Location: Russia
Short-Run and Long-Run Effects of Sizeable Child Subsidy: Evidence from Russia
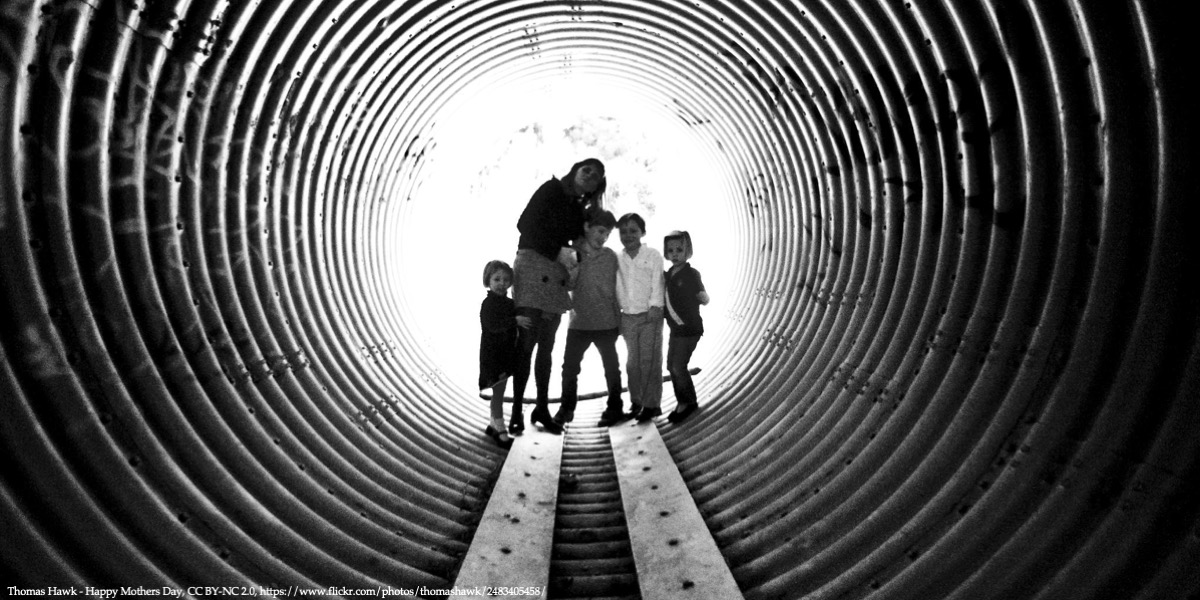
How to design the optimal pro-natalist policy is an important open question for policymakers around the world. Our paper utilizes a large-scale natural experiment aimed to increase fertility in Russia. Motivated by a decade-long decrease in fertility and population, the Russian government introduced a sequence of sizable child subsidies (called Maternity Capitals) in 2007 and 2012. We find that the Maternity Capital resulted in a significant increase in fertility both in the short run and in the long run. The subsidy is conditional and can be used mainly to buy housing. We find that fertility grew faster in regions with a shortage of housing and with a higher ratio of subsidy to housing prices. We also find that the subsidy has a substantial general equilibrium effect. It affected the housing market and family stability. Finally, we show that this government intervention comes at substantial costs.
In all European and Northern American countries the fertility is below the replacement level (United Nations, 2017). Following this concern, most of the developed countries have implemented various large scale and expensive pro-natalist policies. Yet, the effectiveness of these policies is unclear, and the design of the optimal pro-natalist policy remains a challenge.
There are several important open research questions on the evaluation of these programs. The first is whether these programs can induce fertility in the short-run and/or in the long-run horizon. Indeed, very few of these expensive and large-scale policies are proved to be an effective tool to increase fertility (Adda et al, 2017). The next set of questions deals with further evaluation of the programs: What are the characteristics of families that are affected by this policy? How costly is the policy, i.e. how much is the government paying per one birth that is induced by the policy? Finally, what are the non-fertility related effects of these policies? While most of the studies that analyze the effect of pro-natalist policies concentrate on fertility and mothers’ labor market outcomes, these, usually large-scale, policies may have important general equilibrium and multiplier effects that may affect economies both in the short run and long run (Acemoglu, 2010).
In our paper we utilize a natural experiment aimed to increase fertility in Russia to address these questions.
Motivated by a decade-long decrease in fertility and depopulation, the Russian government introduced a sizable conditional child subsidy (called Maternity Capital). The program was implemented in two waves. The first wave, the Federal Maternity Capital program, was enacted in 2007. Starting from 2007, a family that already has at least one child, and gives birth to another, becomes eligible for a one-time subsidy. Its size is approximately 10,000 dollars, which exceeds the country’s average 18-month wage and exceeds the country’s minimum wage over a 10-year period. The recipients of the subsidy can use it only on three options: on housing, the child’s education, and the mother’s pension. Four years later, at the end of 2011, Russian regional governments introduced their own regional maternity programs that give additional – on the top of the federal subsidy – money to families with new-born children.
In our paper, we document that the Maternity Capital program results in a significant increase in fertility rates both in the short run (by 10%) and in the long run (by more than 20%). This effect can be seen from both within-country analysis and from comparing the long-term growth of fertility rates in Russia with Eastern and Central European countries that face similar economic conditions and had similar pre-reform fertility trends. Like Russia, Eastern European countries experienced a drop in fertility rates right after the collapse of the Soviet Union and had similar trends in fertility up until 2007. Our results show that while having similar trends in fertility before 2007, afterward Russia significantly surpassed all the countries from this comparison group.
Figure 1 illustrates the effect of the Maternity Capital on birth rates. The top two panels show monthly birth rates (simple counts and de-seasoned); the bottom panels show total fertility rates in Russia versus Eastern European countries, and versus the European Union and the US.
Figure 1. Total Fertility Rate, Russia, Eastern European countries, USA and EU.
Source: Sorvachev and Yakovlev (2019), and http://www.fertilitydata.org/.
The effects of the policy are not limited to fertility. This policy affects family stability: it results in a reduction in the share of single mothers and in the share of non-married mothers.
Also, the policy affects the housing market. Out of three options (education, housing and pension), 88% of families use Federal Maternity Capital money to buy housing. We find that the supply of new housing and housing prices increased significantly as a result of the program. Confirming a close connection between the housing market and fertility, we find that in regions where the subsidy has a higher value for the housing market, the program has a larger effect: the effect of maternity capital was stronger, both in the short run and long run, in regions with a shortage of housing, and in regions with a higher ratio of subsidy to price of apartments (i.e. those regions where the real price of subsidy as measured in square meters of housing is higher).
Figure 2 below shows the effect of Federal Maternity Capital on birth rates in different regions. It shows no effect on fertility in Moscow, small effect in Saint-Petersburg; whereas the sizable effect of maternity capital in other Russian regions.
Figure 2. Effect of Federal Maternity capital, by regions
Source: Sorvachev and Yakovlev (2019), and http://www.gks.ru/.
These results suggest that cost-benefit analysis of such policies should go beyond the short-run and long-run effects on fertility. Ignoring general equilibrium issues may result in substantial bias in the evaluation of both short-run and long-run costs and benefits of the program.
While there are many benefits of the program, we show that this government intervention comes at substantial costs: the government’s willingness to pay for an additional birth induced by the program equals approximately 50,000 dollars.[1]
For more detailed evaluation of the results see Evgeny Yakovlev and Ilia Sorvachev, 2019, “Short-Run and Long-Run Effects of Sizable Child Subsidy: Evidence from Russia”, NES working Paper # 254, 2019.
References
- Acemoglu, Daron 2010 “Theory, General Equilibrium, Political Economy and Empirics in Development Economics”, Journal of Economic Perspectives, 24(3), pp. 17-32. 2010
- Adda, Jérôme, Christian Dustmann and Katrien Stevens 2017. “The Career Costs of Children”. Journal of Political Economy, 125, 2, 293-337.
- Ilia Sorvachev and Evgeny Yakovlev, 2019, “Short-Run and Long-Run Effects of Sizable Child Subsidy: Evidence from Russia”, NES working Paper #254 and LSE IGA Research Working Paper Series 8/2019
[1] Roughly, the WTP (US$50,000) exceeds nominal US$10,000 subsidy because the government pays for all (100%) families that give birth to a child to induce additional (20%) increase in fertility. See paper for more accurate elaboration.
