8/2019
[1] Roughly, the WTP (US$50,000) exceeds nominal US$10,000 subsidy because the government pays for all (100%) families that give birth to a child to induce additional (20%) increase in fertility. See paper for more accurate elaboration.
Social Media and Xenophobia
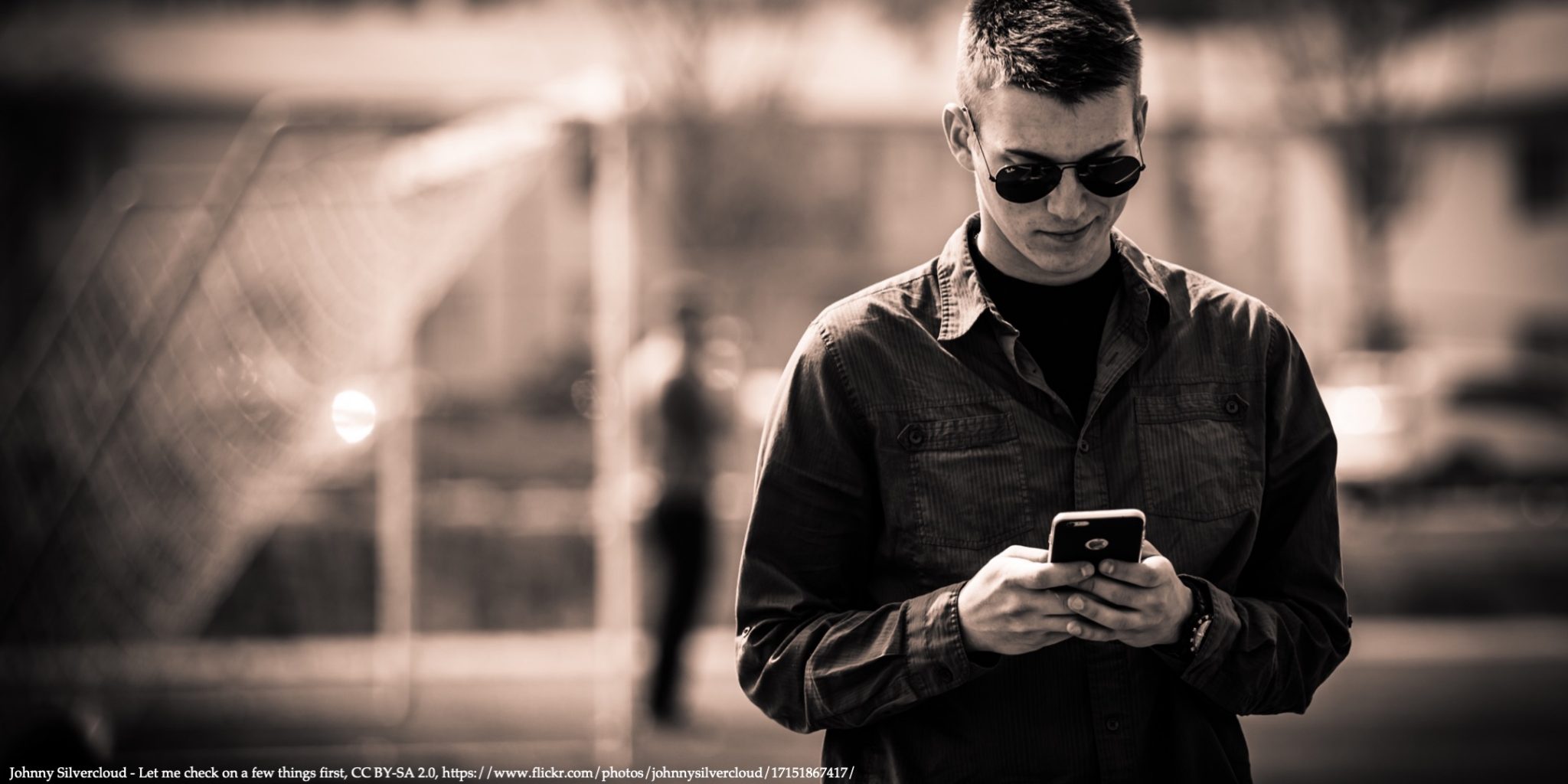
We study the causal effect of social media on hate crimes and xenophobic attitudes in Russia, using variation in social media penetration across cities. We find that higher penetration of social media leads to more ethnic hate crimes, but only in cities with a high baseline level of nationalist sentiment prior to the introduction of social media. Consistent with a mechanism for the coordination of crimes, the effects are stronger for crimes with multiple perpetrators. We show that social media penetration also had a persuasive effect on young and uneducated individuals, who became more likely to have xenophobic attitudes.
In recent years, the world has witnessed a large increase in expressions of hate, particularly of xenophobia. Candidates and platforms endorsing nationalism and views associated with intolerance toward specific groups have also gathered increased popular support both in the U.S. and across Europe. There is a lot of speculation about the potential drivers of this increase in the expression of hate. In our recent paper (Enikolopov et al, 2019) we study the role of social media in this process. This brief introduces the topic and offers a short outline of our findings.
Conceptually, social media could foster hate being expressed through different channels. First, social media reduces the cost of coordination. For example, there is evidence that it facilitates political protest (Enikolopov, Makarin, Petrova, 2018). Coordination facilitated through social media might be particularly relevant for illegal and stigmatized activities, such as hate crime: social media might make it easier to find like-minded people (through targeted communities and groups); it might also reduce the cost of asking or exposing oneself by providing a more anonymous forum for social interactions. Social media might also influence people’s opinions: tolerant individuals might be more exposed to intolerant views, while intolerant individuals might end up in an “echo chamber” (Sunstein 2001, 2017, Settle 2018) that make their views even more extreme. In our paper, we study the causal effect of social media exposure on xenophobic crimes and xenophobic attitudes in Russia and provide evidence on the particular mechanisms behind these effects.
The challenge in identifying a causal effect of social media is that access and consumption of social media are not randomly assigned. To surmount this challenge, we follow the approach of Enikolopov et al. (2018) and exploit a feature of the introduction of the main Russian social media platform – VKontakte (VK). This social media, which is analogous to Facebook in functionality, was the first mover on the Russian market and secured its dominant position with a user share of over 90% by 2011. VK was launched in October 2006 by Pavel Durov, its founder, who at that time was an undergraduate student at St Petersburg State University (SPbSU). Initially, users could only join the platform by invitation, through a student forum of the University, which was also created by Durov.
As a result, the vast majority of the early users of VK were students of SPbSU. This, in turn, made their friends and relatives more likely to open an account. And since SPbSU attracted students from around the country, this sped up the development of VK in the cities, from which these students were coming from. Network externalities magnified these effects and, as a result, the idiosyncratic variation in the distribution of the home cities of Durov’s classmates had a long-lasting effect on VK penetration. Following this logic, we use fluctuations in the distribution of student of SPbSU across cities as an instrument for the city-level penetration of VK. We then evaluate the effect of higher VK penetration on hate crimes and hate attitudes, combining data on hate crimes for the period between 2007 and 2015 collected by a reputable Russian NGO SOVA with survey data on hate attitudes.
Previous findings indicate that whether information from media induces people to be involved in the active manifestation of xenophobic attitudes or not depends on predispositions of the population. For example, Adena et al (2015) demonstrate that radio propaganda by the Nazis in the 1930’s was effective only in areas with a historically high levels of anti-Semitism. The role of the underlying level of nationalism is likely to be even stronger for social media, in which the content of the media itself directly reflects the attitudes of the population. This is particularly relevant for hate crimes committed by multiple perpetrators, in which social media can facilitate the coordination of such crimes.
Thus, we test whether the effect of social media depends on the pre-existing level of nationalism. To get at this underlying sentiment, we break cities by their level of support for the Rodina (“Motherland”) party, which ran in the national 2003 elections (the last parliamentary elections before the creation of VK) on an explicit nationalistic, xenophobic platform.
We find that penetration of social media leads to more ethnic hate crimes, but only in cities with a high baseline level of nationalist sentiment prior to the introduction of social media. For example, in cities with a maximum level of support of Rodina an increase in the number of VK users by 10% lead to an increase in ethnic hate crimes by 20%, while it had no significant effect on hater crime in cities with minimal support of Rodina. There is also no evidence that future social media penetration is related to ethnic hate crimes before the creation of social media, regardless of the level of pre-existing nationalistic attitudes.
Further evidence is consistent with social media playing a coordination role in hate crimes. The effect of social media is stronger for crimes perpetrated by multiple individuals (as opposed to crimes committed by a single person), where coordination is more important. These heterogeneous effects are also not consistent with results being simply driven by a higher likelihood of hate crime in places with higher social media penetration, unless this effect were present precisely in cities with higher support for Rodina and for crimes with multiple perpetrators, for example – which we find unlikely.
Having found evidence of a causal effect of social media on ethnic hate crimes, consistent with a mechanism of coordination, we turn next to the impact of social media on xenophobic attitudes. We designed and organized an online survey, and launched it in the summer of 2018, reaching 4,327 respondents from 64 cities. To measure xenophobic attitudes, we examined answers to the question “Do you feel irritation of dislike for individuals from some other ethnicities?” Note that, unlike the coordination of hate crimes, the persuasive effects of social media are not necessarily expected to be strongest in cities with higher baseline nationalistic sentiment since individuals on social media can get as easily connected to people outside their city. In fact, it is conceptually possible that the persuasion would be stronger in cities with lower baseline nationalistic sentiment: individuals might have previously been less aware of and less exposed to these types of views before the introduction of social media.
Since there might be a stigma in reporting xenophobic attitudes even in anonymous surveys, we use a “list experiment” to approximate “truly-held” xenophobic attitudes. In particular, the list experiment works as follows: first, respondents are randomly assigned either into a control group or a treatment group. Respondents in all groups are asked to indicate the number of policy positions they support from a list of positions on several issues. Support for any particular policy position is never indicated, only the total number of positions articulated on the list that a respondent supports. In the control group, the list includes a set of contentious, but not stigmatized, opinions. In the treatment group, the list includes all the contentious opinions from the control list, but also adds the opinion of interest, which is potentially stigmatized. The degree of support for the stigmatized opinion can be assessed by comparing the average number of issues supported in the treatment and control groups. The question of interest, randomly added to half of the questionnaires, was “Do you feel irritation of dislike for individuals from some other ethnicities?”.
The results indicate that the average share of people who agree with the statement is 37%. While there is no significant effect of social media penetration on xenophobic attitudes for the whole sample, there is a significant effect for important subsamples, which are at a higher risk of being involved in hate crime, such as respondents with lower levels of education or young respondents. Of course, the individuals that became more likely to engage in hate crime are not necessarily the same that have been persuaded to have more xenophobic attitudes (especially given the question used to assess attitudes) – though it is possible that some individuals who would have been close to committing crimes in the absence of social media might have been persuaded enough to switch their behavior in the presence of social media.
At the same time, we do not find that social media leads to an increase in xenophobic attitudes when measured with a direct question. The results are confirmed if we use a much larger, nationally representative survey of more than 30,000 respondents conducted by one of the biggest Russian survey companies FOM in 2011. In principle, it is possible that social media not only changed real attitudes but also the perception of the social acceptability of expressing these attitudes. However, we do not find any evidence that social media reduces the stigma of admitting xenophobic attitudes. The fact that we find the effect of social media on actual attitudes, but not on the expressed ones suggests, that if anything the stigma increased, at least for the respondents who acquired xenophobic attitudes as a result of social media influence. This highlights the importance of using a survey method that reduces concerns with social acceptability, such as list experiments.
Overall, our results indicate that social media lead to an increase in both ethnic hate crimes and xenophobic attitudes in Russia. However, the effect on hate crime is observed only in cities in which there was already a high level of nationalism. Additional evidence indicates that this effect is driven both by facilitating the coordination of nationalists and by persuading people to become more xenophobic. These findings contribute to a growing body of evidence that social media is a complex phenomenon that has both positive and negative effects on the welfare of people (see also Allcott et al, 2019), which has to be taken into account in discussing policy implications of the recent changes in media technologies.
References
- Allcott, H., Braghieri, L., Eichmeyer, S., Gentzkow, M. (2019) “The Welfare Effects of Social Media”, Working paper.
- Burzstyn, L., Egorov, G., Enikolopov, R., Makarin, A. (2019) “Social Media and Xenophobia: Evidence from Russia”, Working paper.
- Enikolopov, R., Makarin, A., Petrova, M. (2018) “Social Media and Protest Participation: Evidence from Russia“, Working paper.
- Settle, J. E. (2018) Frenemies: How Social Media Polarizes America. Cambridge University Press.
- Sunstein, C. (2001) Republic.com. Princeton University Press.
- Sunstein, C. (2017) Republic: Divided Democracy in the Age of Social Media. Princeton University Press.
Disclaimer: Opinions expressed in policy briefs and other publications are those of the authors; they do not necessarily reflect those of the FREE Network and its research institutes.