Social Media and Xenophobia
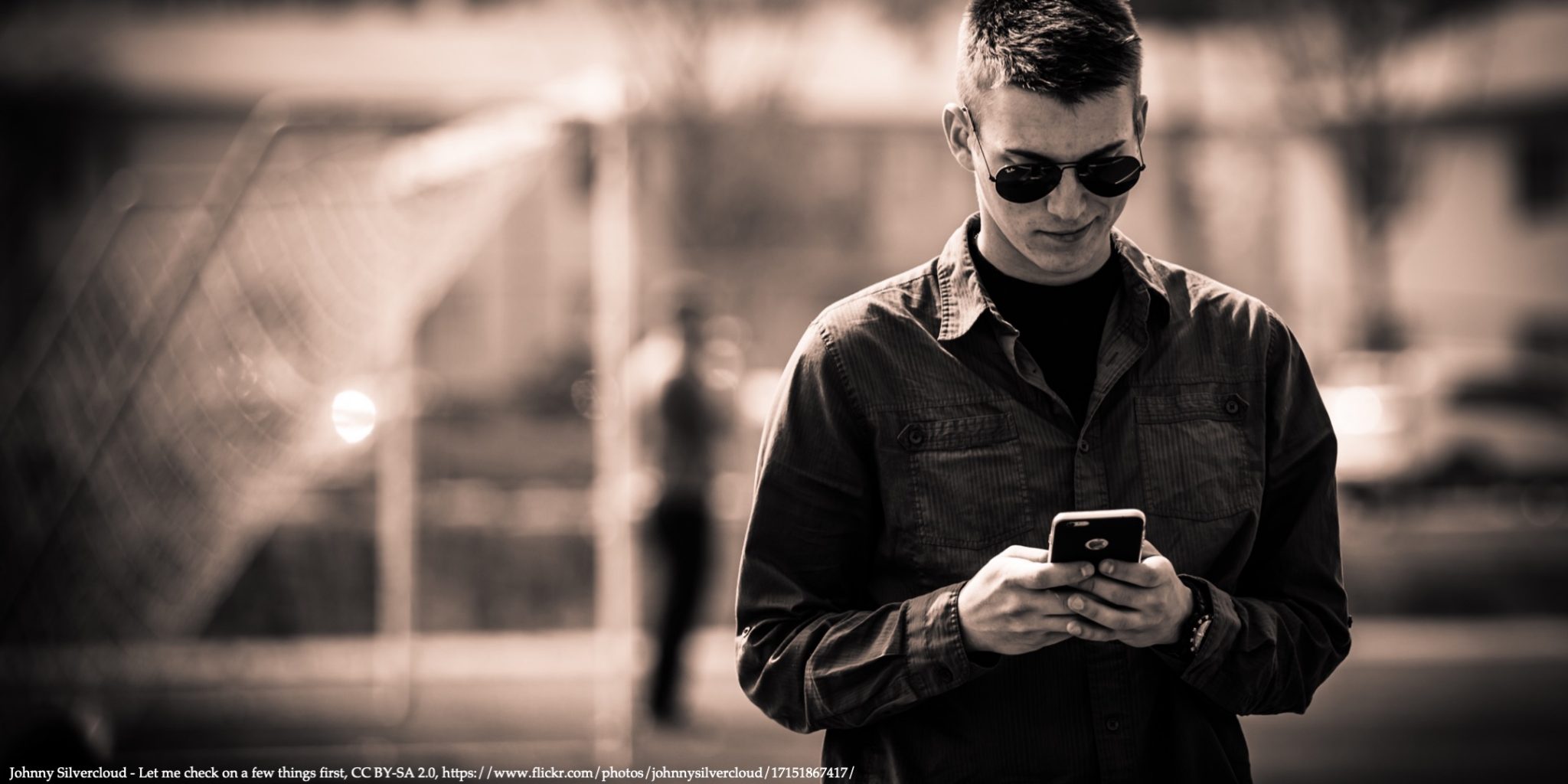
We study the causal effect of social media on hate crimes and xenophobic attitudes in Russia, using variation in social media penetration across cities. We find that higher penetration of social media leads to more ethnic hate crimes, but only in cities with a high baseline level of nationalist sentiment prior to the introduction of social media. Consistent with a mechanism for the coordination of crimes, the effects are stronger for crimes with multiple perpetrators. We show that social media penetration also had a persuasive effect on young and uneducated individuals, who became more likely to have xenophobic attitudes.
In recent years, the world has witnessed a large increase in expressions of hate, particularly of xenophobia. Candidates and platforms endorsing nationalism and views associated with intolerance toward specific groups have also gathered increased popular support both in the U.S. and across Europe. There is a lot of speculation about the potential drivers of this increase in the expression of hate. In our recent paper (Enikolopov et al, 2019) we study the role of social media in this process. This brief introduces the topic and offers a short outline of our findings.
Conceptually, social media could foster hate being expressed through different channels. First, social media reduces the cost of coordination. For example, there is evidence that it facilitates political protest (Enikolopov, Makarin, Petrova, 2018). Coordination facilitated through social media might be particularly relevant for illegal and stigmatized activities, such as hate crime: social media might make it easier to find like-minded people (through targeted communities and groups); it might also reduce the cost of asking or exposing oneself by providing a more anonymous forum for social interactions. Social media might also influence people’s opinions: tolerant individuals might be more exposed to intolerant views, while intolerant individuals might end up in an “echo chamber” (Sunstein 2001, 2017, Settle 2018) that make their views even more extreme. In our paper, we study the causal effect of social media exposure on xenophobic crimes and xenophobic attitudes in Russia and provide evidence on the particular mechanisms behind these effects.
The challenge in identifying a causal effect of social media is that access and consumption of social media are not randomly assigned. To surmount this challenge, we follow the approach of Enikolopov et al. (2018) and exploit a feature of the introduction of the main Russian social media platform – VKontakte (VK). This social media, which is analogous to Facebook in functionality, was the first mover on the Russian market and secured its dominant position with a user share of over 90% by 2011. VK was launched in October 2006 by Pavel Durov, its founder, who at that time was an undergraduate student at St Petersburg State University (SPbSU). Initially, users could only join the platform by invitation, through a student forum of the University, which was also created by Durov.
As a result, the vast majority of the early users of VK were students of SPbSU. This, in turn, made their friends and relatives more likely to open an account. And since SPbSU attracted students from around the country, this sped up the development of VK in the cities, from which these students were coming from. Network externalities magnified these effects and, as a result, the idiosyncratic variation in the distribution of the home cities of Durov’s classmates had a long-lasting effect on VK penetration. Following this logic, we use fluctuations in the distribution of student of SPbSU across cities as an instrument for the city-level penetration of VK. We then evaluate the effect of higher VK penetration on hate crimes and hate attitudes, combining data on hate crimes for the period between 2007 and 2015 collected by a reputable Russian NGO SOVA with survey data on hate attitudes.
Previous findings indicate that whether information from media induces people to be involved in the active manifestation of xenophobic attitudes or not depends on predispositions of the population. For example, Adena et al (2015) demonstrate that radio propaganda by the Nazis in the 1930’s was effective only in areas with a historically high levels of anti-Semitism. The role of the underlying level of nationalism is likely to be even stronger for social media, in which the content of the media itself directly reflects the attitudes of the population. This is particularly relevant for hate crimes committed by multiple perpetrators, in which social media can facilitate the coordination of such crimes.
Thus, we test whether the effect of social media depends on the pre-existing level of nationalism. To get at this underlying sentiment, we break cities by their level of support for the Rodina (“Motherland”) party, which ran in the national 2003 elections (the last parliamentary elections before the creation of VK) on an explicit nationalistic, xenophobic platform.
We find that penetration of social media leads to more ethnic hate crimes, but only in cities with a high baseline level of nationalist sentiment prior to the introduction of social media. For example, in cities with a maximum level of support of Rodina an increase in the number of VK users by 10% lead to an increase in ethnic hate crimes by 20%, while it had no significant effect on hater crime in cities with minimal support of Rodina. There is also no evidence that future social media penetration is related to ethnic hate crimes before the creation of social media, regardless of the level of pre-existing nationalistic attitudes.
Further evidence is consistent with social media playing a coordination role in hate crimes. The effect of social media is stronger for crimes perpetrated by multiple individuals (as opposed to crimes committed by a single person), where coordination is more important. These heterogeneous effects are also not consistent with results being simply driven by a higher likelihood of hate crime in places with higher social media penetration, unless this effect were present precisely in cities with higher support for Rodina and for crimes with multiple perpetrators, for example – which we find unlikely.
Having found evidence of a causal effect of social media on ethnic hate crimes, consistent with a mechanism of coordination, we turn next to the impact of social media on xenophobic attitudes. We designed and organized an online survey, and launched it in the summer of 2018, reaching 4,327 respondents from 64 cities. To measure xenophobic attitudes, we examined answers to the question “Do you feel irritation of dislike for individuals from some other ethnicities?” Note that, unlike the coordination of hate crimes, the persuasive effects of social media are not necessarily expected to be strongest in cities with higher baseline nationalistic sentiment since individuals on social media can get as easily connected to people outside their city. In fact, it is conceptually possible that the persuasion would be stronger in cities with lower baseline nationalistic sentiment: individuals might have previously been less aware of and less exposed to these types of views before the introduction of social media.
Since there might be a stigma in reporting xenophobic attitudes even in anonymous surveys, we use a “list experiment” to approximate “truly-held” xenophobic attitudes. In particular, the list experiment works as follows: first, respondents are randomly assigned either into a control group or a treatment group. Respondents in all groups are asked to indicate the number of policy positions they support from a list of positions on several issues. Support for any particular policy position is never indicated, only the total number of positions articulated on the list that a respondent supports. In the control group, the list includes a set of contentious, but not stigmatized, opinions. In the treatment group, the list includes all the contentious opinions from the control list, but also adds the opinion of interest, which is potentially stigmatized. The degree of support for the stigmatized opinion can be assessed by comparing the average number of issues supported in the treatment and control groups. The question of interest, randomly added to half of the questionnaires, was “Do you feel irritation of dislike for individuals from some other ethnicities?”.
The results indicate that the average share of people who agree with the statement is 37%. While there is no significant effect of social media penetration on xenophobic attitudes for the whole sample, there is a significant effect for important subsamples, which are at a higher risk of being involved in hate crime, such as respondents with lower levels of education or young respondents. Of course, the individuals that became more likely to engage in hate crime are not necessarily the same that have been persuaded to have more xenophobic attitudes (especially given the question used to assess attitudes) – though it is possible that some individuals who would have been close to committing crimes in the absence of social media might have been persuaded enough to switch their behavior in the presence of social media.
At the same time, we do not find that social media leads to an increase in xenophobic attitudes when measured with a direct question. The results are confirmed if we use a much larger, nationally representative survey of more than 30,000 respondents conducted by one of the biggest Russian survey companies FOM in 2011. In principle, it is possible that social media not only changed real attitudes but also the perception of the social acceptability of expressing these attitudes. However, we do not find any evidence that social media reduces the stigma of admitting xenophobic attitudes. The fact that we find the effect of social media on actual attitudes, but not on the expressed ones suggests, that if anything the stigma increased, at least for the respondents who acquired xenophobic attitudes as a result of social media influence. This highlights the importance of using a survey method that reduces concerns with social acceptability, such as list experiments.
Overall, our results indicate that social media lead to an increase in both ethnic hate crimes and xenophobic attitudes in Russia. However, the effect on hate crime is observed only in cities in which there was already a high level of nationalism. Additional evidence indicates that this effect is driven both by facilitating the coordination of nationalists and by persuading people to become more xenophobic. These findings contribute to a growing body of evidence that social media is a complex phenomenon that has both positive and negative effects on the welfare of people (see also Allcott et al, 2019), which has to be taken into account in discussing policy implications of the recent changes in media technologies.
References
- Allcott, H., Braghieri, L., Eichmeyer, S., Gentzkow, M. (2019) “The Welfare Effects of Social Media”, Working paper.
- Burzstyn, L., Egorov, G., Enikolopov, R., Makarin, A. (2019) “Social Media and Xenophobia: Evidence from Russia”, Working paper.
- Enikolopov, R., Makarin, A., Petrova, M. (2018) “Social Media and Protest Participation: Evidence from Russia“, Working paper.
- Settle, J. E. (2018) Frenemies: How Social Media Polarizes America. Cambridge University Press.
- Sunstein, C. (2001) Republic.com. Princeton University Press.
- Sunstein, C. (2017) Republic: Divided Democracy in the Age of Social Media. Princeton University Press.
Disclaimer: Opinions expressed in policy briefs and other publications are those of the authors; they do not necessarily reflect those of the FREE Network and its research institutes.
Russia’s Real Cost of Crimean Uncertainty
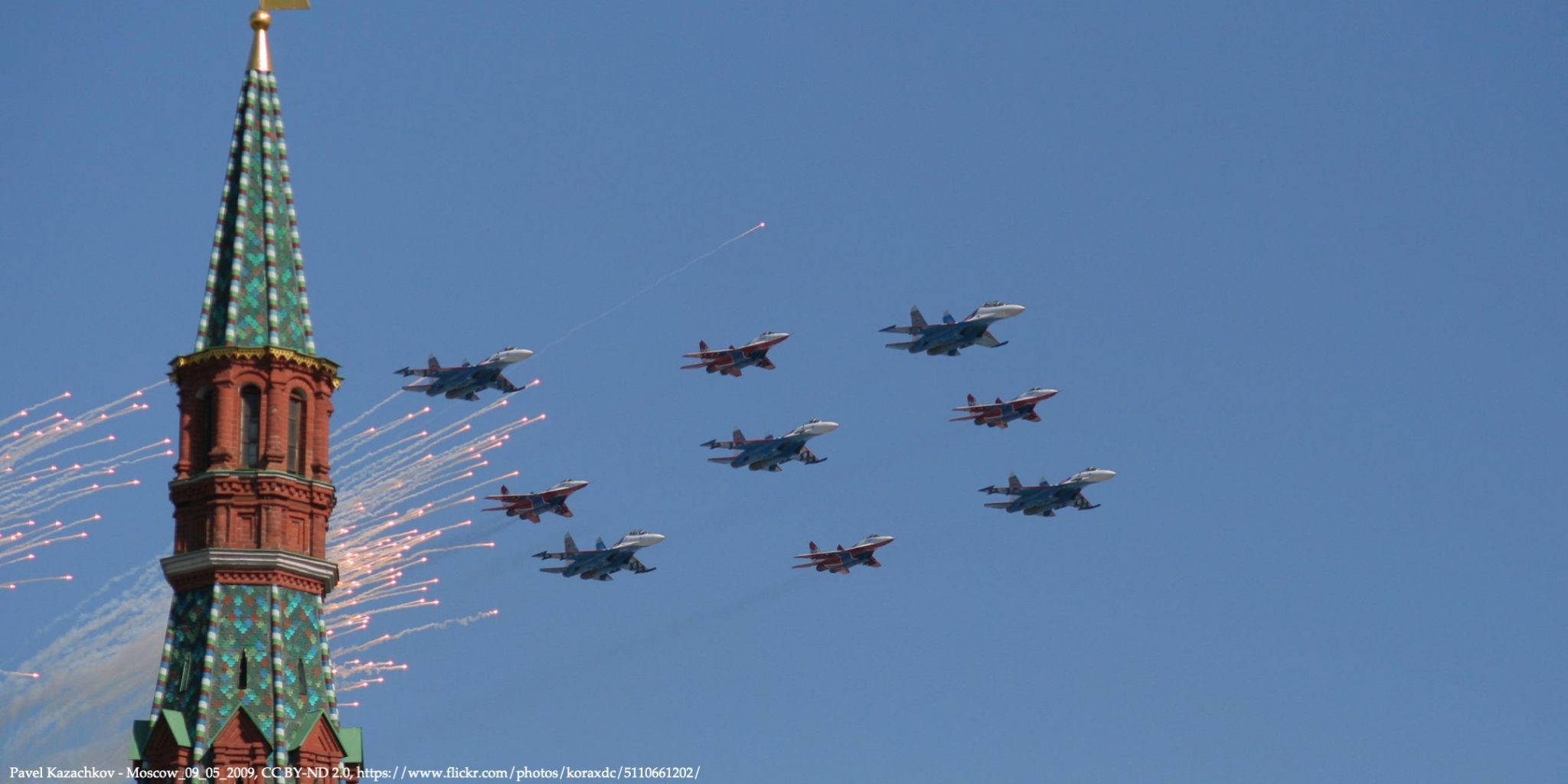
The annexation of Crimea has real costs to the Russian economy beyond what is measured by some items in the armed forces’ budget; social spending in the occupied territories; or the cost of building a rather extreme bridge to solve logistics issues. Russia’s real cost of the annexation of Crimea is also associated with the permanent loss of income that the entire Russian population is experiencing due to increased uncertainty, reduced capital flows and investment, and thus a growth rate that is significantly lower than it would have been otherwise. Since the years of lost growth are extremely hard to make up for in later years, there will be a permanent loss of income in Russia that is a significant part of the real cost of annexing Crimea and continuing the fighting in Eastern Ukraine. It is time to stop not only the human bleeding associated with Ukraine, but also the economic.
Estimating the real cost of Russia’s annexation of Crimea and the continued involvement in Eastern Ukraine is complicated since there are many other things going on in the Russian economy at the same time. In particular, oil prices fell from over $100/barrel in late 2013 to $30/barrel in 2016 (Figure 1). Becker (2016) has shown that 60-80 percent of the variation in GDP growth can be explained by changes in oil prices, so this makes it hard to just look at actual data on growth to assess the impact of Crimea and subsequent sanctions and counter sanctions.
Figure 1. Russian GDP and oil price
Source: Becker (2019)
The approach here is instead to focus on one channel that is likely to be important for growth in these circumstances, which is uncertainty and its impact on capital flows and investment.
From uncertainty to growth
The analysis presented here is based on several steps that link uncertainty to GDP growth. All the details of the steps in this analysis are explained at some length in Becker (2019). Although this brief will focus on the main assumptions and estimates that are needed to arrive at the real cost of Crimea, a short description of the steps is as follows.
First of all, in line with basic models of capital flows, investors that can move their money across different markets (here countries) will look at relative returns and volatility between different markets. When relative uncertainty goes up in one market, capital will leave that market.
The next step is that international capital flows affect investment in the domestic market. If capital leaves a country, less money will be available for fixed capital investments.
The final step is that domestic investments is important for growth. Mechanically, in a static, national accounts setting, if investments go down, so does GDP. More long term and dynamically, investments have a supply side effect on growth, and if investments are low, this will affect potential as well as actual growth negatively.
These steps are rather straightforward and saying that uncertainty created by the annexation of Crimea leads to lower growth is trivial. What is not trivial is to provide an actual number on how much growth may have been affected. This requires estimates of a number of coefficients that is the empirical counterparts to the theoretical steps outlined here.
Estimates to link uncertainty to growth
In short, we need three coefficients that link: domestic investments to growth; capital flows to domestic investments; and uncertainty to capital flows.
There are many studies that look at the determinants of growth, so there are plenty of estimates on the first of these coefficients. Here we will use the estimate of Levine and Renelt (1992), that focus on finding robust determinants of growth from a large set of potential explanatory variables. In their preferred specification, growth is explained well by four variables, initial income, population growth, secondary education and the investments to GDP ratio. The coefficient on the latter is 17.5, which means that when the investment to GDP ratio increases by 10 percentage points, GDP grows an extra 1.75 percentage points per year. Becker and Olofsgård (2018) have shown that this model explains the growth experience of 25 transition countries including Russia since 2000 very well, which makes this estimate relevant for the current calculation.
The next coefficient links capital flows to domestic investments. This is also a subject that has been studied in many empirical papers. Recent estimates for transition countries and Russia in Mileva (2008) and Becker (2019) find an effect of FDI on domestic investments that is larger than one, i.e., there are positive spillovers from FDI inflows to domestic investments. Here we will use the estimate from Becker (2019) that finds that 10 extra dollars of FDI inflows are associated with an increase of domestic investments of 15 dollars.
Finally, we need an estimate linking uncertainty with capital flows. There are many studies looking at risk, return and investment in general, and also several studies focusing on international capital flows and uncertainty. Julio and Yook (2016) look at how political uncertainty around elections affect FDI of US firms and find that FDI to countries with high institutional quality is less affected by electoral uncertainty than others. Becker (2019) estimates how volatility in the Russian stock market index RTS relative to the volatility in the US market’s S&P 500 is associated with net private capital outflows. The estimate suggests that when volatility in the RTS goes up by one standard deviation, this is associated with net private capital outflows of $30 billion.
These estimates now only need one more thing to allow us to estimate how much Crimean uncertainty has impacted growth and this is a measure of the volatility that was created by the annexation of Crimea.
Measuring Crimean uncertainty
In Becker (2019), the measure of volatility that is used in the regression with net capital outflows is the 60-day volatility of the RTS index. Since we now want to isolate the uncertainty created by Crimea related events, we need to take out the volatility that can be explained by other factors in order to arrive at a volatility measure that captures Crimean induced uncertainty. In Becker (2019) this is done by running a regression of RTS volatility on the volatility of international oil prices and the US stock market as represented by the S&P 500. The residual that remains after this regression is the excess volatility of the RTS that cannot be explained by these two external factors. The excess volatility of the RTS index is shown in figure 2.
It is clear that the major peaks in excess volatility are linked to Crimea related events, and in particular to the sanctions introduced at various points in time. From March 2014 to March 2015, there is an average excess volatility of 0.73 standard deviations with a peak of almost 4 when the EU and the USA ban trade with Crimea. This excess volatility is our measure of the uncertainty created by the annexation of Crimea.
Figure 2. RTS excess volatility
Source: Becker (2019)
From Crimean uncertainty to growth
The final step is simply to use our measure of Crimean induced uncertainty together with the estimates that link uncertainty in general to growth.