Russia’s Real Cost of Crimean Uncertainty
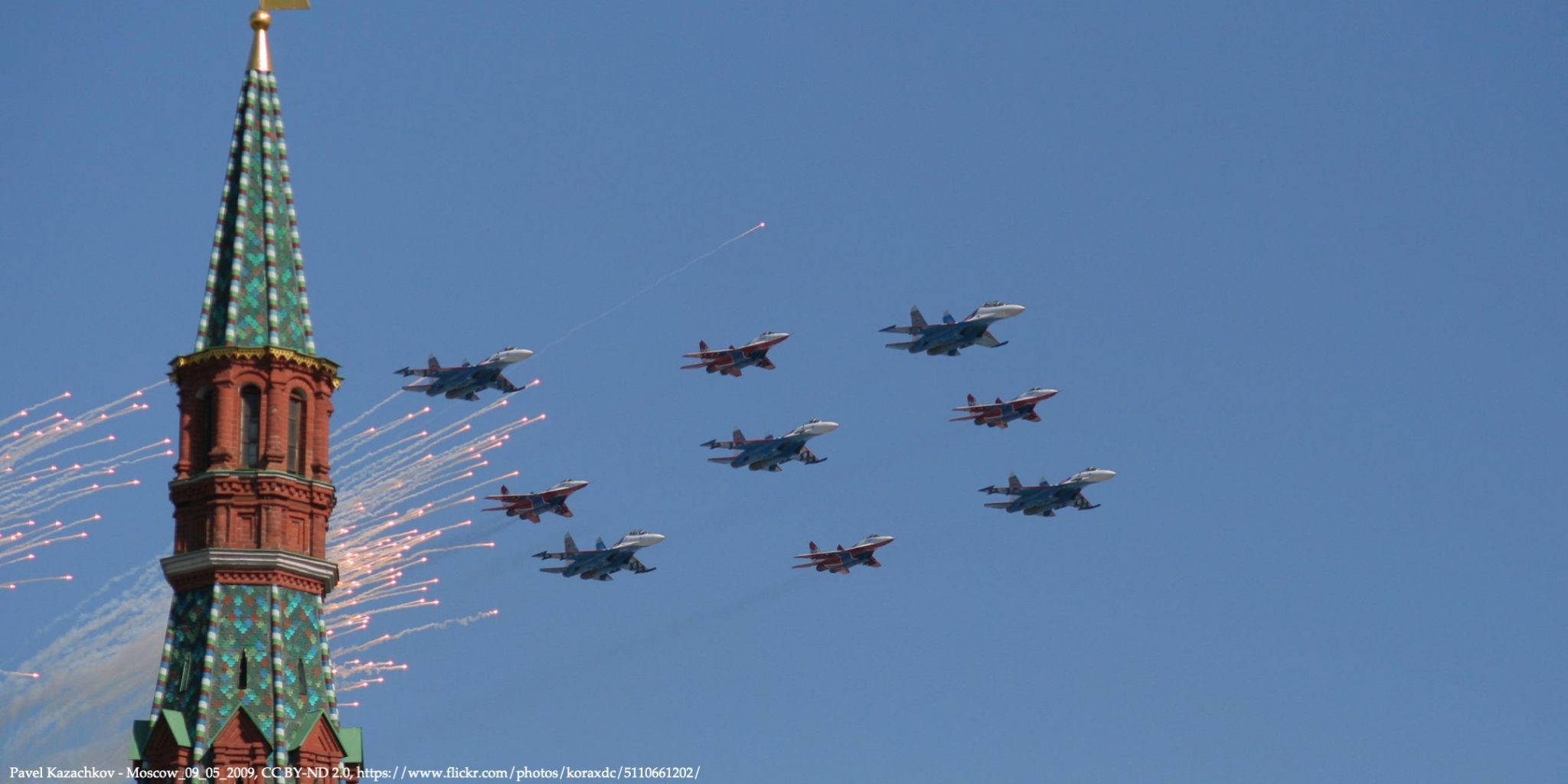
The annexation of Crimea has real costs to the Russian economy beyond what is measured by some items in the armed forces’ budget; social spending in the occupied territories; or the cost of building a rather extreme bridge to solve logistics issues. Russia’s real cost of the annexation of Crimea is also associated with the permanent loss of income that the entire Russian population is experiencing due to increased uncertainty, reduced capital flows and investment, and thus a growth rate that is significantly lower than it would have been otherwise. Since the years of lost growth are extremely hard to make up for in later years, there will be a permanent loss of income in Russia that is a significant part of the real cost of annexing Crimea and continuing the fighting in Eastern Ukraine. It is time to stop not only the human bleeding associated with Ukraine, but also the economic.
Estimating the real cost of Russia’s annexation of Crimea and the continued involvement in Eastern Ukraine is complicated since there are many other things going on in the Russian economy at the same time. In particular, oil prices fell from over $100/barrel in late 2013 to $30/barrel in 2016 (Figure 1). Becker (2016) has shown that 60-80 percent of the variation in GDP growth can be explained by changes in oil prices, so this makes it hard to just look at actual data on growth to assess the impact of Crimea and subsequent sanctions and counter sanctions.
Figure 1. Russian GDP and oil price
Source: Becker (2019)
The approach here is instead to focus on one channel that is likely to be important for growth in these circumstances, which is uncertainty and its impact on capital flows and investment.
From uncertainty to growth
The analysis presented here is based on several steps that link uncertainty to GDP growth. All the details of the steps in this analysis are explained at some length in Becker (2019). Although this brief will focus on the main assumptions and estimates that are needed to arrive at the real cost of Crimea, a short description of the steps is as follows.
First of all, in line with basic models of capital flows, investors that can move their money across different markets (here countries) will look at relative returns and volatility between different markets. When relative uncertainty goes up in one market, capital will leave that market.
The next step is that international capital flows affect investment in the domestic market. If capital leaves a country, less money will be available for fixed capital investments.
The final step is that domestic investments is important for growth. Mechanically, in a static, national accounts setting, if investments go down, so does GDP. More long term and dynamically, investments have a supply side effect on growth, and if investments are low, this will affect potential as well as actual growth negatively.
These steps are rather straightforward and saying that uncertainty created by the annexation of Crimea leads to lower growth is trivial. What is not trivial is to provide an actual number on how much growth may have been affected. This requires estimates of a number of coefficients that is the empirical counterparts to the theoretical steps outlined here.
Estimates to link uncertainty to growth
In short, we need three coefficients that link: domestic investments to growth; capital flows to domestic investments; and uncertainty to capital flows.
There are many studies that look at the determinants of growth, so there are plenty of estimates on the first of these coefficients. Here we will use the estimate of Levine and Renelt (1992), that focus on finding robust determinants of growth from a large set of potential explanatory variables. In their preferred specification, growth is explained well by four variables, initial income, population growth, secondary education and the investments to GDP ratio. The coefficient on the latter is 17.5, which means that when the investment to GDP ratio increases by 10 percentage points, GDP grows an extra 1.75 percentage points per year. Becker and Olofsgård (2018) have shown that this model explains the growth experience of 25 transition countries including Russia since 2000 very well, which makes this estimate relevant for the current calculation.
The next coefficient links capital flows to domestic investments. This is also a subject that has been studied in many empirical papers. Recent estimates for transition countries and Russia in Mileva (2008) and Becker (2019) find an effect of FDI on domestic investments that is larger than one, i.e., there are positive spillovers from FDI inflows to domestic investments. Here we will use the estimate from Becker (2019) that finds that 10 extra dollars of FDI inflows are associated with an increase of domestic investments of 15 dollars.
Finally, we need an estimate linking uncertainty with capital flows. There are many studies looking at risk, return and investment in general, and also several studies focusing on international capital flows and uncertainty. Julio and Yook (2016) look at how political uncertainty around elections affect FDI of US firms and find that FDI to countries with high institutional quality is less affected by electoral uncertainty than others. Becker (2019) estimates how volatility in the Russian stock market index RTS relative to the volatility in the US market’s S&P 500 is associated with net private capital outflows. The estimate suggests that when volatility in the RTS goes up by one standard deviation, this is associated with net private capital outflows of $30 billion.
These estimates now only need one more thing to allow us to estimate how much Crimean uncertainty has impacted growth and this is a measure of the volatility that was created by the annexation of Crimea.
Measuring Crimean uncertainty
In Becker (2019), the measure of volatility that is used in the regression with net capital outflows is the 60-day volatility of the RTS index. Since we now want to isolate the uncertainty created by Crimea related events, we need to take out the volatility that can be explained by other factors in order to arrive at a volatility measure that captures Crimean induced uncertainty. In Becker (2019) this is done by running a regression of RTS volatility on the volatility of international oil prices and the US stock market as represented by the S&P 500. The residual that remains after this regression is the excess volatility of the RTS that cannot be explained by these two external factors. The excess volatility of the RTS index is shown in figure 2.
It is clear that the major peaks in excess volatility are linked to Crimea related events, and in particular to the sanctions introduced at various points in time. From March 2014 to March 2015, there is an average excess volatility of 0.73 standard deviations with a peak of almost 4 when the EU and the USA ban trade with Crimea. This excess volatility is our measure of the uncertainty created by the annexation of Crimea.
Figure 2. RTS excess volatility
Source: Becker (2019)
From Crimean uncertainty to growth
The final step is simply to use our measure of Crimean induced uncertainty together with the estimates that link uncertainty in general to growth.
The estimated excess volatility associated with Crimea is conservatively estimated at 0.7 standard deviations. Using this with the estimate that increasing volatility by one standard deviation is associated with $30 billion in capital outflows, we get that the Crimean uncertainty would lead to $21 billions of capital outflows in one quarter or $84 billions in one year. If this is in the form of reduced FDI flows, we have estimated that this means that domestic investments would fall by a factor of 1.5 or $126 billions.
In this period, Russia had a GDP of $1849bn and fixed capital investments of $392bn. This means that $126 billions in reduced investments correspond to a reduction in the investments to GDP ratio of 7 percentage points (or that the investments to GDP ratio goes from around 21 percent to 14 percent).
Finally, using the estimate of 17.5 from Levine and Renelt, this implies that GDP growth would have been 1.2 percentage points higher without the estimated decline in investments to GDP.
In other words, the Crimean induced uncertainty is estimated to have led to a significant loss of growth that has to be added to all the other costs of the annexation of Crimea and continued fighting in Eastern Ukraine. Note that recent growth in Russia has been just barely above 1 percent per year, so this means that growth has been cut in half by this self-generated uncertainty.
Of course, the 1.2 percentage point estimate of lost growth is based on many model assumptions, but it provides a more sensible estimate of the cost of Crimea than we can get by looking at actual data that is a mix of many other factors that have impacted capital flows, investments and growth over this period.
Policy conclusions
The annexation of Crimea and continued fighting in Eastern Ukraine carry great costs in terms of human suffering. In addition, they also carry real costs to the Russian economy. Not least to people in Russia that see that their incomes are not growing in line with other countries in the world while the value of their rubles has been cut in half. Some of this is due to falling oil prices and other global factors that require reforms that will reorient the economy from natural resource extraction to a more diversified base of income generation. This process will take time even in the best of worlds.
However, one “reform” that can be implemented over night is to stop the fighting in Eastern Ukraine and work with Ukraine and other parties to get out of the current situation of sanctions and counter-sanctions. This would provide a much-needed boost to foreign and domestic investments required to generate high, sustainable growth to the benefit of many Russians as well as neighboring countries looking for a strong economy to do trade and business with.
References
- Becker, T, (2019), “Russia’s macroeconomy—a closer look at growth, investment, and uncertainty”, forthcoming SITE Working paper.
- Becker, T. and A. Olofsgård, (2018), “From abnormal to normal—Two tales of growth from 25 years of transition”, Economics of Transition, vol. 26, issue 4.
- Becker, T. (2016), “Russia and Oil – Out of Control”, FREE policy brief, October.
- Julio, B. and Yook, Y. (2016), ‘Policy uncertainty, irreversibility, and cross-border flows of capital’, Journal of International Economics, Vol. 103, pp. 13-26.
- Levine, R. and Renelt, D. (1992). ‘A Sensitivity Analysis of Cross-Country Growth Regressions’, American Economic Review, 82(4), pp. 942–963.
- Mileva, E. (2008), ‘The Impact of Capital Flows on Domestic Investment in Transition Economies, ECB Working Paper No. 871, February.
Disclaimer: Opinions expressed in policy briefs and other publications are those of the authors; they do not necessarily reflect those of the FREE Network and its research institutes.
Economic Growth and Putin’s Approval Ratings —The Return of the Fridge
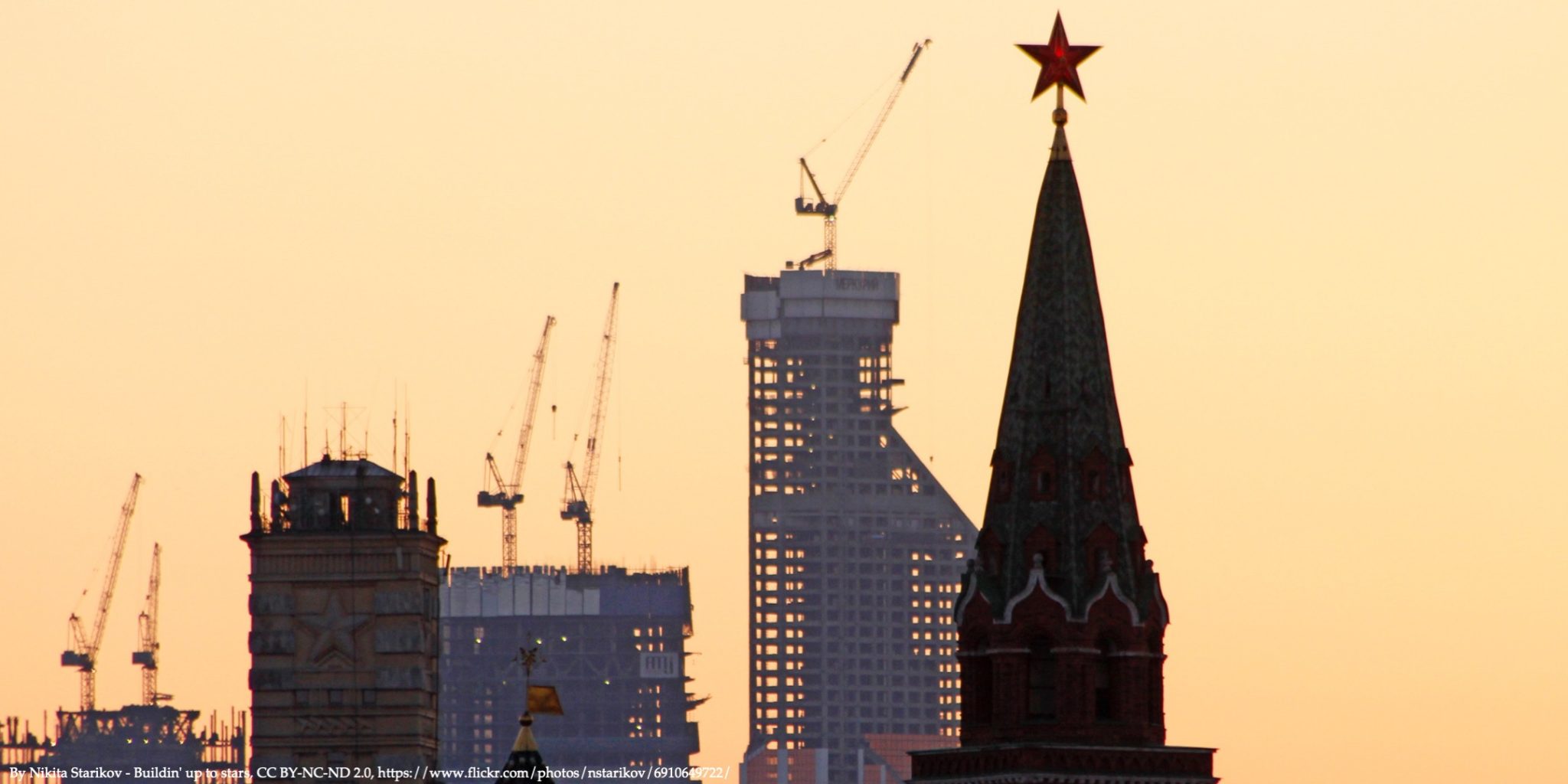
This brief discusses how the approval ratings of president Putin covaries with economic growth. In most years the relationship between approval ratings for Putin and growth looks like approval ratings for politicians in most countries so that when growth is higher, the president is more popular. Or to use an American expression “it’s the economy stupid”. The caveat in Russia is that external events at times overshadow the importance of growth to the extent that the president’s ratings stay high and can even go up despite a faltering economy. In a time of low Russian growth, this is not good news for geopolitics unless Putin can be convinced to focus on policies that generate high, sustainable growth instead of international turbulence. That said, it is clear that poor economic growth carries a political cost also in Russia. The only sustainable way of maintaining high approval ratings for the president is by fostering economic growth since in the contest between “the TV and the fridge”, the fridge will eventually win.
Russia is a complex country and culture. Instead of the simple American saying “it’s the economy stupid”, Russians talk about “the TV vs the fridge”. This translates into that concerns about the economic situation can be made irrelevant by propaganda so that voters turn their eyes away from the half-empty fridge to follow how Russia’s armed forces fight the enemy in foreign countries.
The propaganda messages have of course varied over the years, but it seems that external enemies that threaten the nation are at the heart of many of the messages. This theme in propaganda is of course not unique to Russia, but it seems to carry more weight in Russia than in other countries.
The observation that propaganda is used and that it seems to work to a relatively large extent at times can lead to the conclusion that “it’s not the economy stupid” when it comes to approval ratings of the Russian leadership.
This observation is tempting as another piece of evidence on how Russia is different and unique, but this brief will show that in most times, it is indeed “the economy stupid” also in Russia.
Putin’s ratings and growth
The idea that Russia is different in that growth would not be important for the president’s approval rating can be justified empirically when we look at the full series of approval ratings of Putin as measured by the Levada center and corresponding quarterly growth rates going back to 1999 (Figure 1).
Instead of showing a strong positive correlation as we would expect, the correlation is negative 0.3. However, a more careful look at the observations in the scatter plot suggests that there are a few clusters of observations that create this negative correlation. In the figure, three distinct clusters are marked; first there is the period when Russia rebounded strongly from the 1998 crisis in 1999-2000, with growth rates that have not been seen before or after that time in Russia. The growth was an artefact of the previous massive decline in income in combination with a large devaluation, and later followed by oil price increases. This happened in Putin’s initial time in the highest offices when he was prime minister, interim president and then elected president. Although Putin enjoyed high ratings as a consequence, it was not in line with the extreme growth rates that were the result of events preceding his tenure and can thus be regarded as outliers.
The second cluster is related to the global financial crisis in 2008/09 when Russian growth took a major hit as oil prices collapsed and the exchange rate was not allowed to appreciate correspondingly. However, this crisis was blamed (as in many other countries) on the US and the West and did not cost Putin in terms of approval ratings.
The final cluster is related to the annexation of Crimea and ongoing involvement in the conflict in Eastern Ukraine. This period also coincides with a sharp drop in oil prices that taken together led to negative growth that then remained low for a prolonged period. We should note that before the annexation of Crimea, growth rates in 2013 were very low at 1-2 percent with approval ratings going down to 63 percent, which was an all-time low since Putin’s first year in office.
Figure 1. Ratings and growth
Source: Becker (2019)
If we purge the data from the three exceptional episodes that we have identified above, we get Figure 2. Note that the scale has not been changed from Figure 1. Now there are no observations of negative growth rates, but the distribution of growth rates is still rather spread out, going from around 1 to 9 percent growth. The spread of the growth distribution is important since it allows us to identify the relationship between growth and approval ratings more clearly.
The relationship between approval ratings and growth in Figure 2 is strongly positive with a correlation coefficient of 0.7, and in line with what we would expect in other countries. This is a quite remarkable shift from the negative correlation in Figure 1. Note that if approval ratings in 2014 had been behaving as in “normal” years, the regression line would have put them around 60 percent instead of the actual approval rating that peaked at 86 percent after the annexation of Crimea. Such is the strength of the TV.
Figure 2. Ratings and growth
Source: Becker (2019)
This is very clear evidence that Russia is a “normal” country in “normal” times, but that there are also times when other forces overshadow this normalcy.
Policy conclusions
Are there any policy conclusions that can be drawn from the stark contrast between figures 1 and 2? The answer is a very clear “yes”, both for the Russian leadership but also for the rest of the world that has economic interests and security concerns with Russia.
For the Russian president, the message is that it pays in terms of high approval ratings to generate growth and “keeping the fridge well stocked”. It is also clear that the high popularity rating that was seen after the annexation of Crimea has been followed by several years of poor growth. A forthcoming brief discusses how the increased uncertainty created by this event has led to lower capital inflows, lower domestic investments and lower growth.
Not surprisingly, the sustained low growth has started to show in terms of falling approval ratings. The polls at the end of 2018 and early 2019 (for when there is not yet data on growth rates) indicate a significant decline in approval ratings, down to 64 percent from over 80 percent at the end of 2017. This is linked to protests over pension reforms, but they in turn are a result of lower government revenues in an economy that lacks growth.
In other words, if growth does not return before the propaganda loses its appeal, this will eventually result in falling approval ratings for the president, which is what we are seeing now.
There are potentially also some policy conclusions for Russia’s foreign investors, trading partners and neighbors. When growth in Russia is low and no credible reform programs are on the horizon, expect external actions that take the attention away from poor economic performance while increasing the level of uncertainty both in Russia and abroad.
For the more pro-active external actors, finding ways to support Russia’s return to growth through dialogue on real economic reforms could perhaps be both politically feasible and of mutual interest to Russia and the West. There are clearly some geopolitical issues that may interfere with this process, but it should still remain high on the wish list of regular people in Russia and elsewhere. Let the fridge rule!
References
- Becker, T., 2019. “Russia’s macroeconomy—a closer look at growth, investment, and uncertainty”, forthcoming SITE Working Paper.
Disclaimer: Opinions expressed in policy briefs and other publications are those of the authors; they do not necessarily reflect those of the FREE Network and its research institutes.
Losers and Winners of Russian Countersanctions: A welfare analysis
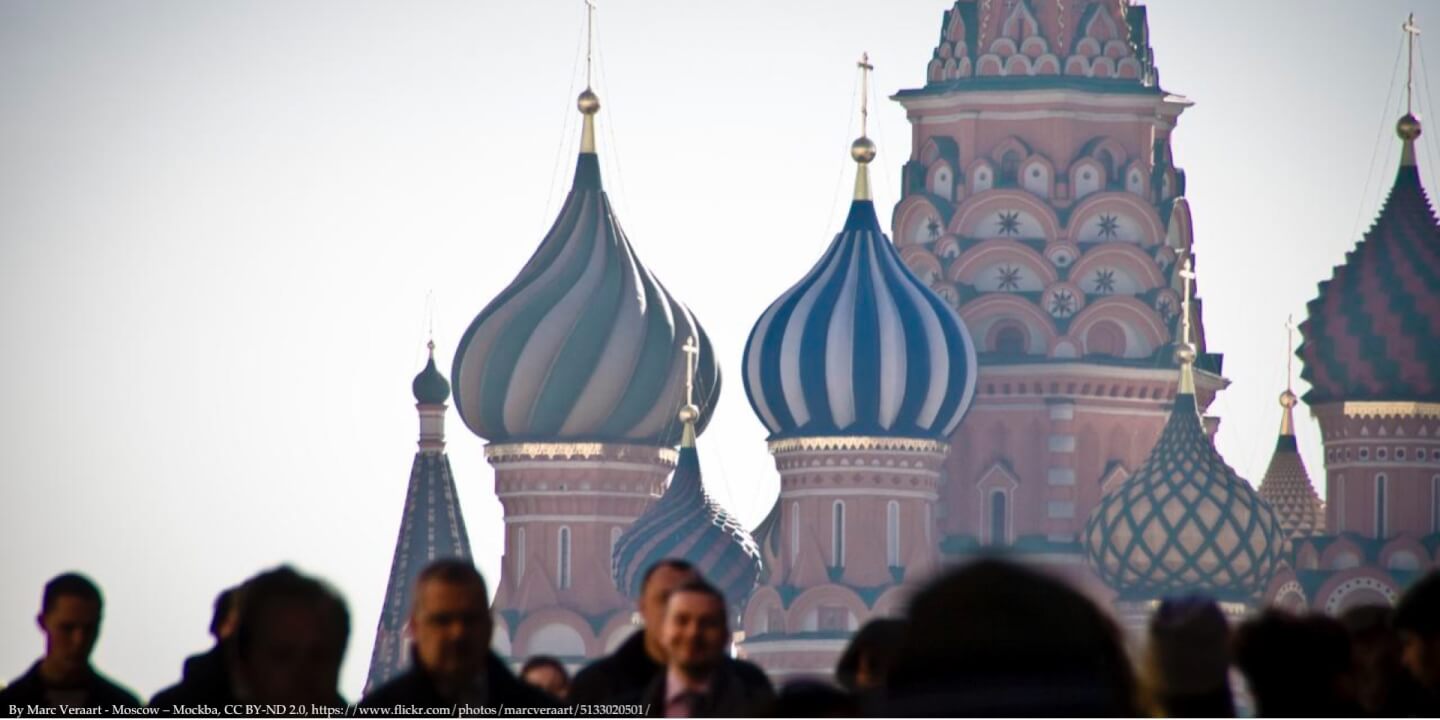
In this brief we provide a quantitative assessment of the consequences of countersanctions introduced by the Russian government in 2014 in response to sectoral restrictive measures initiated by a number of developed countries. Commodity groups that fell under countersanctions included meat, fish, dairy products, fruit and vegetables. By applying a basic partial equilibrium analysis to data from several sources, including Rosstat, Euromonitor, UN Comtrade, industry reviews etc., we obtain that total consumers’ loss due to countersanctions amounts to 288 bn Rub or 2000 rubles per year for each Russian citizen. Producers capture 63% of this amount, importers 26%, while deadweight loss amounts to 10%. 30% of the transfer from Russian consumers toward importers was acquired by Belarus. The gain of Belarusian importers of cheese is especially impressive – 83% of total importer’s gains on the cheese market.
In August 2014, in response to sectoral sanctions initiated by some countries against Russia, the national government issued resolution No. 778, which prohibited import of processed and raw agricultural products from the United States, the EU, Ukraine and a number of other countries (Norway, Canada, Australia, etc.).
Russian countersanctions were, in particular, imposed on meat, fish, dairy products, fruit and vegetables. Later the list of counter sanctioned goods was edited: inputs for the production of baby food and medicines have been deleted from the ban list, while new items were added. Salt was added to the list in November 2016 and animal fats in October 2017.
The popular idea behind the countersanctions was to limit market access for countries, which supported sectoral sanctions. The other rhetoric of the countersanctions was to support domestic producers via trade restrictions, or by other words – import substitution.
We apply a basic partial equilibrium analysis in order to evaluate the effect of countersanctions on the welfare of main stakeholders – consumers, producers and importers. The overall results are in line with general microeconomic consequences of trade restrictions in a small open economy, that is, we observe a decline in consumer surplus, increase in producer surplus and redistribution across importers. Perhaps, even more interestingly, we are able to provide a numerical assessment of redistribution effects between Russian consumers and producers, on the one hand, and among importers from different countries, on the other.