The estimated excess volatility associated with Crimea is conservatively estimated at 0.7 standard deviations. Using this with the estimate that increasing volatility by one standard deviation is associated with $30 billion in capital outflows, we get that the Crimean uncertainty would lead to $21 billions of capital outflows in one quarter or $84 billions in one year. If this is in the form of reduced FDI flows, we have estimated that this means that domestic investments would fall by a factor of 1.5 or $126 billions.
In this period, Russia had a GDP of $1849bn and fixed capital investments of $392bn. This means that $126 billions in reduced investments correspond to a reduction in the investments to GDP ratio of 7 percentage points (or that the investments to GDP ratio goes from around 21 percent to 14 percent).
Finally, using the estimate of 17.5 from Levine and Renelt, this implies that GDP growth would have been 1.2 percentage points higher without the estimated decline in investments to GDP.
In other words, the Crimean induced uncertainty is estimated to have led to a significant loss of growth that has to be added to all the other costs of the annexation of Crimea and continued fighting in Eastern Ukraine. Note that recent growth in Russia has been just barely above 1 percent per year, so this means that growth has been cut in half by this self-generated uncertainty.
Of course, the 1.2 percentage point estimate of lost growth is based on many model assumptions, but it provides a more sensible estimate of the cost of Crimea than we can get by looking at actual data that is a mix of many other factors that have impacted capital flows, investments and growth over this period.
Policy conclusions
The annexation of Crimea and continued fighting in Eastern Ukraine carry great costs in terms of human suffering. In addition, they also carry real costs to the Russian economy. Not least to people in Russia that see that their incomes are not growing in line with other countries in the world while the value of their rubles has been cut in half. Some of this is due to falling oil prices and other global factors that require reforms that will reorient the economy from natural resource extraction to a more diversified base of income generation. This process will take time even in the best of worlds.
However, one “reform” that can be implemented over night is to stop the fighting in Eastern Ukraine and work with Ukraine and other parties to get out of the current situation of sanctions and counter-sanctions. This would provide a much-needed boost to foreign and domestic investments required to generate high, sustainable growth to the benefit of many Russians as well as neighboring countries looking for a strong economy to do trade and business with.
References
- Becker, T, (2019), “Russia’s macroeconomy—a closer look at growth, investment, and uncertainty”, forthcoming SITE Working paper.
- Becker, T. and A. Olofsgård, (2018), “From abnormal to normal—Two tales of growth from 25 years of transition”, Economics of Transition, vol. 26, issue 4.
- Becker, T. (2016), “Russia and Oil – Out of Control”, FREE policy brief, October.
- Julio, B. and Yook, Y. (2016), ‘Policy uncertainty, irreversibility, and cross-border flows of capital’, Journal of International Economics, Vol. 103, pp. 13-26.
- Levine, R. and Renelt, D. (1992). ‘A Sensitivity Analysis of Cross-Country Growth Regressions’, American Economic Review, 82(4), pp. 942–963.
- Mileva, E. (2008), ‘The Impact of Capital Flows on Domestic Investment in Transition Economies, ECB Working Paper No. 871, February.
Disclaimer: Opinions expressed in policy briefs and other publications are those of the authors; they do not necessarily reflect those of the FREE Network and its research institutes.
Resident Altruism and Local Public Goods
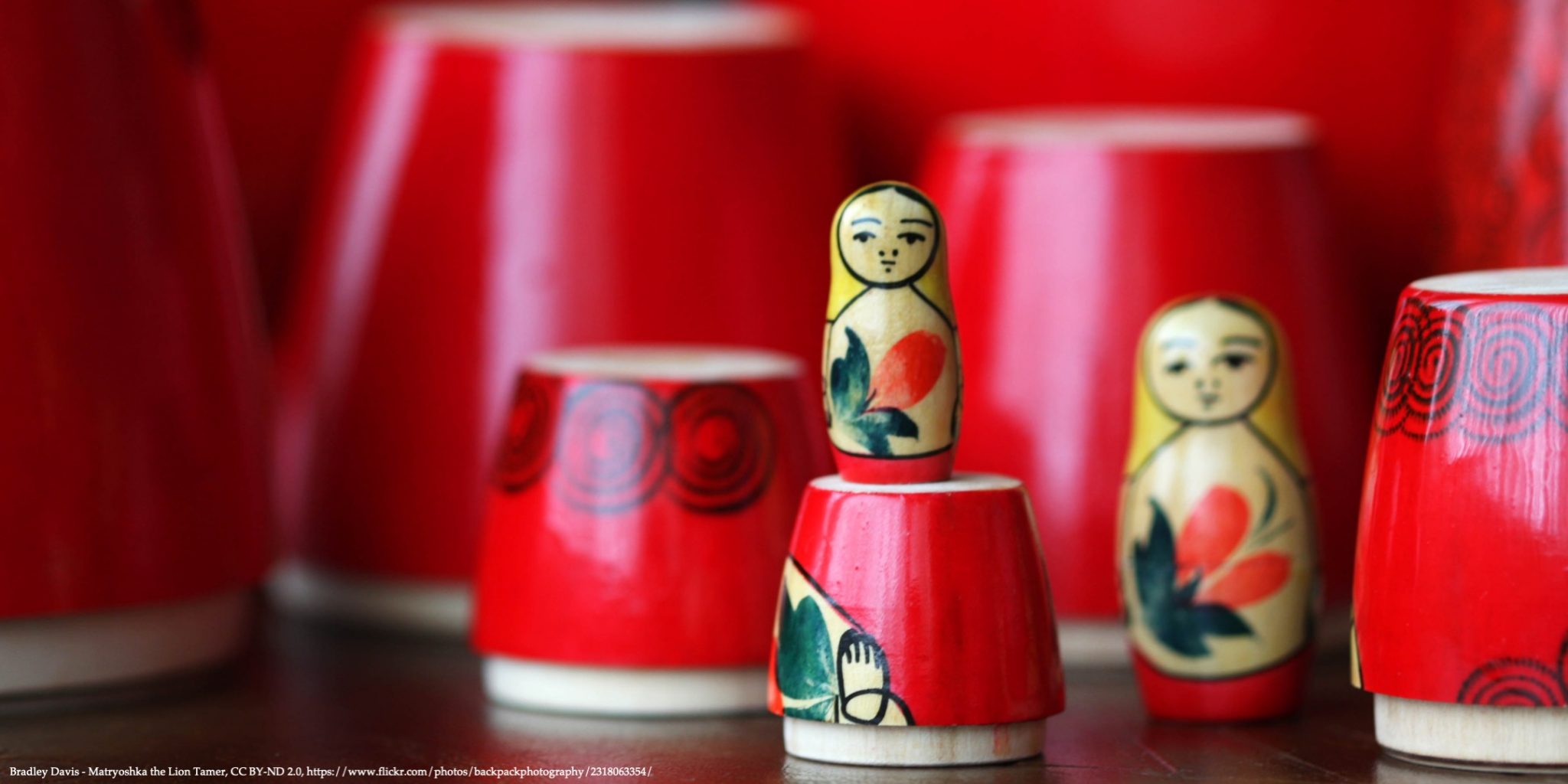
This policy brief discusses residents’ voluntary payments for local public goods in Russian municipalities in a historic and a comparative international context. We emphasize the behavioral aspects of such collective action and the political economy risks of implementing this financial mechanism. Finally, we use data from the Russian Federal Treasury to offer empirical evidence on the regional variation in the amounts of these payments.
Theoretical grounds
One of the drawbacks of a system of fiscal federalism is that it often results in an inadequate distribution of fiscal authority between regional and local governments. As a result, municipalities may be incapable of levying a due amount of taxes for the provision of the required quantity or quality of local public goods. A solution to the problem may be found in residents’ voluntary monetary and nonmonetary contributions to local projects. In Russia these are financial contributions to projects such as the renovation of roads, pedestrian bridges, parks, sports grounds and playgrounds, street lighting, the cleaning of ponds and rivers, etc. (Besstremyannaya, 2019). The experience of municipalities in the U.S. provides similar examples of residents’ financial contributions to municipal projects: here these resources are used, for example, as additional funds for financing secondary education (Winerip, 2003).
There are several potential theoretical explanations for the motivation of individuals to engage in such voluntary contributions. To start with, they can be motivated by purely altruistic concerns about local public welfare and the benefits of others (Ferris, 1984). Alternatively, the motives of individuals may be better (and, perhaps, more realistically) described by the approach of impure altruism (Andreoni, 1990), which trades off the amount of the public good against the size of contribution in motivating the behavior of the individual.
Further, the phenomenon of voluntary contributions is closely related to the desire of local authorities to substitute for insufficient budgetary revenues. As a result, residents may be coerced to submit monetary or in-kind labor payments for community development (Beard, 2007), for instance to put asphalt on rural roads (Olken and Singhal, 2011). Accordingly, instead of referring to residents’ contributions as donations, one may consider this mechanism of raising extra resources for local budgets as a type of informal tax. Arguably, altruism for the provision of public goods may be more prevalent among residents in developed countries, while contributions for local projects in developing countries reflect direct or indirect coercion by local authorities.
This policy brief analyses voluntary contributions to municipal budgets by residents in modern Russia. The presence of only fragmentary evidence from other countries limits our formal comparative analysis. However, we attempt to summarize common issues on the implementation of this financial mechanism.
Russian experience and legal framework
In contemporary Russia, residents’ contributions to local projects are called self-taxes (“samooblozheniye”) and constitute non-tax revenues of local governments (Article 131 of the Budget Code of 1998, Article 56 of the Local Government Law of 2003).
According to the Budget Code, self-taxes are fixed-size and one-time payments by residents for purposes of local projects, with the list of projects initiated directly by residents and voted at a local referendum. The projects commonly include activities on improving local infrastructure (Balynin, 2015). The December 2017 revision of the Local Government Law attempted to add additional incentives for self-financing by making it more targeted (in terms choosing urgent local projects) and easier to coordinate (in terms of organizing a referendum) by allowing referendums by residents of only selected parts of a municipality.
According to the Budget Code of the Russian Federation, self-taxes are earmarked items in non-tax revenues of local budgets, and may not be interrelated with other types of revenues or with the deficit of local government.
It should be noted that the use of self-taxes is not new in modern Russia: this funding mechanism was temporary exploited by Soviet authorities in the 1920s and 1930s, and was revitalized in the emerging Russian economy in the 1990s.
The early use of self-taxes in Soviet Russia illustrates the issue with the transformation of self-taxation into informal taxation. Self-taxation was introduced in 1924 as a formally voluntary decision of residents on financing local public goods. However, one may doubt whether the decision was indeed made by residents without pressure. Moreover, the lists of local public goods to be financed by self-taxes were determined by public authorities (Resolution by the Central Executive Committee and Soviet of National Commissars of the USSR of 3.08.1931). An illustration of the opposition to this informal tax may be found in the protocols of the council of residents of Roksanka, a village in Kaluzhskaya region from August 1928: citizens decided not to use self-taxes to finance a local school, since they believed there were sufficient budgetary funds – namely revenues from sold public property (Sergienko, 2015).
Calls to avoid similar retransformation of self-taxes into an informal tax were noted in modern Russia in 2006-2007, when the Bills on the amendments to the Local Government Law attempted to empower local authorities with the rights to deal with issues of self-taxation (Emetz and Makarov, 2016).
International experience
Private contributions in the form of monetary payments or labor participation are common in developing countries and are explained by the need to improve the insufficient quality of basic local public goods. For instance, the mechanism is used for road construction, water supply or primary education (Olken and Singhal, 2011). At the same time, residents of developed countries may choose to contribute to sustaining a high quality of local public goods: fire departments, medical centers, museums (Bice and Hoyt, 2000; Ferris, 1984). For example, the introduction of a redistributional mechanism of budgetary funds across rich and poor municipalities may lead to a decrease in the quality of public goods in the richest municipalities (owing to a fall in per capita funds after the redistribution). Accordingly, residents of rich municipalities may voluntarily decide to collect extra funds to recover the formerly high quality of public goods (e.g. secondary education in the U.S., see Brunner and Sonstelie, 2003; Winerip, 2003).
A common challenge to implementing a mechanism of voluntary payments is associated with the difficulties of reaching a decision within a large group of individuals. Indeed, residents may demonstrate selfish behavior or may follow selected local leaders (Jack and Recalde, 2015; Blackwell and McKee, 2003). Moreover, the common lack of enforcement instruments makes voluntary contributions unreliable (Slemrod, 1998).
Interestingly, the methods of dealing with non-compliance are similar across countries: the Perm krai of Russia, municipalities in the U.S. and villages in developing countries use techniques as such notification by mail, home visits, disclosing the lists of non-compliers and employing various ways of informal coercion by neighbors or public leaders (Olken and Sighal, 2011; Miguel and Gugerty, 2005; Winerip, 2003).
Data from Russian regions
We use the 2013-2016 annual data of the Russian Treasury, which allows disentangling self-taxes as an item in the list of non-tax revenues of local budgets. Owing to municipal-level data being unavailable, our analysis concerns the sum of local budgets in each region. Only 33 regions out of 83 analyzed regions had positive self-tax revenues in 2013, and the leading regions in 2015-2016 are the Tatarstan Republic, the Bashkortostan Republic, Kirovskaya oblast, Lipetskaya oblast, Kaluzhskaya oblast, and Perm Krai. The share of self-taxes in non-tax revenues is rather low: it amounts to 2-3% in Tatarstan, while it is less than 1% in the remaining regions (Table 1).
Table 1. Top regions according to levied self-taxes in 2015-2016
Self-taxes in 2015 | Self-taxes in 2016 | |||
Thousand rubles | % of non-tax revenues of local budgets | Thousand rubles | % of non-tax revenues of local budgets | |
Tatarstan republic | 122268.26 | 2.11 | 183413.26 | 3.44 |
Kirovskaya oblast | 11431.02 | 0.50 | 7301.01 | 0.36 |
Bashkortostan republic | 1943.96 | 0.02 | 2781.02 | 0.03 |
Lipetskaya oblast | 3670.65 | 0.22 | 2701.14 | 0.16 |
Kaluzhskaya oblast | 2073.97 | 0.11 | 2620.54 | 0.15 |
Permskii krai | 3087.31 | 0.08 | 2335.02 | 0.06 |
Republic South Osetiya-Alaniya | 1807.11 | 0.38 | 1451.16 | 0.30 |
Tyva republic | 792.93 | 0.42 | 1342.60 | 0.78 |
Rostovskaya oblast | 1307.36 | 0.02 | 1124.40 | 0.02 |
Zabaikalskiy krai | 847.58 | 0.08 | 1092.68 | 0.11 |
Samarskaya oblast | 1023.76 | 0.02 | 978.87 | 0.02 |
Arguably, the share of self-taxes in non-tax revenues is not associated with the desire to compensate for insufficient transfers from the federal or regional budgets: the absolute value of the correlation coefficient with the share of transfers to local budgets in non-tax revenues is below 0.25 (Besstremyannaya, 2019, Table 1). Similarly, we found no interrelation of the share of self-taxes with such socio-economic variables as (per capita) gross regional product and density of population.
Next, we focus on the policy of regional governments to provide budgetary funds on top of the money collected through self-taxes. As of 2016, such regional co-financing was present in the Tatarstan Republic, Kirovskaya oblast, Vladimirskaya oblast and Perm Krai (Emetz and Makarov, 2016; Balynin, 2015). The coefficient of regional co-financing of local projects (the amount of regional funds over the locally provided funds) equals 1 in Vladimirskaya oblast and varies from 1.5 to 5 in other above-mentioned regions. Examples of such co-provision of local public goods by region and municipalities include the renovation of water supply facilities in Perm Krai and the cleaning of lakes in Tatarstan (Nikitin, 2018, Platoshino budget, 2017).
Our estimates reveal that coefficients higher than 1 are associated with a higher prevalence of self-taxes. Indeed, the increase in the share of self-taxes in the revenues of local budgets in such regions is much higher than the corresponding growth in regions without co-financing or with unity co-financing (Besstremyannaya, 2019, Table 2).
Finally, we use the data for Perm Krai which experienced a recent reform with a rise of the coefficient from 3 to 5 in 2014. Our estimates of the treatment effect of such a reform and an extrapolation to other regions reveal that a unit increase of the coefficient causes a 55% growth in the share of self-taxes in non-tax revenues (Besstremyannaya 2019, Table 3).
To sum up, regional co-financing of local projects is associated with a growth in the collected self-taxes.
Conclusion
The phenomenon of voluntary contributions to local budgets is relatively common in real life. However, the literature addressing it is rather fragmented. In particular, little is known empirically on the motivation of individuals to engage in such contributions.
Our analysis with the 2013-2016 annual data for Russian regions reveals that residents’ contributions to local public goods are unrelated to insufficient revenues by local budgets. Moreover, the share of residents’ contributions in the budgetary non-tax revenues is positively associated with regional co-financing of these local projects. Hence, one may conjecture that in Russia, this phenomenon may be viewed as an altruistic attempt to raise quality of local public goods or as a means to signal about the most urgent local projects to regional governments.
References
- Andreoni, J. (1990). Impure altruism and donations to public goods: A theory of warm-glow giving. The Economic Journal, Vol. 100(401), pp. 464-477.
- Balynin I.V. (2015). The use of self–taxes of citizens in forming the revenues of the local budgets. Finansy i Upravleniye, No.2, pp. 53–62. (In Russian).
- Beard, V. A. (2007). Household contributions to community development in Indonesia. World Development, Vol. 35(4), pp. 607-625.
- Besstremyannaya G.E. (2019) Informal taxes for the provision of public goods in Russian regions. Voprosy Ekonomiki No.1, pp 124-134. (In Russian).
- Bice D.C., Hoyt W.H. (2000). The impact of mandates and tax limits on voluntary contributions to local public services: An application to fire–protection services. National Tax Journal, Vol. 53(1), pp. 79–104.
- Blackwell C., McKee M. (2003). Only for my own neighborhood?: Preferences and voluntary provision of local and global public goods. Journal of Economic Behavior and Organization, Vol. 52(1), pp. 115–131.
- Brunner E., Sonstelie J. (2003). School finance reform and voluntary fiscal federalism. Journal of Public Economics, Vol. 87(9–10), pp. 2157–2185.
- CEFIR project for the Ministry of Finance of the Russian Federation “The development of methodological recommendations for increasing the revenues of Russian regions and municipalities” (Final report of November 2017)
- Emetz M.I., Makarov M.A. The self–taxation of citizens as a source of local budget revenues. Ekonomika i Menedgment Innovatsionnyh Tehnologii, No. 12, http://ekonomika.snauka.ru/2016/12/13433 (In Russian).
- Ferris J.M. (1984). Coprovision: Citizen time and money donations in public service provision. Public Administration Review, pp. 324–333.
- Jack B.K., Recalde M.P. (2015). Leadership and the voluntary provision of public goods: Field evidence from Bolivia. Journal of Public Economics, Vol. 122, pp. 80–93.
- Miguel E., Gugerty M.K. (2005). Ethnic diversity, social sanctions, and public goods in Kenya. Journal of Public Economics, Vol. 89(11–12), pp. 2325–2368.
- Nikitin, E. (2018) Self-taxation in Tatarstan republic provides for 4 to 1 budgetary cofinancing https://www.tatar-inform.ru/news/2018/02/16/593910/
- Olken B.A., Singhal M. (2011). Informal taxation. American Economic Journal: Applied Economics, Vol. 3(4), pp. 1–28.
- Platoshino budget for 2017 and forecast for 2018-2019. http://www.platoshino59.ru/index.php/2012-01-05-09-21-25/2016-08-28-09-18-38/item/403-publichnyj-byudzhet-platoshinskogo-selskogo-poseleniya-na-2017-god-i-planovyj-period-2018-i-2019-godov-byudzhet-dlya-grazhdan
- Sergienko, N.S. (2015). Self-taxation as a form of voluntary participation of the population in socioeconomic development of settlements. Sovremennye Issledovaniya Sotsialnyh Problem, Vol. 1, No. 21, pp. 266–270. (In Russian).
- Slemrod, J. (1998). On voluntary compliance, voluntary taxes, and social capital. National Tax Journal, Vol. 51(3), pp. 485–491.
- Winerip, M. 2003. On Education: Giving green or turning red. The New York Times, Feb 26.
Disclaimer: Opinions expressed in policy briefs and other publications are those of the authors; they do not necessarily reflect those of the FREE Network and its research institutes.