Partial equilibrium welfare analysis
We apply a framework of the classical analysis of import tariff increases to Russian countersanctions. Countersanctions resulted in increased domestic prices, declining consumption and increased domestic production. Given the increase in prices and declined volumes of consumption, we evaluate the losses by consumers as a decline in consumer surplus. Respectively, given the increase in prices and increase in domestic output we identify the producers gains as an increase in producer surplus. The only difference with a classical analysis is the lack of increase in government revenues. In this case increases in domestic prices were driven by restrictions on trade with historical partners which were substituted by more costly producers. Given the changes in the composition of importers after sanctions, we identify countries which lost and gained access to the Russian market. We use changes in volumes of trade as a measure of respective gains and losses. Figure 1 presents all relevant concepts.
In order to measure all relevant welfare changes, we rely on consumption, production and price data from Rosstat and Euromonitor, trade data from the UN Comtrade database. We use data for 2013 as a benchmark before countersanctions and compare it to 2016. The measures of own price elasticities of Russian demand and supply were taken from the literature. We use real price (in terms of 2013 prices) and volume information for consumption and supply in 2016 as the resulting points on the supply (point C) and demand (point A) curves as shown on Figure 1. Then we restore the consumption and production points on these curves (points F and B) as they would have been in 2013 given the own price elasticities of demand and supply and price level as of 2013.
Figure 1. Visualization of deadweight losses, consumer and producer surplus changes
Welfare analysis
Data
We consider 12 commodity groups that were included in 2014 in the countersanctions list: pork, cheese, poultry, apples, beef, tomatoes, processed meat, fromage frais, butter, oranges, condensed milk, grapes, cream, sour milk products, milk, and bananas.
Prices and volumes information are taken from Rosstat official statistics, which in a few cases were adjusted by data from Euromonitor. Import values were obtained from the UN Comtrade database. The summary of the original data and results of welfare analyses are reported in table 1. Below we discuss in details the situation in three markets – beef, apples and cheese.
Table 1. Summary table of the welfare effects of countersanctions
Group | Price (RUR per kg, 2013) | Production (thous. tons) | Consumption (thous. tons) | Elasticity | Consumer losses, RUR mn | Producer surplus, RUR mn | Deadweight loss, RUR mn | Importer gains, RUR mn | ||||
2016 | 2013 | 2016 | 2013 | 2016 | 2013 | demand | supply | |||||
Beef | 376 | 357 | 238 | 240 | 600 | 897 | -0.78 | 0.1 | 11311 | 4388 | 234 | 6690 |
Poultry | 109 | 108 | 4468 | 3610 | 4577 | 4084 | -0.78 | 0.45 | 3263 | 3173 | 13 | 77 |
Pork | 286 | 289 | 2042 | 1299 | 2282 | 1919 | -0.78 | 0.2 | -7167 | -6447 | 38 | -757 |
Milk | 55 | 47 | 5540 | 5386 | 5704 | 5595 | -0.93 | 0.3 | 48234 | 42507 | 4443 | 1284 |
Butter | 343 | 271 | 251 | 225 | 340 | 340 | -0.93 | 0.18 | 27468 | 17680 | 3370 | 6419 |
Cheese | 358 | 283 | 605 | 435 | 748 | 764 | -0.93 | 0.28 | 63493 | 44259 | 8437 | 10797 |
Fromage frais | 233 | 190 | 407 | 371 | 456 | 457 | -0.93 | 0.3 | 21803 | 17104 | 2600 | 2099 |
Apples | 84 | 70 | 324 | 313 | 986 | 1665 | -0.85 | 0.1 | 15225 | 4562 | 1238 | 9425 |
Bananas | 61 | 47 | 0 | 0 | 1141 | 1165 | -0.9 | 0.1 | 18967 | 0 | 2315 | 16652 |
Oranges | 65 | 59 | 0 | 0 | 932 | 1059 | -0.9 | 0.1 | 6054 | 0 | 272 | 5782 |
Grapes | 175 | 131 | 174 | 101 | 366 | 459 | -0.85 | 0.1 | 18312 | 7527 | 2351 | 8435 |
Tomatoes | 82 | 65 | 1130 | 863 | 1583 | 1718 | -0.97 | 0.1 | 28824 | 18177 | 3290 | 7357 |
Data sources: Rosstat, Euromonitor, UN COMTRADE
Bold figures were used to mark the commodity groups with a noticeable consumption growth in 2013-2016, italic figures – for those with consumption decrease, and underlined – for groups where consumption changed insignificantly during the period.
Beef
The Russian beef market experienced a drastic decrease in consumption during two years under countersanctions. In 2013 constant prices, the average real of 1 kg of beef increased by 5.3% from 357 Rub/kg in 2013 up to 376 Rub/kg in 2016. Domestic output decreased by 0.8% and to 238 thousand tons in 2016 from 240 in 2013. Domestic consumption decreased by 33.1% to 600 thousand tons in 2016 from 897 in 2013. Our estimations indicate that consumer losses amount to 11.3 bn Rub or 3.5% of beef consumption in 2013; producers’ gains are 4.4 bn Rub or 1.4%; deadweight losses are estimated at 0.2 bn Rub or 0.07%; and importers’ gains equal 6.7 bn Rub or 2.1%.
Out of total 6.7 bn Rub of importers’ gains, importers from Belarus acquire the major share (88%) – 5.9 bn Rub. Importers of beef from India and Colombia gained 0.4 bn Rub (6% of total) and 0.3 bn Rub (5%) respectively. Beef importers from Mongolia gained 0.03 bn Rub, from Kazakhstan – 0.01 bn Rub. Importers of beef from Brazil, Paraguay, Australia, Uruguay, Ukraine, Lithuania, Poland, and Argentina lost market shares in over the period 2013-2016.
Cheese
Average real price for 1 kg of cheese increased by 26.5% up to 358 Rub/kg in 2016 from 283 Rub/kg in 2013, both in constant 2013 prices. Domestic output increased by 39.1% to 605 thousand tons in 2016 from 435 thous. tons in 2013. Domestic consumption decreased by 2.1% to 748 thous. tons in 2016 from 764 thous. tons in 2013. Our results indicate the following effects of countersanctions on cheese market: consumers’ losses amounted to 63.5 bn Rub or 29.4% of cheese consumption in 2013; producer’s gain is 44.3 bn Rub or 20.5%; deadweight loss is estimated at 8.4 bn Rub or 3.9%; importers’ gains equal 10.8 bn Rub or 5.0%.
Out of a total 10.8 bn Rub of importer’s gains on the cheese market, importers of cheese from Belarus acquired the major share (82.9%) – 9.0 bln Rub, importers of cheese from Argentina gained 0.5 bn Rub (4.8% of total importers’ gain), importers from Uruguay gained 0.4 bn Rub (3.9%), Swiss cheese importers gained 0.2 bn Rub, importers from Armenia – 0.2 bn Rub (1.8%). While importers of cheese from Ukraine, the Netherlands, Germany, Finland, Poland, Lithuania, France, Denmark, Italy, and Estonia lost market access over 2013-2016.
Apples
In 2013 constant prices, average real price for 1 kg of apples increased by 20.0% up to 84 Rub/kg in 2016 from 70 Rub/kg in 2013. Domestic output increased by 3.5% to 324 thous. tons in 2016 from 313 thous. tons in 2013. Domestic consumption decreased by 40.8% to 986 thous. tons in 2016 from 1665 thous. tons in 2013. According to our analysis, the effects of countersanctions on the apple market are the following: consumers’ losses amounted to 15.2 bn Rub or 13.1 of apple consumption in 2013; producer’s gain is 4.6 bn Rub or 3.0%; deadweight loss is estimated at 1.2 bn Rub or 1.1%; importers’ gains equal 9.4 bln Rub or 8.1%.
Out of a total 9.4 bn Rub of importer’s gains, importers from Serbia acquired the major share (49.7%) – 4,7 bn Rub, importers of apples from China gained 1.6 bn Rub (16.7% of total importers’ gains), those importing from Macedonia gained 0.8 bn Rub (8.4%), from Azerbaijan 0.6 bn Rub (6.0%), and from South Africa 0.4 bn Rub (4.5% of total importers’ gains). While importers of apples from Poland, Italy, Belgium, and France lost market access.
Overall effects for 12 commodity groups
We calculated the welfare effects for 12 commodity groups: beef, poultry, milk, cheese, cottage cheese, ton butter, dairy products, apples, bananas, oranges, grapes and tomatoes.
Total consumers’ loss due to countersanctions amounts to 288 bn Rub, producers gain 63% out of this amount (182 bn Rub), 26% of total consumers’ loss is redistributed to importers (75 bn Rub), deadweight losses amount to 10% (31 bn Rub).
Distribution of importers’ gains
Belarus is the major beneficiary of Russians countersanctions: its exporters gain 29.4 bn Rub (38%), Ecuador’s exporters are in the second place with 16.4 bln Rub (21). Exporters from Serbia gained 5.1 bn Rub (7%).
Conclusion
There is no doubt that countersanctions were paid out of the pockets of Russian consumers: our estimation of total consumer losses amounts to 288 billion rubles, i.e. each Russian citizen paid 2000 rubles per year. Out of this sum, Russian producers received 144 billion rubles, i.e. transfer from Russian consumers to producers equals 1260 rubles per person per year. Among Russian sectors, major gains and associated increases in production happened in pork industries (50%), poultry (20%), dairy products (10-30%), fruit and vegetables (10-50%).
The transfer from Russian consumers toward importers from non-sanctioned countries equals 75 billion rubles a year (520 rubles per person per year), out of which 30% was acquired by Belarusian importers. Countersanctions lead to deadweight losses in the efficiency of Russian economy equal to 31 billion rubles or 215 rubles per person per year.
Disclaimer: Opinions expressed in policy briefs and other publications are those of the authors; they do not necessarily reflect those of the FREE Network and its research institutes.
Conflict, Minorities and Well-Being
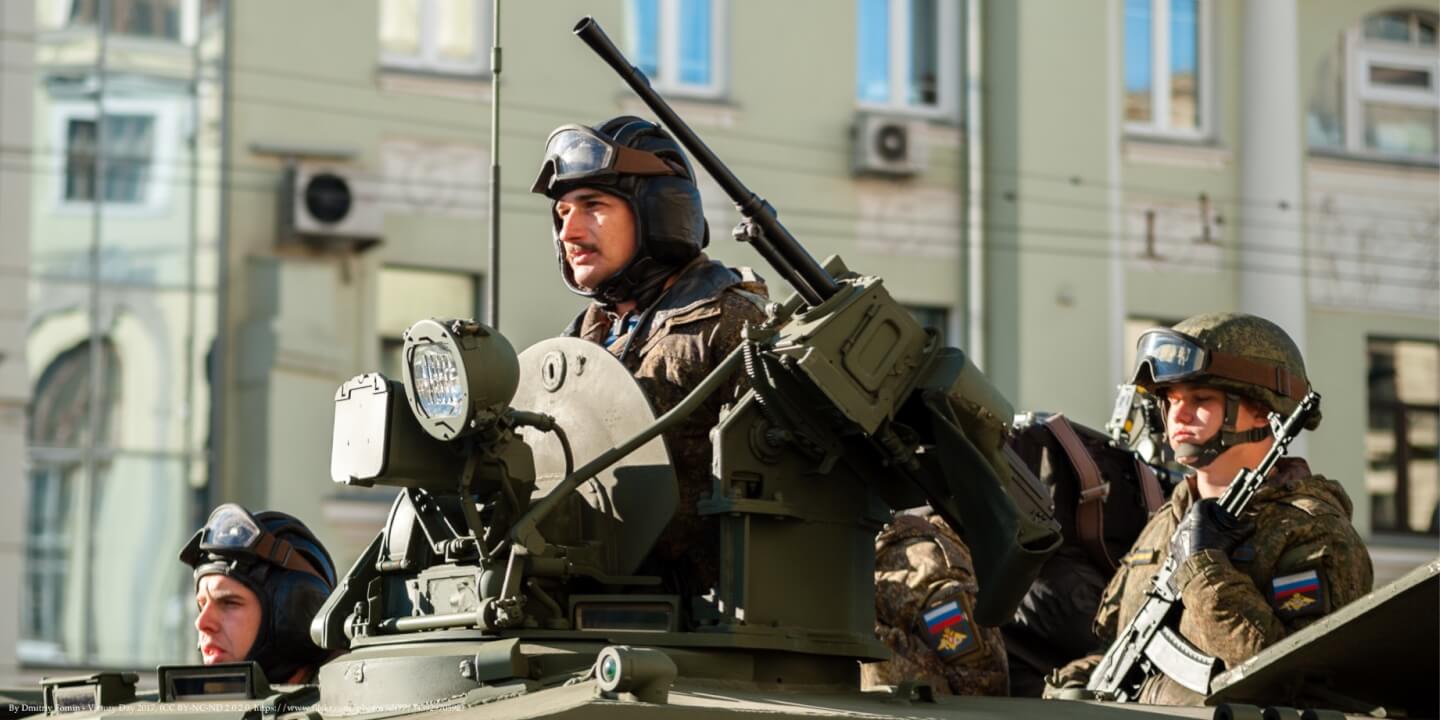
We assess the effect of the Russo-Georgian conflict of 2008 and the Ukrainian-Russian conflict of 2014 on the well-being of minorities in Russia. Using the Russian Longitudinal Monitoring Survey (RLMS), we find that the well-being of Georgians in Russia suffered negatively from the 2008 Russo-Georgian conflict. In comparison, we find no general effect of the Ukrainian-Russian conflict of 2014 on the Ukrainian nationals’ happiness. However, the life satisfaction of Ukrainians who reside in the southern regions of Russia in close proximity to Ukraine is negatively affected. We also show that the negative effect of conflict is short-lived with no long-term legacy. Additionally, we analyze the spillover effect of conflict on other minorities in Russia. We find that while the well-being of non-Slavic and migrant minorities who have recently moved to Russia is negatively affected, there is no effect on local minorities who have been living in Russia for at least ten years.
Militarized conflict affects a myriad of socioeconomic outcomes, such as the level of GDP (Bove et al. 2016), household welfare (Justino 2011), generalized trust and trust in central institutions (Grosjean 2014), social capital (Guriev and Melnikov 2016), and election turnout (Coupe and Obrizan 2016). Importantly, conflict has also been found to directly affect individual well-being (Frey 2012, Welsch 2008).
However, previous research studying individual well-being in transition countries largely abstracts from heightened political instability and conflict proneness, while this has been particularly pertinent in transition countries. Examples of transition countries facing various types of conflicts are abound, such as Yugoslavia, Ukraine, Tajikistan, Russia, Armenia, Azerbaijan, Moldova, and so on. Therefore, it is imperative to explore how conflict shapes well-being in transition countries.
In a new paper (Gokmen and Yakovlev, forthcoming), we add to our understanding of well-being in transition in relation to conflict. We focus on the effect of Russo-Georgian conflict of 2008 and the Ukrainian-Russian conflict of 2014 on the well-being of minorities in Russia. The results suggest that the well-being of Georgians in Russia suffered negatively from the 2008 Russo-Georgian conflict. However, we find no general effect of the Ukrainian-Russian conflict of 2014 on the Ukrainian nationals’ happiness, while the life satisfaction of Ukrainians who reside in the southern regions of Russia in close proximity to Ukraine is negatively affected. Additionally, we analyze the spillover effect of conflict on other minorities in Russia. We find that while the well-being of non-slavic and migrant minorities who have recently moved to Russia is negatively affected, there is no effect on local minorities who have been living in Russia for at least ten years.
Data and Results
We employ the Russian Longitudinal Monitoring Survey (RLMS) which contains data on small neighborhoods where respondents live. Starting from 1992, the RLMS provides nationally-representative annual surveys that cover more than 4000 households with 10000 to 22000 individual respondents. The RLMS surveys comprise a broad set of questions, including a variety of individual demographic characteristics, health status, and well-being. Our study utilizes rounds 9 through 24 of the RLMS from 2000 to 2015.
In this survey, we identify minorities with the question of “What nationality do you consider yourself?” Accordingly, anybody who answers this question with a non-Russian nationality is assigned to that minority group.
We employ three measures of well-being. Our main outcome variable is “life satisfaction.” The life satisfaction question is as follows: “To what extent are you satisfied with your life in general at the present time?”, and evaluated on a 1-5 scale from not at all satisfied to fully satisfied. Additionally, we use “job satisfaction” and “health evaluation” as outcomes of well-being.
Our results suggest that our primary indicator of well-being, life satisfaction, for Georgian nationals has gone down in the Russo-Georgian conflict year of 2008 compared to the Russian majority (see Figure 1). The magnitude of the drop in life satisfaction is about 39 percent of the mean life satisfaction. Our estimates for the other two well-being indicators, job satisfaction and health evaluation, also indicate a dip in the conflict year of 2008. Lastly, our estimates show that the negative impact of the conflict does not last long. Although there is a reduction in the well-being of Georgians both on impact in 2008 and in the immediate aftermath in 2009, the rest of the period until 2015 is no different from the pre-2008 period.
Figure 1. Life Satisfaction of Georgian Nationals in Russia
Source: Authors’ own construction based on RLMS data and diff-in-diff estimates.
Furthermore, when we investigate the effect of the Ukrainian-Russian conflict of 2014, we find no negative effect on the life satisfaction of Ukrainians. One explanation for why the happiness of Ukrainians in Russia does not seem to be negatively affected in 2014 is that the degree of integration of Ukrainians into the Russian society is much stronger than the degree of integration of Georgians. On the other hand, our heterogeneity analysis reveals that in the southern parts of Russia closer to the Ukrainian border, where there are more Ukrainians who have ties to Ukraine, Ukrainian nationals are differentially more negatively affected by the 2014 conflict. The differential reduction in the happiness of Ukrainians is about 19 percent of the mean life satisfaction.
Moreover, we also look into whether there is any spillover effects of the Russo-Georgian and the Ukrainian-Russian conflicts on the well-being of other minorities. We first carry out a simple exercise on non-Slavic minorities of Russia. We pick the sample of non-Slavic ex-USSR nationals that are similar to Georgians in their somatic characteristics, such as hair color and complexion. This group of people include the nationals of Azerbaijan, Kazakhstan, Uzbekistan, Kyrgyzstan, Turkmenistan and Tajikistan. We treat this group as “the countries with predominantly non-Slavic population” as their predominant populations are somatically different from the majority Russians, and thus, might either have been subject to discrimination or might have feared a minority backlash to themselves during the times of conflict. This conjecture finds some support below in Figure 2 in terms of violence against minorities. We observe in Figure 2 that hate crimes and murders based on nationality and race peak in 2008.
Our estimates also support the above hypothesis and propose that there is some negative effect of the 2008 conflict on non-slavic minorities’ happiness as well as their job satisfaction, whereas 2014 conflict has no effect.
Figure 2. Hate Murders in Russia over Time
Source: Sova Center
Next, we investigate the spillover effects of conflict on Migrant Minorities. Migrant minorities are minorities who have been living in their residents in Russia for less than 10 years. We conjecture that these minorities, as opposed to the minorities who have been in place for a long time, could be more susceptible to any internal or external conflict between Russia and some other minority group for fear that they themselves could also be affected. Whereas other types of longer-term resident minorities, which we call Local Minorities, are probably less vulnerable since they have had more time to establish their networks, job security, and most likely also have Russian citizenship. Our estimates back up the above conjecture and demonstrate that migrant minorities suffer negatively from the spillover effects of the 2008 conflict onto their well-being captured by any of the three measures, and not from the 2014 conflict, whereas there is no negative impact on local minorities.
Conclusion
In this paper, instead of focusing on the direct impact of conflict on happiness in war-torn areas, we contribute to the discussion on conflict and well-being by scrutinizing the well-being of people whose country of origin experiences conflict, but they themselves are not in the war zone. Additionally, we show that some other minority groups also suffer from such negative spillovers of conflict. Being aware of such negative indirect effects of conflict on well-being is essential for policy makers, politicians and researchers. Most policy analyses ignore such indirect costs of conflict, and this study highlights the bleak fact that the cost of conflict on well-being is probably larger than it has been previously estimated.
References
- Bove, V.; L. Elia; and R. P. Smith, 2016. “On the heterogeneous consequences of civil war,” Oxford Economic Papers.
- Coupe, T.; and M. Obrizan, 2016. “Violence and political outcomes in Ukraine: Evidence from Sloviansk and Kramatorsk”, Journal of Comparative Economics, 44, 201-212.
- Frey, B. S., 2012. “Well-being and war”, International Review of Economics, 59, 363-375.
- Gokmen, Gunes; and Evgeny Yakovlev, forthcoming. “War and Well-Being in Transition: Evidence from Two Natural Experiments”, Journal of Comparative Economics.
- Grosjean, P., 2014. “Conflict and social and political preferences: Evidence from World War II and civil conflict in 35 European countries” Comparative Economic Studies, 56, 424-451.
- Guriev, S.; and N. Melnikov, 2016. “War, inflation, and social capital,” American Economic Review: Papers & Proceedings, 106, 230-35.
- Justino, P., 2011. “The impact of armed civil conflict on household welfare and policy,” IDS Working Papers.
- Welsch, H., 2008. “The social costs of civil conflict: Evidence from surveys of happiness” Kyklos, 61, 320-340.
Disclaimer: Opinions expressed in policy briefs and other publications are those of the authors; they do not necessarily reflect those of the FREE Network and its research institutes.
Understanding Currents in the Contesting Information Spheres
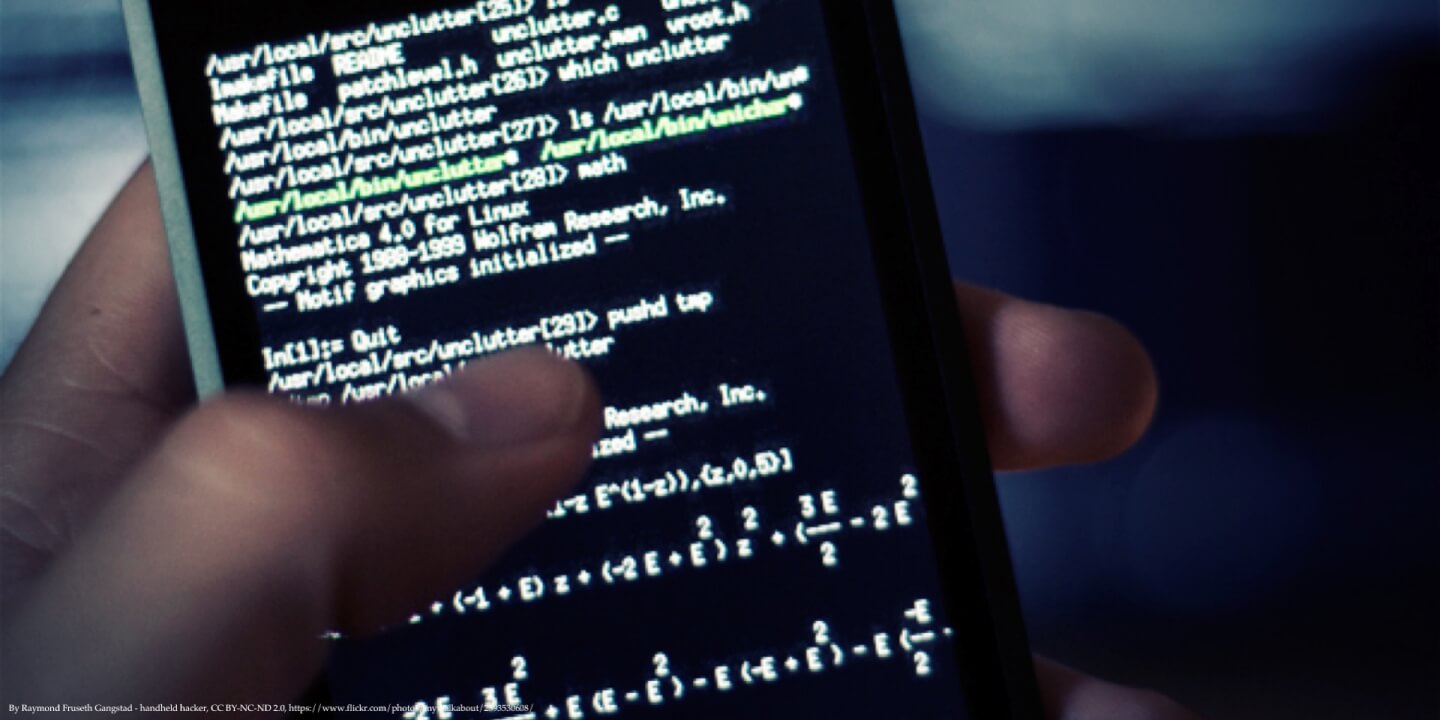
Computers and internet merely added new forms to age-old forms of propaganda. Its general purpose is, as it always has been, dualistic: to shape citizens’ image of their own country, and to streamline their views of foreign partners, competitors or enemies. Studies on information wars are often one-dimensional, i.e. presenting only actions directed against one’s own state. New Russian textbooks on information wars have a more complex approach and present long historical retrospective overviews.
Reports on disinformation campaigns are nowadays regular in the information sphere in Sweden, as in the West in general. The changes of today’s propaganda compared to classic stereotypes of the Cold War confrontations seem obvious. However, many debates on how to counter a feared information war or fake news campaigns apparently lack a long-term historical perspective. Therefore, they appear unnecessarily alarmist and might even miss their claimed purpose – to promote a sound political debate on domestic and international affairs.
Trends in Swedish information spheres – a retrospective overview
From time to time, a dominant political climate and consensus is challenged. During the prosperous 1950s, Sweden formed a self-image of the “golden middle way” between capitalism and socialism. Many aspects of this self-image were indeed partly myths. A Swedish author, Göran Palm, happened to be one of the succinct observers to challenge our prejudiced visions. His books “An unjust reflection” and “Indoctrination in Sweden” reached a wide audience and forced many to reconsider our achievements as a welfare state. Gunnar Fredriksson, editor of a Social-Democratic newspaper, alerted readers to the intricacies of “the politicians’ language” as a means to distort realities or evoke positive or negative emotions.
These books from the late 1960s were milestones for heightening the public awareness of mass media manipulation. A similar trend and radical change of Sweden’s self-image is taking place today. Until recently, the predominant view has been that Sweden represents a successful experience in forming a multicultural society, despite a few obvious crisis phenomena.
However, an awareness concerning the stress on the social fabric has spread from outsiders in the political scene towards mainstream parties. One example can highlight how changes have occurred. In January 2017, the Swedish journalist Katerina Janouch was scolded for an interview on Czech television, in which she inter alia stated her own personal view of the many problems that Sweden definitely is confronted with. After a vivid debate with harsh arguments involving even high-ranking politicians over her apparently controversial statements, she wrote a diary-like book “The Image of Sweden”. On a micro level, this fascinating personal experience succinctly shows how the image of Sweden changed over the last year, what has been accepted and what is still hotly debated concerning economics, migration and social problems.