Capital Flows from Russia — The Bigger Picture
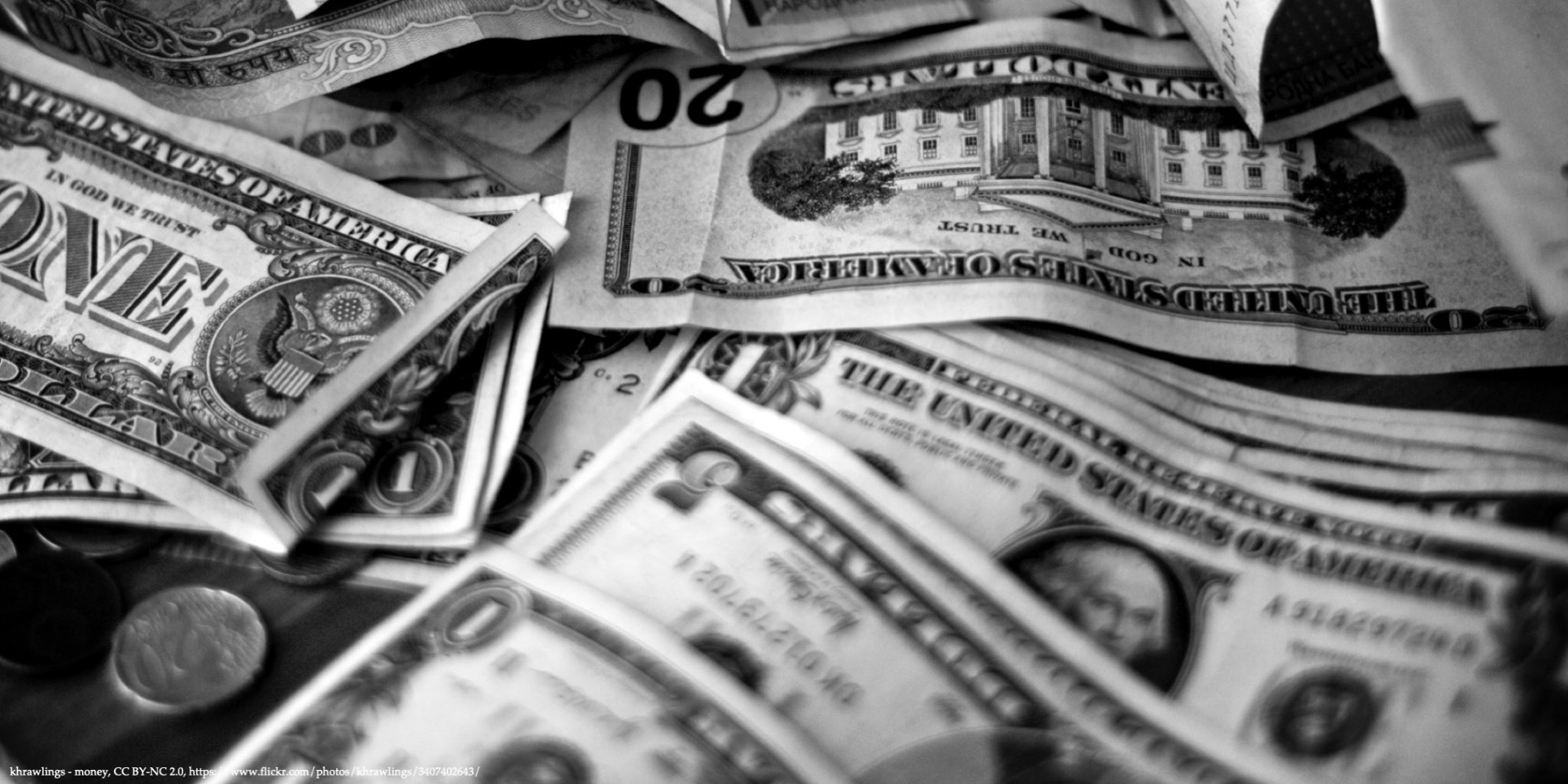
There is an increasing focus on how Russian capital flows are being channelled through Western banks to various destinations, including offshore havens. There are of course legitimate reasons and legal ways of moving capital across borders, but much of the international focus on capital flows in recent decades is linked to the financing of terrorism, tax evasion, and money laundering in connection with criminal activities. This brief provides the macro view of capital flows between Russia and the rest of the world to paint the bigger picture behind the more specific stories we read about in the news that involve individual businessmen, corrupt officials, criminals, and banks.
International capital movements have a clear role in allocating resources efficiently across countries. However, today’s media coverage instead typically focuses on the role of capital flows in financing terrorists and avoiding taxes. Recently, money laundering has been creating headlines around the world in the Panama papers and other similar stories, illuminating complicated schemes in the global financial system in connection with illegal activities such as tax evasion, corruption, drug dealing and human trafficking.
In the international policy making arena, since 1989, the Financial Action Task Force (FATF) has the objective “to set standards and promote effective implementation of legal, regulatory and operational measures for combating money laundering, terrorist financing and other related threats to the integrity of the international financial system”. After the terrorist attacks in 2001, the issues of anti-money laundering (AML) and combatting the financing of terrorism (CFT) also became a central area of the IMF’s work and has since become an increasingly important policy question.
In several of the news stories, money flowing from Russia features prominently. This brief provides the bigger picture of Russian capital flows based on publicly available data as a complement and background to the news stories that are based on inside information, or “leaks”, and that focus on particular individuals and banks.
Composition of capital flows
In the official balance of payments statistics, capital flows are divided into a number of different categories, for example, private vs public or banks vs non-banks. There is also a distinction made between foreign direct investments (FDI) on the one hand and portfolio flows, loans and other types of transactions (PLO) on the other. Since the balance of payments also has to balance (despite the fact that not all international transactions have been recorded) there is also a term called errors and omissions (E&O) that take care of various discrepancies. In environments with poor data collection and a large share of activities that take place “off the books”, this term tends to be large. For Russia, this term has become smaller over time as the economy and data collection has matured.
In terms of volatility and magnitude of flows, the distinction between FDI and PLO is often important and so also in Russia. Figure 1 shows the private sector flows to and from Russia over the last two plus decades.
Figure 1. Capital flows to and from Russia
Source: Central Bank of Russia and author’s calculations
After a rather slow start in the early years of transition, capital flows took off as Russia started to generate growth in 2001, and the flows kept growing until the global financial crisis. As expected, FDI flows have been less volatile than PLO flows but perhaps more surprising, in- and outflows in both categories seem to move closely together (see Becker (2019) on why this is the case). We can also note that there has been a marked downturn in flows at the time of the annexation of Crimea and subsequent sanctions and counter sanctions between the West and Russia.
Cumulative capital flows
By computing net flows from the data in Figure 1 and accumulating this over time, we get a clearer idea in Figure 2 of the massive amounts of capital that have left Russia over the last decades. In the early years, the outflows were in the form of errors and omissions (E&O) and PLO, but the PLO trend was reversed in the early 2000’s and turned total accumulated flows back to zero before the global financial crisis hit. The global financial crisis was a clear turning point for capital flows in general and PLO flows in particular.
Figure 2. Net private capital flows
Source: Central Bank of Russia and author’s calculations
In the year following the global financial crisis, almost USD 300 billion left Russia. Outflows then continued, albeit at a slower pace, only to accelerate again at the time of Russia’s annexation of Crimea. By mid-2018, USD 700 billion had left Russia since 2008, mainly in the form of PLO flows. This is equivalent to twice the amount of fixed capital investments in Russia in 2017.
For a country like Russia that is in need of increased investments both from domestic and foreign sources to generate long-term sustainable growth, these outflows are very costly at the macro level even if they are beneficial to individual entities that are behind the flows.
Destinations of capital flows
Where the money from Russia ultimately ends up should matter less to people in Russia than the fact that they are not invested and generating growth at home. However, it can matter a great deal to people, policy makers and businesses in the destination countries. Not only because it involves business opportunities and employment to some, but also because it generates concerns among regulators, law enforcement and tax authorities regarding the origins and purposes of the investments.
We do not have full coverage of where all the money Russian entities invest or park abroad end up, but official statistics are available for at least part of the investments. First of all, there is data on cross-border assets and liabilities of the banks that report to the Bank of International Settlement (BIS), which shows what foreign residents have deposited in the banks. Russian claims on BIS reporting banks are shown in Figure 3, where we can note that total claims by Russians amount to USD 131 billion. Half of this amount was deposited with French, Swiss, UK, and Belgian banks at the end of September 2018.
Figure 3. Russian claims on BIS reporting banks in different countries (USD bn, Sept. 2018)
Source: BIS and author’s calculations
Given the recent scandal in Danske Bank, we can also note that USD 8 billion was deposited by Russian entities in Danish banks, which may not sound much in this context but amounts to around 2 per cent of Danish GDP.
Again, macro level data does not tell us if the flows behind the numbers are illicit or legitimate, but it provides some sense of the order of magnitude and possible significance for the entities involved in the transactions and their regulators and supervisors.
The next piece of information is due to the IMF’s and others’ efforts to collect and harmonize data on the destination of portfolio and FDI assets, and the data for Russia is presented in Figures 4 and 5.
The prime locations for Russian owned portfolio assets are Ireland and Luxembourg, followed far behind by the Netherlands, UK and US. In total, official portfolio assets are rather modest at USD 69 billion, which is far off the cumulative net PLO flows in Figure 2 of over USD 500 billion even if we add the BIS reporting bank deposits in Figure 3.
Figure 4. Russian portfolio assets by the destination country (USD bn, Sept. 2018)
Source: Central Bank of Russia and author’s calculations
This could have many explanations, including that a significant share of Russian PLO assets is not in BIS reporting banks or in countries that provide transparent reporting of other types of PLO assets. The fact that cumulative flows and stocks reported in international statistics are so different, though, clearly asks the question where the remaining assets are invested.
The last component for which we have data is the location of Russian FDI assets. This turns out to be the most significant asset class available in the official statistics with a total of USD 364 billion invested abroad. Given that the magnitudes of FDI flows in Figures 1 and 2 are much smaller than PLO flows, this is somewhat surprising. Less surprising is the fact that more than half of this is invested in Cyprus, which is a well-known destination for Russian money.