Picture 1. “Bilden av Sverige” Book Cover
Over a short period, new political trends appeared. The political agenda has changed; serious debates treat formerly taboo topics. This is essentially because objective challenges to the economic stability, social fabric and cohesion cannot be ignored.
Even more noteworthy is, that given the outcome of the US presidential election campaign and the Brexit plebiscite of 2016, in particular the alleged role of outsiders’, supposedly decisive, involvement in these political events, Sweden has revitalized its organs on countering foreign political propaganda, which had been inactive after the Cold War era. Leading newspapers jointly with radio and TV intend to cooperate in order to thwart any attempts in 2018 to covertly interfere or overtly influence the upcoming parliamentary elections in September. Alerts against supposed disinformation campaigns by Russian mass media were at the center-stage of an annual defense policy conference in Sälen. The previous attempts to describe and analyze the supposed Russian information war efforts towards Sweden as presented hitherto seem, in my view, to lack in source collection from Russian mass media and blogospheres. They merely illustrate rather than form a structured picture of the Russian information spheres as a multiform complex.
Contests between the information spheres in Russia and the West
Therefore, as the Swedish proverb goes, “let’s turn the keg” and try to see things in a new perspective, by turning our usual modes of thought and preconceptions upside-down. A broad awareness on state propaganda in Russia, in the past as well as at present, can deepen our understanding of ongoing information wars. How does a Russian student in political sciences become aware of the formations of their nation’s self-image, as well as of foreign propaganda against their country? How do Russian scholars analyze their recent conflicts with neighboring states? What can they tell us of the general awareness concerning information warfare in the Russian public?
Three Russian historians, Viktor Barabash, Gennadii Bordiugov and Elena Kotelenets, all active in AIRO-XXI about which you can read more of here, give a broader perspective on how state propaganda has changed since the early 20th century till our times. They illustrate how countries at war, starting during World War I, directed propaganda to mass armies with, in general, literate soldiers and by that tried to influence the enemy’s morale. They evaluate how effective various forms of propaganda were, given the new technologies radio and TV during the Second World War and the Cold War eras.
After several in-depth chapters on the technological changes in the information era, on the cyber technological advances that have radically transformed traditional espionage, they finally describe how the information wars were carried out in Russia’s conflicts since 2000 (South Ossetia in 2008, Ukraine during the “Orange Revolution” and “Euro-Maidan”). Particular emphasis is devoted to how the conflicting parties formed their propaganda to their own population, on the one hand, and versus the opposing state, on the other hand.
Picture 2. ”Gosudarstvennaia propaganda i informatsionnye voiny” Book Cover
It is striking that in contrast to the Russian textbook by Barabash, Bordiugov and Kotelenets, very few analysts in Sweden have managed to present the contemporary information wars as a two-sided conflict; with two sides mutually intertwined in their mass media and social media strivings. Instead, information warfare is described as originating solely from more or less sophisticated “troll factories” in various locations in Russia. A couple of obviously forged “documents” ascribed to Swedish political leaders are sometimes referred to, although their actual effects have been nil.
In Sweden, as well as in the West in general, much has been stated on the real or imagined disinformation campaigns launched by Russia. Sometimes, they are said to direct public opinion in other states or even to influence the electorate (USA, United Kingdom). The role of relatively peripheral news agencies like RT (Russia Today) or Sputnik have seen their role amplified beyond reasonable belief. A further simplification is to reduce any Russian interpretation of events as a piece of falsification (fake news). Warnings of “Putin’s narrative” or “Russian Television fake stories” are common in mass media. In comparison, students of the Barabash textbook must undertake textual analyses of conflicting Russian and foreign opinions.
If one does not know history, you are likely to repeat its mistakes – so goes the proverb. Just as likely is the case where one repeat past generations’ mistakes because you are leaning on the mythology surrounding many events in your country’s past.
Minister of Culture Vladimir Medinskii has carried out a broad research project on the shifting images of Russia in the West, from eldest time when written sources by travelers are available. Although other historians criticized his original thesis on this subject for certain methodological flaws, there is no doubt that Medinskii accomplished a great feat as a popularizer of intricate phases in Russia’s history.
One book concerns the new historiography of the 1939–45 war on the Eastern Front. Since the late 1980s, many formerly taboo topics concerning the war were studied based on formerly secret archives as well as on interviews with veterans. In his book on the Great Patriotic War, Medinskii carefully unravels old myths and rejects new simplifications or distortions of battle histories.
Picture 3. “Mify o Rossii” Book Cover
Every historical nation tends to develop its own historiographical paradigm, which might be more or less objective and in conformity with general interpretations in other nations. However, just as often one nation’s image of their neighbors, former enemies or partners may differ substantially; thus are created the stereotypes of “the others”. In his grand comparative survey of Russia from the 12th century to the present, Medinskii provides the engaged reader with a plethora of examples of distortions of Russia’s history, created not only by foreign observers but also by ideologically motivated compatriots. Many legends on “eternal traits” in Russia are challenged. A Western reader of Medinskii’s book is bound to reflect on the various measures by which his or her country is evaluated in comparison with Russia.
In conclusion, the information contests or wars are only one element in the wider concept of cyber and hybrid wars. Observing our Swedish debate on the nefarious effects of alleged Russian disinformation, the absence of self-awareness is remarkable on how our own image of Russia (in our mass media and in the public opinion) is in itself the unconscious product of a pre-war attitude (sometimes alluded to as our age-long Russia-fear /Rysskräck/).
On the contrary, the legacy of the Soviet epoch has apparently raised the cultural curiosity among the Russian public. Mass media and publishing companies created a multidimensional panorama of their country’s past. The concerned Russian readers seem fairly well aware of politicization of historical issues and international affairs. Not for nothing do they often get substantial “food for thought” from the foreign news media translations, provided online by the InoSmi.ru site; a translation bureau, which took over the task of the Soviet-era magazine “Za Rubezhom”, and which lends its commentary fields open for anyone to comment. Even a cursory survey of commentary fields reveals their spontaneous character, rather than something created by Kremlin’s purported “troll armies”.
It goes without saying that a general and highly sophisticated awareness of overt or covert forms of meddling by a foreign state in the political process of any country must be welcomed and promoted. However, it is an open question how successful certain organized counter-disinformation strategies will be, e.g. EU’s site EUvsDisinfo.eu, NATO’s East StratCom Task Force or the Swedish joint public radio and TV with leading newspapers to “combat fake news”. Leaving much broader fields in the information sphere for freer opinion making in mainstream media as well as in the blog sphere might prove to be a sounder path towards dialogues, debates and mutual understanding.
References
- Barabash, V. & G. Bordiugov & E, Kotelenets, Gosudarstvennaia propaganda i informatsionnye voiny (2015), AIRO-XXI
- Fredriksson, G., Det politiska språket (1966 and later editions), Tiden.
- Janouch, K., Bilden av Sverige (2017), Palm Publishing.
- Palm, G., En orättvis betraktelse, (1966) and Indoktrineringen i Sverige (1968), PAN/Norstedts
- Medinskii, V., Voina: Mify SSSR, 1939 – 1945 (2011) and Mify o Rossii (2015), Abris/OLMA
Disclaimer: Opinions expressed in policy briefs and other publications are those of the authors; they do not necessarily reflect those of the FREE Network and its research institutes.
Is There a Dutch Disease in Russian Regions?
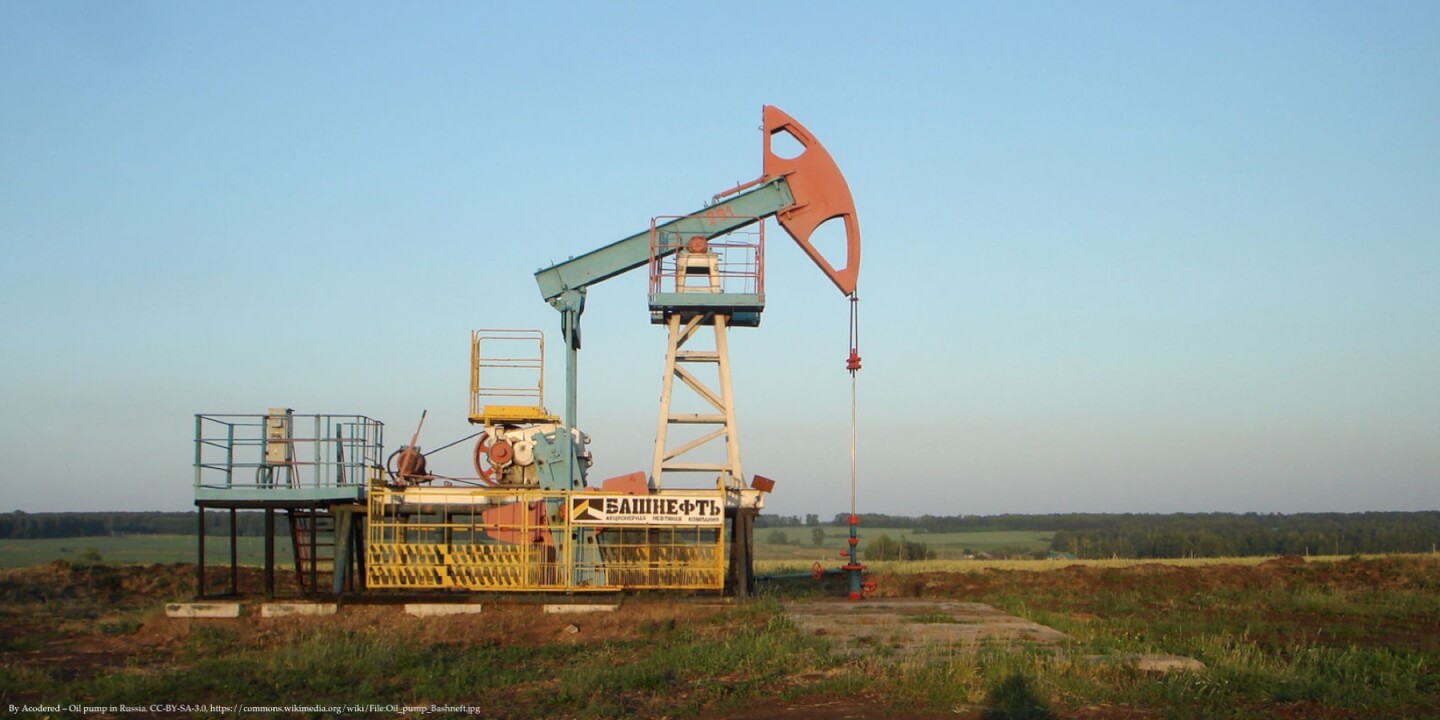
The low economic diversification in Russia is commonly blamed on the abundance of energy resources. This brief summarizes the results of our research that investigates the presence of Dutch disease effects across Russian regions. We compare manufacturing subsectors with different sensitivity to the availability of natural resources across Russian regions with varying natural resource endowments. We find no evidence of differential deindustrialization across subsectors, thereby offering no support for a Dutch disease. This finding suggests that the impact of energy resources on Russian manufacturing is more likely to go through the “institutional resource curse” channel. Thereby, we argue that more efficient policies to counteract the adverse effect of resources on the Russian economy should focus on improving the institutional environment.
Russian abundance in oil and gas, and the ways it could negatively affect long-term economic performance and institutional development is not a new debate. One of the key concerns is the influence of energy resources on Russian industrial structure. Energy resources are often blamed for the low diversification of the economy, with an extensive resource sector and the dominant oil and gas export share.
In a forthcoming chapter (Le Coq, Paltseva and Volchkova), we contribute to this debate by exploring the channels through which abundance in energy resources influences the industrial structure in Russia. Our main focus is on the deindustrialization due to the expansion of the natural resource sector, the so-called ‘Dutch disease’. Specifically, we explore the impact of energy resources on the growth of manufacturing subsectors in Russian regions. Adopting a regional perspective allows us to separate the Dutch disease mechanism from the main alternative channel of the institutional ‘resource curse’. This brief summarizes our findings.
Dutch disease vs. institutional resource curse
The Dutch disease and the institutional resource curse are, perhaps, the most discussed mechanisms proposed to explain the influence of natural resources on economic performance (see e.g., earlier FREE brief by Roine and Paltseva for a review). In an economy facing a Dutch disease, a resource boom and resulting high resource prices shift production factors from manufacturing industries towards resource and non-tradable sectors. As a result, a country experiencing a resource boom would end up with a slow-growing manufacturing and an under-diversified economic structure. Since the manufacturing sector is often the main driver of economic growth, the economic development may be delayed. If, instead, an economy is suffering from the institutional ‘resource curse’, it is the interplay of weak institutions and adverse incentives created by resource rents that leads to a slow growth of manufacturing and delayed development.
Importantly, offsetting the potential negative impact of these two channels requires different policy interventions. In the case of a Dutch disease, a state can rely on direct industrial policy mechanisms targeted towards increasing the competitiveness of the manufacturing sector and isolating it from the effect of booming resource prices. For example, it can use subsidies or targeted trade policy instruments, or channel money from increased resource prices out of the economy through reserve fund investments abroad.
In the case of an institutional resource curse, on the other hand, resource rents and weak institutions may undermine and disrupt the effect of such policies. In this case, state policies should be targeted, first and foremost, towards promoting good institutions such as securing accountability and the transparency of the state, and protecting property rights. This suggests that properly understanding the channels through which resource wealth impacts the economy is necessary for choosing appropriate remedial measures.