However, it also begs the question on how assets are classified and where; Cyprus annual GDP was USD 24 billion in 2018, or 13 per cent of what is classified as Russian FDI assets in Cyprus. The only reasonable interpretation is that Cyprus is an offshore destination to park Russian money and not the ultimate location of direct investments from Russia. It is not unlikely that similar explanations are also valid for a significant share of the assets recorded as investments in the Netherlands, Austria and Switzerland, not to mention the British Virgin Islands (BVI) or the Bahamas. This problem is not unique for Russian data, but the magnitude of the problem regarding this data is still striking.
Figure 5. Russian FDI assets by the destination country (USD bn, Sept. 2018)
Source: Central Bank of Russia and author’s calculations
Policy conclusions
Capital leaving Russia is mainly a problem for investments and growth in Russia, but, as has become far too clear recently, some of the flows also create problems in other countries. In particular, flows that are associated with money laundering and channelled through financial institutions in the West can create massive problems for banks that do not have sufficient control mechanisms in place or are guided by short-term profit maximization that encourages staff to look the other way when illicit flows are coming in.
Given the massive scale of flows coming from Russia, it can obviously be tempting to be part of this business while at the same time very costly to implement procedures and routines that control all of the flows adequately. However, not understanding the bigger picture of Russian flows can be even costlier.
References
- Becker, T, (2019), “Russia’s macroeconomy—a closer look at growth, investment, and uncertainty”, forthcoming SITE Working paper.
Disclaimer: Opinions expressed in policy briefs and other publications are those of the authors; they do not necessarily reflect those of the FREE Network and its research institutes.
The Tragedies in the Soviet Countryside of the Early 1930s: Research Frontiers and Rival Interpretations of the Famines
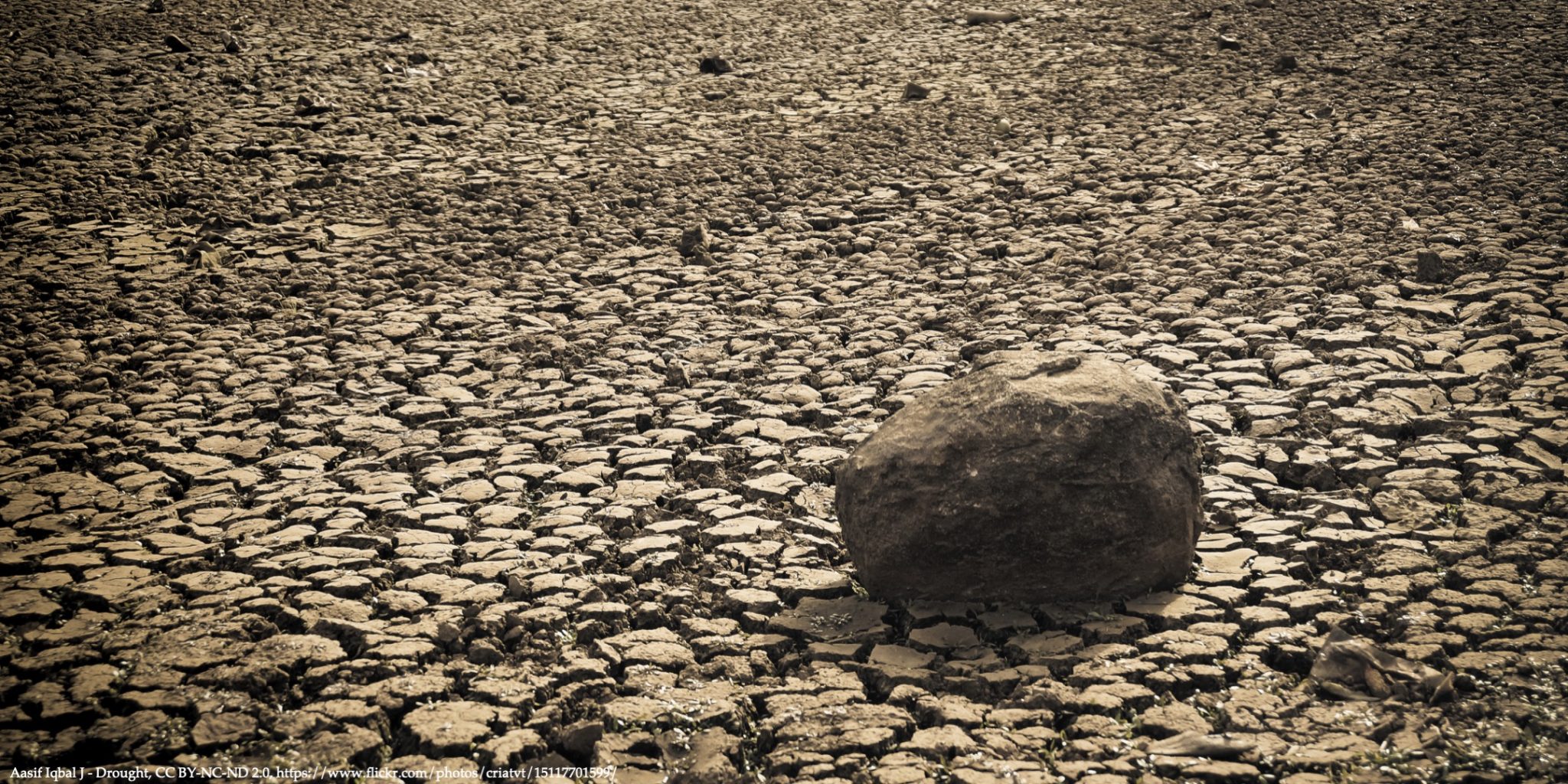
In November 2018, Viktor Kondrashin, expert historian on the Soviet famine in the early 1930s, organized a workshop at the Institute for Russian History of the Academy of Sciences (IRI RAN). Contributions by Russian and Ukrainian as well as British, Irish and Swedish historians summed up their research projects concerning the famines in Russia, Kazakhstan and Ukraine in 1929–1933. They highlighted the opposing interpretations of the causes of these famine-stricken regions. They also scrutinized the recent debates in the West of Anne Applebaum’s book Red Famine. Motivated by this enlightening discussion, this brief offers a short overview of the history, and contemporary state, of the Soviet famine debate.
In this brief, my purpose is to describe research frontiers concerning the Soviet famine in the early 1930s, based on my participation in the workshop at the Institute of Russian History of the Academy of Sciences in November 2018. Starting with a short historiographical background, I give examples of important archival contributions. Then I present contributions by individual scholars and their interpretations, concerning the causes of the 1929–33 famines in Soviet Russia and Ukraine. The separate famine in Kazakhstan 1929–30 was caused by forced sedentarisation and disbanding of the Kazakh people’s nomadic life; it claimed a proportionally higher number of deaths but would require quite a different analysis.
The collectivization of the peasantry was enforced in 1930–31. An initial abundant harvest 1930 seemed as a success for the kolkhoz system. It appeared to supply enough food for city dwellers and industrial workers as well as Red army soldiers. It also seemed to permit a large export of grain. This, and raw material exports in general, was crucial for the Soviet Union as hard currency generators for the import of machinery from the West. The five-year plan 1928–32 had overestimated how much these export commodities would generate. During the Great Depression, terms of trade turned dramatically against the Soviet export commodities. Likewise, the Soviet leadership had not foreseen how intensively collectivization would be resisted by peasants. Not only were many farming processes neglected in the new kolkhozes. Many peasants chose to slaughter horses, rather than giving them to the kolkhozy. The production of tractors could not yet substitute the loss of draught animals by ca. 15 per cent in 1930–31.
Weather conditions worsened and resulted in lower harvests in 1931 and 1932. A severe famine was imminent. Grain exports were cut. Rationing was severed. Although grain collection quotas were lowered in 1932, the greater famine severely hit the grain-producing regions in Ukraine, but also Kuban and Lower Volga regions and Northern Caucasus. The overall Soviet population losses by 1933 are estimated as 6–7 million, with approximately 3.5 million famine-related deaths in Ukraine.
This famine was a taboo topic in the Soviet era. The authorities managed to divert attention from the catastrophic consequences of collectivization and rapid industrialization. After World War Two numerous oral witnesses’ collections were gathered in the Ukrainian diaspora in Canada. In the late 1980s, the British historian Robert Conquest wrote his pioneering study Harvest of Sorrow. An accompanying TV documentary film made the general public in the West aware of the 1930s famine in the USSR.
Before that, during the “Thaw” in the early 1960s, established Russian historians had got Nikita Khrushchev’s directive to study primary sources concerning Stalin’s forced collectivization. However, their dramatic findings and frank book manuscript on the real costs of the collectivization was prohibited in 1965 by Khrushchev’s successors. Only during glasnost could these specialists, with Viktor Danilov (1925 – 2004) as one of the most visionary researchers, again analyze the agrarian history of the Soviet Union in the late 1920s and early 1930s.
Danilov’s primary concern was to make as many archival documents as possible available. He guided archivists and researchers to examine all relevant, central as well as regional, archives. Numerous documentary volumes, articles and monographs were produced, notably the 5-volume project The Tragedy of the Soviet Countryside, which showed both the excesses of the forced collectivization in 1930-33, and the famine conditions all over the USSR. Danilov also cooperated with other archivists to compile a documentary of how the secret police’s local officers in the countryside – often more accurately and frankly the communist party organs – did indeed describe the real situation in the villages. This project The Soviet Countryside as seen by VChK-OGPU-NKVD covers the whole interwar period 1918–39, and added unexpected perspectives on the dramatic period. In particular, it proves – contrary to what is sometimes believed – that Stalin and the leadership were very well informed of the dire situation that resulted from their policy decisions (see Samuelson 2007).
Today, we have not only oral history – eye-witnesses and relatives of the famine victims – but also a sufficiently reliable source base to describe all aspects of the transformation in the countryside. What remains however, is to set all this new empirical material into its context and to explain what caused the famine years 1929–1933? Which were its specific traits in different parts of the Soviet Union?