In our analysis, we address the differential impact of energy resources in Russian regions. This regional perspective allows us to single out the Dutch disease effect, and disregard the mechanisms of a political resource curse to the extent that the relevant institutions do not differ much across regions.
Resource reallocation effect vs. spending effect
The mechanism of a Dutch disease implies two channels through which a resource boom negatively affects the manufacturing sector. First, a resource boom implies the reallocation of production factors from other sectors of economy such as manufacturing or services to the resource sector, a so-called ‘resource reallocation effect’. Second, an additional income resulting from a boom in the resource sector leads to an increase in demand for all goods and services in the economy. This increase in demand will be accommodated differently by different sectors, depending on their openness to world markets. Namely, in non-tradable sectors, isolated from international competition, there will be an increase in prices and output. This, in turn, will increase the prices on domestic factor markets. For tradable manufacturing sectors the price is determined internationally and cannot be adjusted domestically. As a result, production factors will also reallocate away from manufacturing to non-tradable sectors, a so-called “spending effect”.
The strength of either effect is likely to be different across different subsectors of manufacturing depending on the sectoral specificities. In particular, subsectors with higher economies of scale are likely to be more affected by the outflow of factors towards the resource sector through the “resource reallocation effect”. Similarly, subsectors that are more open to international trade are likely to be affected by the “spending effect”.
These observations give raise to our empirical strategy: we access differences in growth of regional manufacturing subsectors with different sensitivity to the availability of energy resources, where sensitivity reflects economies of scale, for the first mechanism, and openness to the world market, for the second mechanism. In other words, we test whether manufacturing subsectors with higher economies of scale (or openness) grow slower than subsectors with lower economies of scale (or openness) in regions rich in energy resources, as compared to the regions poor in energy resources. Observing differential deindustrialization, depending on the industry’s exposure to the tested mechanism, would offer support to the presence of a Dutch disease.
Note that the validity of our empirical strategy relies on the fact that there is high variation in resource abundancy and structure of the manufacturing sectors across Russian regions (as illustrated by Figures 1 and 2).
Figure 1. Geographical distribution of fuel extractions relative to gross regional product; 2014, percent.
Source: Authors’ calculation based on Rosstat data. Note: Figures for regions exclude contribution of autonomous okrugs where applicable.
Figure 2. Regional diversity in manufacturing structure, 2014.
Data and results
Our empirical investigation covers the period 2006—2014. The data on manufacturing subsector growth and regional energy resource abundancy come from Rosstat, the sensitivity measures across different manufacturing sectors are approximated based on data from Diewert and Fox (2008) (economies of scale in US manufacturing), and OECD (sectoral openness to trade).
The results of our estimation show that the differences in growth rates of manufacturing subindustries across Russian regions with varying natural resource endowments cannot be explained by the sensitivity of these subindustries to the availability of energy resources. This can be seen from Table 1, where the coefficient of interest – the one of the interaction term between the measure of sectoral sensitivity if resource abundance and regional energy resource wealth – is not significantly different from zero, no matter how we measure the sensitivity: by the returns to scale or by openness to international trade.
Table 1. Estimation of Dutch disease effect with different sensitivity measures.
Dependent variable: average annual growth index of sectoral output | ||
Sensitivity measure: Economies of scale | Sensitivity measure: Openness | |
Subsector sensitivity * Size of the fuel extraction sector in the region
|
-0.0353
(0.0873) |
0.0674
(0.0954) |
Subsector fixed effect | YES | YES |
Region fixed effect | YES | YES |
Observations | 1,185 | 1,185 |
R-squared | 0.1574 | 0.1577 |
Source: Authors’ calculations.
These results hold true if we control for differences in regional taxes, labor market conditions, and other region-specific characteristics by including regional and sectoral dummy variables, if we consider alternative measures of energy resource wealth, and if we use other, non-parametric estimation methods.
In other words, our data robustly offers no support for the presence of a Dutch disease in Russian regions.
Conclusion and policy implications
Diversification is often mentioned by the Russian government, as one of the top economic policy priorities, and the need for ‘diversification’ has been used in the political debate as an argument for an active industrial policy.
However, the policy measures that are necessary to counter the effect of abundant energy resources on diversification and, more generally, on economic development may be highly dependent on the prevailing channel through which resources affect the economy. In particular, while active industrial policy may be justified as a remedy in the case of a Dutch disease, industrial policy may well be ineffective, or even harmful, in the presence of an institutional resource curse mechanism.
In our study, we find no support for the Dutch disease effect when looking at the impact of energy resources on the growth of regional manufacturing sectors. Thereby, to counterbalance the resource curse effect on the Russian economy, we argue that it may be more efficient to improve the institutional environment than to use active government policies affecting industrial structures.
References
- Diewert, W. E and Fox, K. J. (2008) ‘On the estimation of returns to scale, technical progress and monopolistic markups’, Journal of Econometrics, 145(1-2): 174-93.
- Le Coq, C., Paltseva E., and Volchkova N., forthcoming. “Regional impacts of the Russian energy sector”, in Perspectives on the Russian economy under Putin, eds. Becker and Oxenstierna, London, Routledge.
Disclaimer: Opinions expressed in policy briefs and other publications are those of the authors; they do not necessarily reflect those of the FREE Network and its research institutes.
The Russian economy under Putin (so far)
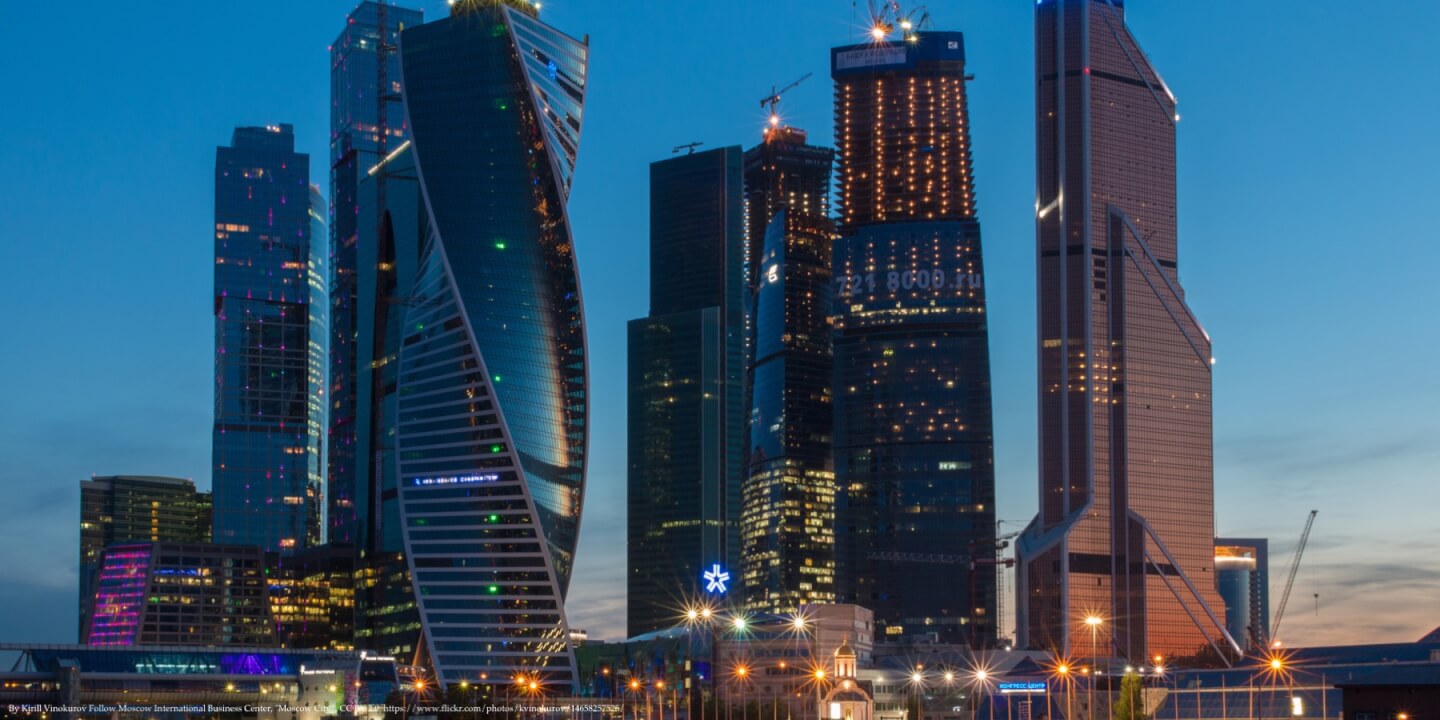
Russians are heading to the polling booths on March 18, but where will the economy head after Putin has been elected president again? This brief provides an overview of the economic progress Russia has made since 2000 as well as an economic scorecard of Putin’s first three tenures in the Kremlin and uses this to discuss what can be expected for the coming six years. Although significant growth has been achieved since 2000, all of this came in the first two tenures of Putin in the Kremlin on the back of increasing oil prices. In order to generate growth in his upcoming presidential term, Putin and his team will need to address the significant needs for reforms in the institutions that form the basis for modern market economies. Otherwise, Russia will continue to be hostage to the whims of the international oil market and eventually lose most of its exports and government revenues as the world moves towards a carbon free future. Perhaps this is beyond the scope of Putin as president, but not beyond the horizon of young Russians that will be casting their votes on Sunday and in future elections.
Let’s assume that Putin will be elected president again on March 18 (for once a very realistic assumption made by an economist). What will this mean for the Russian economy in the coming six years given what happened during his previous and current tenures in the Kremlin? To assess the future as well as to understand Putin’s power and popularity, this brief starts by looking back at the economic developments in Russia since Putin first became president.
Although many different factors enter the power and popularity function of Putin, economic developments have a special role in providing the budget constrain within which the president can operate. A higher income level means more resources to devote to any particular sector, project, voting group or power base. This is not unique to Russia, but sometimes forgotten in discussions about Russia, that often instead only focus on military power or control of the security apparatus and media. These are of course highly relevant dimensions to understand power and popularity in Russia, but so is economic development, particularly in the longer run.
Russia’s economy in the world
The economic greatness and progress of a country is usually assessed in terms of the size of the economy, how much growth that has been generated, and how well off the citizens are relative to the citizens of other countries. So, by our common indicator gross domestic product (GDP), has Russia become a greater and more powerful country since Putin first became president? Table 1 shows two things, the absolute level of GDP measured in USD at market exchange rates and the rank this gives a country in a sample of 192 countries in the world that the IMF collects data on (this brief is too short for a long discussion of the most relevant GDP measure, but GDP at market exchange rates makes sense when comparing the economic strength of countries in a global context, Becker 2017 provides a discussion of alternative measures as well). When Putin become president for the first time in 2000, the value of domestic production was estimated at $279 billion, which implied a 19th place in the world rankings of countries’ GDP. In 2016, almost three presidential terms of Putin later, Russia’s GDP had increased by 4½ times to $1281 billion and its ranking improved to 12th place in the world. This clearly is an impressive record by most standards. However, the Russian economy is still the smallest economy of the BRIC countries and corresponds to only 7 percent of the US economy in 2016. In other words, impressive progress by Russia but the country is (still) not a global superpower in the economic arena.
Table 1. Russia in the world (GDP in USD bn)
For the average Russian, income per capita is a measure more closely connected to consumption and investment opportunities or ‘welfare’. Progress in this area is also more likely to affect how individuals assess the performance of its political leaders. Of course, progress in terms of overall GDP and GDP per capita is closely linked unless something unusual is happening to population growth. Therefore, it is not surprising that GDP per capita also increased by around 4½ times between 2000 and 2016 (Table 2). This is the first order effect of the economic development in Russia, but in addition, citizens of Russia moved up from a world income rank of 92nd to 71st. This has implications when Russian’s compare themselves with other countries and can in itself provide a boost of national pride.
It also directly affects opportunities and status for Russians visiting other countries. Being at place 71 may not be fully satisfactory to many, but we should remember that due to the rather uneven income distribution in Russia, many of the people that travel abroad are far higher up on the global income ranking than what this table indicate. Nevertheless, Russia is far behind the Western and Asian high-income countries in terms of GDP per capita. And although the picture would look less severe if purchasing power parity measures are used, the basic message is the same; Russia has still a lot of catching up to do before its (average) citizens enjoy the economic standards of high-income countries.
Table 2. Russian’s in the world (GDP/capita)
The macro scorecard of Putin
So what generated the impressive 4½ times increase in income in USD terms from 2000 to 2016 and can we expect high growth during Putin’s next six years in office? The short answer to the first question is the rise in international oil prices and to the second question, we don’t know. Table 3 provides a comparison of different economic indicators for Putin’s two first terms in office compared with his current term (where GDP data ends in 2016 so the sample is cut short by a year). It is evident that the impressive growth over the full period is entirely due to the strong growth performance in the first two presidential tenures. Rather than generating growth in the most recent period, the economy has shrunk. This is explained by the evolution of international oil prices, which quadrupled in the first eight years and instead halved in the more recent period. These swings in oil prices have also been accompanied by significant shifts in foreign exchange reserves, the exchange rate, and the value of the stock market.
In Becker (2017) I discuss in more detail the importance of international oil prices in understanding the macro economic development in Russia. In particular, it is important to note that it is changes in oil prices that correlate with GDP growth and other macro variables and that the problems with predicting oil prices makes it very hard to make good predictions of Russian growth.
Table 3. A macro scorecard of Putin in office
Policy conclusions
To break the oil dependence and take control of the economic future of Russia, the president will need to implement serious institutional reforms that constitute the basis for a modern, well-functioning market economy in his next term. Otherwise, Russia will continue to be hostage to unpredictable swing in international oil prices and nobody—including the president, the central bank, the IMF and financial markets—will be able to predict where the Russian economy is heading in the next couple of years.
Figure 1. Reforms (still) needed
In the longer run, the prediction is much easier. With the world moving towards a green economy, the price of oil will see a structural decline that will rob Russia (and other oil exporters) of most of its export and government revenues. The reforms which basically every economist agree are needed are related to market institutions and Figure 1 provides a clear illustration of key reform areas. The progress during Putin’s years in office has been modest at best. Swedish institutions in 2016 have been added to the figure as a comparison and it is clear that the institutional gap between Russia and Sweden is significant. Of course, all countries are different, but Russian policy makers that are interested in reforming its economy are most welcome to Sweden for a discussion of what we have done to build our institutions.
References
- Becker, T. (2017). ‘Macroeconomic Challenges’, in Rosefielde, S., Kuboniwa, M., Mizobata, S. and Haba K. (eds.) The Unwinding of the Globalist Dream: EU, Russia and China, Singapore: World Scientific Publishing.
- Becker, T. (forthcoming), ‘Russia’s economy under Putin and its impact on the CIS region’, Chapter 2 in T. Becker and S. Oxenstierna (eds.) Perspectives on the Russian Economy under Putin, London: Routledge.
- IMF (2017), World Economic Outlook database, April 2017 edition available at http://www.imf.org/external/pubs/ft/weo/2017/01/weodata/index.aspx
- World Bank (2017), Worldwide Governance Indicators (WGI), 2017 update available at http://info.worldbank.org/governance/wgi/index.aspx#home
School Financing, Teacher Wages and Educational Outcomes in Russia
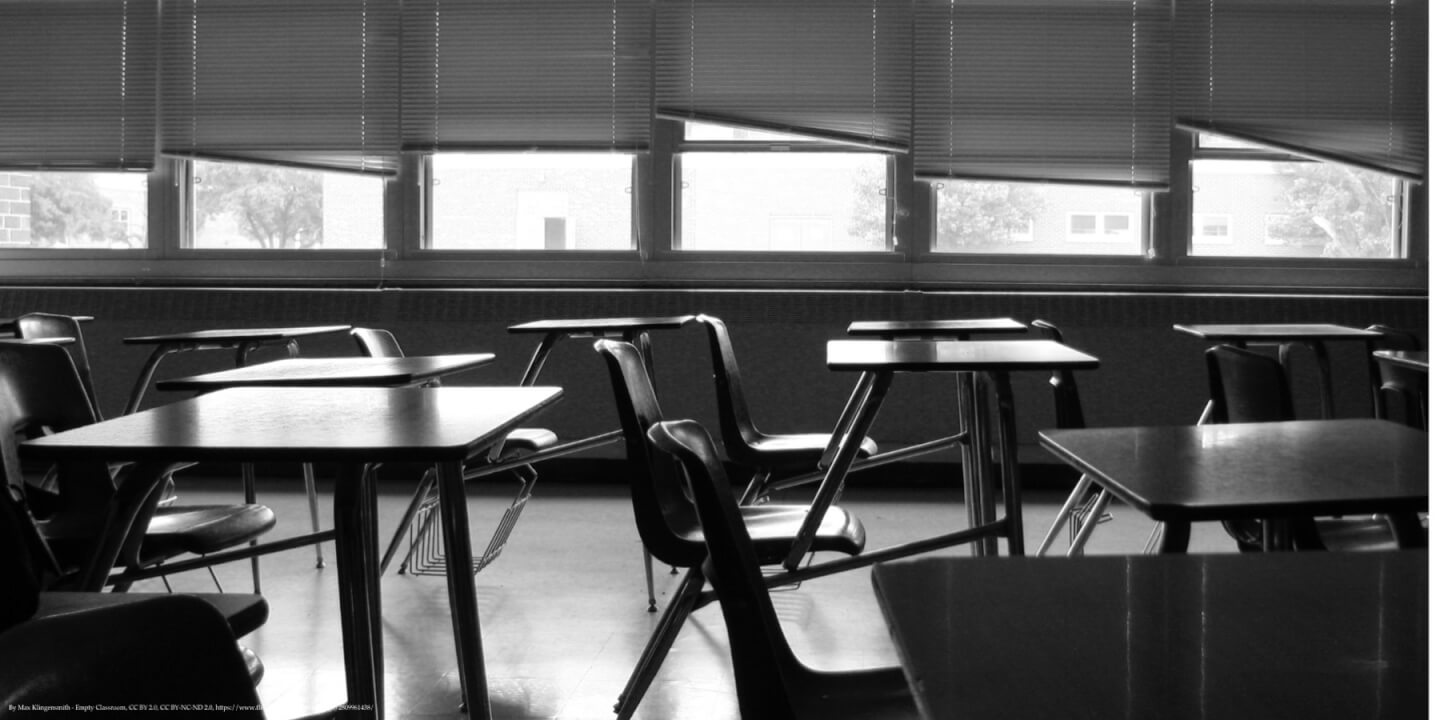
The policy proposal to increase the share of budget spent on public education implies that higher financing leads to better quality of education. This, however, is far from certain. We test and compare the effects that different levels of financial resources available to schools and relative teacher wages have on educational outcomes. Russia provides a good opportunity for testing this relationship due to its high level of regional heterogeneity. We find that increasing school financing per se does not noticeably improve educational outcomes. Only when additional financing leads to an improvement of the position of teachers in the regional wage distribution, we observe higher educational outcomes for students. We provide some tentative evidence on the possible channels of this effect.
School education is a complex and multifaceted process, and measurable educational outcomes are affected by many different factors. These may include students’ innate abilities and family resources as well as various characteristics of the school environment and teaching practices. In the literature, one of the important factors is the level of school financing provided by the government. This is also one of the key issues in the debates about the public policy in education. However, there is no consensus in the academic literature about the degree of influence of financial resources available to schools on educational outcomes.
The effect of school financing should depend on how it is spent. Since education is a human capital-intensive sector, a major part of this money is spent on teacher remuneration. Whether the size and structure of teacher pay affect the effectiveness of their work and ultimately the student outcomes is still an open question. Some studies argue that it is not absolute but that relative teacher wages matter (Loeb and Page, 2000; Britton and Propper, 2016). Hanushek et al. (2017) use cross-country data and show that the relative position of teachers in the wage distribution affects self-selection into the teaching profession in terms of skills, and that teacher skills in turn affect student outcomes.
While there are studies looking at various determinants of the quality of school education in the transition-economy context (e.g. Amini and Commander, 2012), the effect of school financial resources has not yet been studied. In Lazareva and Zakharov (2018), we exploit spatial variation in educational resources in Russia to try to answer this question. We test and compare the effects of school budget financing and relative teacher wages on educational outcomes for the period 2006–2014. We estimate these effects for two different measures of educational outcomes at different levels of school education system.
Institutional Context and Data
In Russia the system of general education covers eleven years: the first nine years are compulsory for all children, after that one can continue to high school for two more years or move into vocational education system. The school system is predominantly financed by the government and the share of private schools is very low.
In the 1990s and early 2000s, the system of general education was heavily underfinanced. Teacher remuneration was quite low compared to the average wage in the economy, and a job as a schoolteacher was not very attractive. In the mid-2000s, with the fast economic growth, the Russian government made an effort to increase school financing and to raise teacher wages. Importantly, schools are financed at the regional level, through the budgets of the regions, which results in significant cross-regional variation.
There are 85 administrative regions currently in Russia and they differ a lot in terms of economic conditions, regional budget income and expenditures. We use data on regional-level budget expenditures on general education from the Russian Treasury statistics (http://www.roskazna.ru/). In order to account for inflation and cross-regional differences in prices, we normalize the per-student amount of school budget financing by the minimum regional cost of living (as estimated by the Russian statistical office) in a particular year.
As our data show, the amount of budget financing of the general education system has been growing in real terms during 2006–2013. The average regional budget financing per student (adjusted for the differences in the cost of living across regions and years) has increased by 40% during this period. A large part of this growth occurred in 2012. In that year a presidential decree was adopted which required that teachers’ wages should be raised to the level of the average regional wage. Regions had to allocate more money for teacher wages during the following years in order to meet this target. Even after adjusting for the regional cost of living, the level of school financing differs a lot across regions throughout the period.
The amount of school financing is also significantly correlated with the gross regional product per capita, i.e. with the level of economic development of the region. We observe the largest gap in school financial resources between the small group of the richest regions (Moscow, Sankt Petersburg and resource extracting regions) and the remaining regions. Such persistent inequality in school resources may lead to unequal access to high quality education across Russian regions. This inequality is exacerbated by the fact that in less economically developed regions families have fewer resources to compensate for the underfinancing of public schools.
The structure of school expenditures in the regional budgets shows that the major part of financing (about 80 percent) is spent on remuneration of teachers and school administration. Hence, the effect of regional school expenditures on student outcomes should go through teacher wages. We use data on average regional teacher wages from Rosstat (Russian Federal State Statistics Service) and the Russian Ministry of Education. As we argued previously, it is important to test the effect of relative teacher salary. Our data show that the average regional school wage relative to the average regional wage has grown during the observation period, in particular in 2008–2009 and, at a higher rate, in 2012–2013 (due to the presidential decree mentioned above). Again, there is a significant variation among regions, which is observed throughout the period.
Empirical Results
In order to test the effect of school resources and teacher wages on educational outcomes, we use two measures of educational outcomes. First, we use the average regional score on Unified State Examination (USE). It was introduced in all Russian regions starting from 2009 and students graduating from grade 11 take the test. This is a high stakes examination as the result of this exam is accepted as entrance exams at universities throughout the country. USE in mathematics and Russian language are compulsory for all graduates of grade 11. Therefore, we will use the scores in these subjects. Note that USE scores measure educational outcomes of those students that stayed in high school after grade 9 – this is about 60 percent of the age cohort.
An alternative measure of educational outcomes is the data from PISA international educational assessment (PISA – Programme for International Student Assessment run by OECD, http://www.oecd.org/pisa/). Russia participates in PISA since 2003. We use data from waves 2006, 2009, 2012, and 2015. Students take this test at the age of 15, which means that the majority of this age cohort is in grade 9.
In our regression analysis on regional data, we additionally control for a number of regional characteristics that may be correlated with school financing or teacher wages, such as population size, share of urban population, regional poverty (share of population below the poverty line), within-region income inequality (decile coefficient), and gross regional income per capita (also adjusted for the cost of living). Since we have panel data, we use a panel fixed effects estimation method, which accounts for all unobserved time-invariant regional heterogeneity.
Our results show that the level of per-student school financing does not significantly affect USE results. At the same time, we find a significant positive effect of relative teacher wages on USE results both in math and Russian language with the lag of one to two years. We find the same results on PISA data: individual student scores in math, reading and science are significantly positively affected by the level of the relative regional teacher wages. Our results hold in instrumental variable estimation, which we conduct in order to account for potential endogeneity problems.
What are the potential channels through which relative teacher wage may affect student results? One possible channel is self-selection of teachers. When teacher wages increase relative to other jobs, being a teacher become more attractive for higher skilled individuals. Higher skilled teachers help students to achieve better educational results. We cannot directly test this channel, as we do not have data on teacher turnover in Russian schools. Besides, we observe a positive effect of relative teacher wages on student scores with a lag of just one-two years. This seems to be a too short time period for teacher turnover to have a significant effect.
Another potential channel of the observed effect is an improvement in teacher motivation or teacher morale. We can only provide some suggestive evidence for this effect. In the early and mid-2000s, when teacher pay was quite low, a significant share of teachers were considering quitting their jobs or switching to another occupation. As teacher survey data show, after the significant increase in teacher pay in 2008–2012 this share declined and teacher motivation and job satisfaction improved. Additional evidence in support of this hypothesis comes from the school-level data in the PISA 2012 survey. We estimate the effect of relative regional school wage on teacher morale (as evaluated by a school head) and find a positive and statistically significant relationship.
Conclusion
We find that increasing school financing from the regional budgets per se does not noticeably improve educational results. Only when additional financing leads to an improvement of the position of teachers in the regional wage distribution, we observe higher educational outcomes for students. The potentially interesting future direction of research is to study how not just the relative size, but also the structure of teacher wages (i.e. elements of incentive pay introduced in Russian schools) affects educational outcomes.
References
- Amini, Chiara & Commander, Simon, 2012.”Educational Scores: How does Russia Fare?” Journal of Comparative Economics, Elsevier, vol. 40(3), pages 508-527.
- Britton, Jack and Carol Propper, 2016, Teacher pay and school productivity: Exploiting wage regulation, Journal of Public Economics 133 (2016) 75–89.
- Hanushek, Eric A., Marc Piopiunik, Simon Wiederhold, 2017, The Value of Smarter Teachers: International Evidence on Teacher Cognitive Skills and Student Performance, NBER Working Paper w20727.
- Lazareva, O. and A. Zakharov, 2018, School Financing, Teacher Wages and Educational Outcomes: Evidence from the Russian School System.
- Loeb, Susanna and Marianne E. Page, 2000, Examining the Link between Teacher Wages and Student Outcomes: The Importance of Alternative Labor Market Opportunities and Non-Pecuniary Variation, the Review of Economics and Statistics 2000 82:3, 393-408.