A most authoritative Russian expert on these topics is Viktor Kondrashin. He has continued the projects started by Danilov and published several archival documentary collections. The first is Famine in the USSR 1930 – 1934 with almost 200 facsimile archival documents. A larger archival publication by Kondrashin in four volumes (2011–13) expands the empirical basis for researchers. Of particular interest are those on the famine in various parts of the USSR. Kondrashin’s recent monograph The 1932-1933 Famine: The Tragedy of the Russian Countryside (2018) outlines his interpretation that the grand famine was the unexpected result of the collectivization. It struck in many regions of the Russian republic. It was not directed specifically against any ethnic group. Grain collection from the kolkhozes in 1931 and 1932 was brutally enforced. The Soviet leadership lacked information in 1932 concerning the real harvest. Only when the famine grew catastrophic in the winter of 1932/33, did the authorities change their policies: lowered grain requisition quotas, sent seed grain back to many regions, tried to evacuate suffering children from famine-struck regions. In short, that millions of people in Soviet Ukraine as well as in Russia died during the famine years was an unforeseen effect of a policy. In other words, it was not an intentional, genocidal policy directed towards the Ukrainian or any other people but a tragedy for all the peoples of the USSR.
In the West, major research on Soviet agriculture was done by Robert Davies and Stephen Wheatcroft. Their monograph The Years of Hunger (2004) is translated into Russian. It provides empirical data on all agricultural branches. Their conclusion is that “the Soviet leadership was struggling with a famine crisis which had been caused by their wrongheaded policies, but was unexpected and undesirable”. Davies and Wheatcroft emphasize that the changing policies in 1932/33 (lowering of grain collection, redistribution back to the peasant and shelter for famine-stricken youngsters) indicate that there was not any intention to “apply famine as a terror-weapon” as Conquest and many Ukrainian historians have stated. Stalin and other leaders made concessions to Ukraine in procurements, and were clearly trying to balance the subsistence needs of Ukraine and other regions. They cut down grain exports in 1932 to a minimum. But they did not acknowledge the famine to the West, asking for relief as Lenin had done in 1921–22, for fear of losing further in credibility worth. Also important to consider is that the Soviet leadership was particularly worried over a possible further Japanese expansion towards the Soviet Far East, after the takeover of Manchuria in 1931-32. This factor precluded any more use of the state reserves of grain (for the armed forces in case of war), to alleviate the situation in the starving areas.
Since the late 1990s, the Ukrainian historians have made different interpretations. The general famine all over the USSR is acknowledged as a consequence of Stalin’s policy. The climate as well as crop diseases led to shrinking harvests in 1931 and 1932. However, the famine that struck Ukraine in 1932/33 was essentially different. It is called Holodomor from holod – famine and morit’ – to kill. This interpretation presumes a genocidal intention of the Soviet leadership against the Ukrainian people, and its peasantry in particular. Since 2006, this interpretation is state law in the Ukraine and stresses that Holodomor was deliberately planned and executed by the Soviet regime in order to systematically destroy the Ukrainian people’s aspirations for a free and independent Ukraine, and subsequently caused the death of millions of Ukrainians in 1932 and 1933. The Ukrainian Rada adopted the law Pro Golodomor 1932 – 1933 rokiv v Ukraïni (Закон України: Про Голодомор 1932–1933 років в Україні) on 28 November 2006, which consequently set out the exact juridical terms that give the established framework for historiography in the Ukraine.
It is testimony of the high standards in the Russian academic tradition that Kondrashin invited the prominent Ukrainian historian Stanislav Kulchitskii and the Canadian-based Roman Serbyn, and others from Ukraine, to contribute to the anthology The Contemporary Russian-Ukrainian Historiography on the Famine 1932 – 1933 in the USSR. Here the reader himself can study and compare their argumentation for the Holodomor-interpretation. They present a different set of empirical data from each theme in this ongoing debate, concerning both agricultural and demographic figures, as well as the Soviet Communist party’s decisions versus Ukraine in late 1932 – early 1933.
The decisive question accordingly remains which of the rival interpretations is most solidly confirmed by traditional methods on history as science. In other words, whether there was an intention from Stalin, from central and/or regional leaders, to cause or to aggravate – as a terror-instrument – the famine in the Ukraine. In addition, it remains to be decided whether or not the concept of genocide, as defined in the 1948 United Nations Convention, is applicable to the famine in the Ukraine and other parts of the Soviet Union. The genocide interpretation is rooted in an academic tradition that stretches from Conquest’s above-mentioned Harvest of Sorrow to more recent works, such as by Timothy Snyder Bloodlands: Europe between Hitler and Stalin, Norman Naimark Stalin’s Genocides and numerous articles by Andrea Graziosi among other historians in the West.
Mention should finally be done of Anne Applebaum’s Red Famine: Stalin’s War on Ukraine (2017) as a most detailed and vivid description of the sufferings of the Ukraine people in the early 1930s. Her book was very positively reviewed not only in the mass media, but also by academic scholars like Sheila Fitzpatrick. However, Fitzpatrick had obviously misread the book and praised it for its refutation of the genocide interpretation. This forced Applebaum to clarify that precisely the opposite was her case: The famine in the Ukraine was intentional and foreseeable by the Soviet leadership, whose intention it was to subdue nationalist aspirations. In turn, this rejoinder forced Fitzpatrick in a commentary to withdraw all her praise for the book!
Given this hot debate, it is not surprising that, in the academic community, it was felt that matters could not be put to rest without thorough arguments. The editors of Contemporary European History organized a solid roundtable on Soviet famines, with written essays by leading historians and specialists (Volume 27, Issue 3, August 2018, pp. 432-434). These concise essays, by N. Naimark, N. Pianciola, T. Penter, J.A. Getty, A. Graziosi, S. Wheatcroft and others may serve as the best introduction to a thorny historical theme that can otherwise be difficult to grasp, not least because of the often politicized nature of the debates.
The autumn 2018 seminar at IRI RAN in Moscow was one in a long row of similar events – conferences and workshops on the history of the collectivization of the peasantry, the dekulakization and repressions against peasant protests, and the famines in various Soviet republics in 1929 – 1934. Already in spring 2004, a landmark conference took place at IRI RAN, where Viktor Danilov and Stanislav Kulchitskii, as representatives of the Russian vs. the Ukrainian perspective, debated for a whole day (!), albeit without converging viewpoints. At that stage, they could sum up a decade of archival research and chisel out divergent points for further research. Over the last fifteen years, scholars in both countries have made great strides to deepen the empirical foundation, notably by detailed mapping of the harvests, demographic changes and other indicators even at the local level. This new level of knowledge was well reflected in the various contributions at the seminar and in the above-mentioned anthology Contemporary Russian-Ukrainian Historiography.
References
- Applebaum, Anne, Red Famine: Stalin’s War on Ukraine, New York: Doubleday, 2017.
- Applebaum, Anne, Reply to Sheila Fitzpatrick’s review of Red Famine, Facebook: https://www.facebook.com/anneapplebaumwp/posts/as-an-author-who-also-writes-reviews-i-generally-try-to-avoid-responding-to-revi/704110623118513/
- Conquest, R., The Harvest of Sorrow: Soviet Collectivization and the Terror-Famine, Oxford University Press, 1986.
- Danilov, V.P. et altere, eds., Tragediia Sovetskoi Derevni: Kollektivizatsiia i raskulachivanie, 1927 – 1939, (The Tragedy of the Soviet countryside: Collectivization and dekulakization), five volumes, Moscow: Rosspen, 1999–2004.
- Danilov, V.P. et altere, eds. Sovetskaia derevnia glazami VChK-OGPU-NKVD, 1918 –1939, (The Soviet countryside as seen by VChK-OGPU-NKVD, 1918–1939), four volumes, Moscow: Rosspen, 1998–2005.
- Davies, R.W. & Wheatcroft, S.G., The Years of Hunger: Soviet Agriculture, 1931–1933, Palgrave Macmillan, 2004; also as translation: Gody goloda: Sovetskoe selskoe khoziastvo, 1931–1933, Moscow: Rosspen, 2011.
- Fitzpatrick, Sheila, “Red Famine by Anne Applebaum review – did Stalin deliberately let Ukraine starve?”, The Guardian, 25 August 2017, see 2019-03-26: https://www.theguardian.com/books/2017/aug/25/red-famine-stalins-war-on-ukraine-anne-applebaum-review
- Golod v SSSR 1929 – 1934, (The Famine in the USSR, 1929–1934), Moscow: Mezhdunarodnyi Fond Demokratiia, 2011–2013.
- Kondrashin, V.V. ed., Sovremennaia rossiisko-ukrainskaia istoriografiia goloda 1932–1933 gg. v. SSSR, (The Contemporary Russian-Ukrainian historiography of the famine 1932–1933 in the USSR), Moscow: Rosspen, 2011.
- Kondrashin, V.V., Golod 1932–1933 godov: Tragediia rossiiskoi derevni, (The Famine in 1932–1933: The Tragedy of the Russian countryside), Moscow: Rosspen, 2018.
- Kondrasjin, V.V., “Hungersnöden i Ryssland och Ukraina 1932–33, in Samuelson, L. (ed.), Bönder och bolsjeviker: Den ryska landsbygdens historia 1902–1939, EFI/HHS 2007, p. 140 – 173.
- Kozlov, V.P., Golod v SSSR 1930–1934//Famine in the USSR 1930–1934, Moscow: Federalnoe Arkhivnoe Agentstvo, 2009.
- Kulchitskii, S.V., “Obshchii i regionalnyi podkody k istorii velikoi tragedii narodov Rossii i Ukrainy”, (The General and regional approach to the great tragedy of the peoples of Russia and Ukraine), in Sovremennaia…istoriografiia, (above), pp. 107 – 206.
- ”Roundtable on Soviet Famines”, Contemporary European History, vol. 27: 3, August 2018, see: https://doi.org/10.1017/S0960777318000279
- Samuelson, L., “Gammal och ny historieskrivning om den sovjetiska landsbygden – “Arkivrevolutionens betydelse sedan 1990-talet”, in Bönder och bolsjeviker: Den ryska landsbygdens historia 1902–1939, EFI/HHS, 2007, p. 26–41.
Disclaimer: Opinions expressed in policy briefs and other publications are those of the authors; they do not necessarily reflect those of the FREE Network and its research institutes.
Economic Growth and Putin’s Approval Ratings —The Return of the Fridge
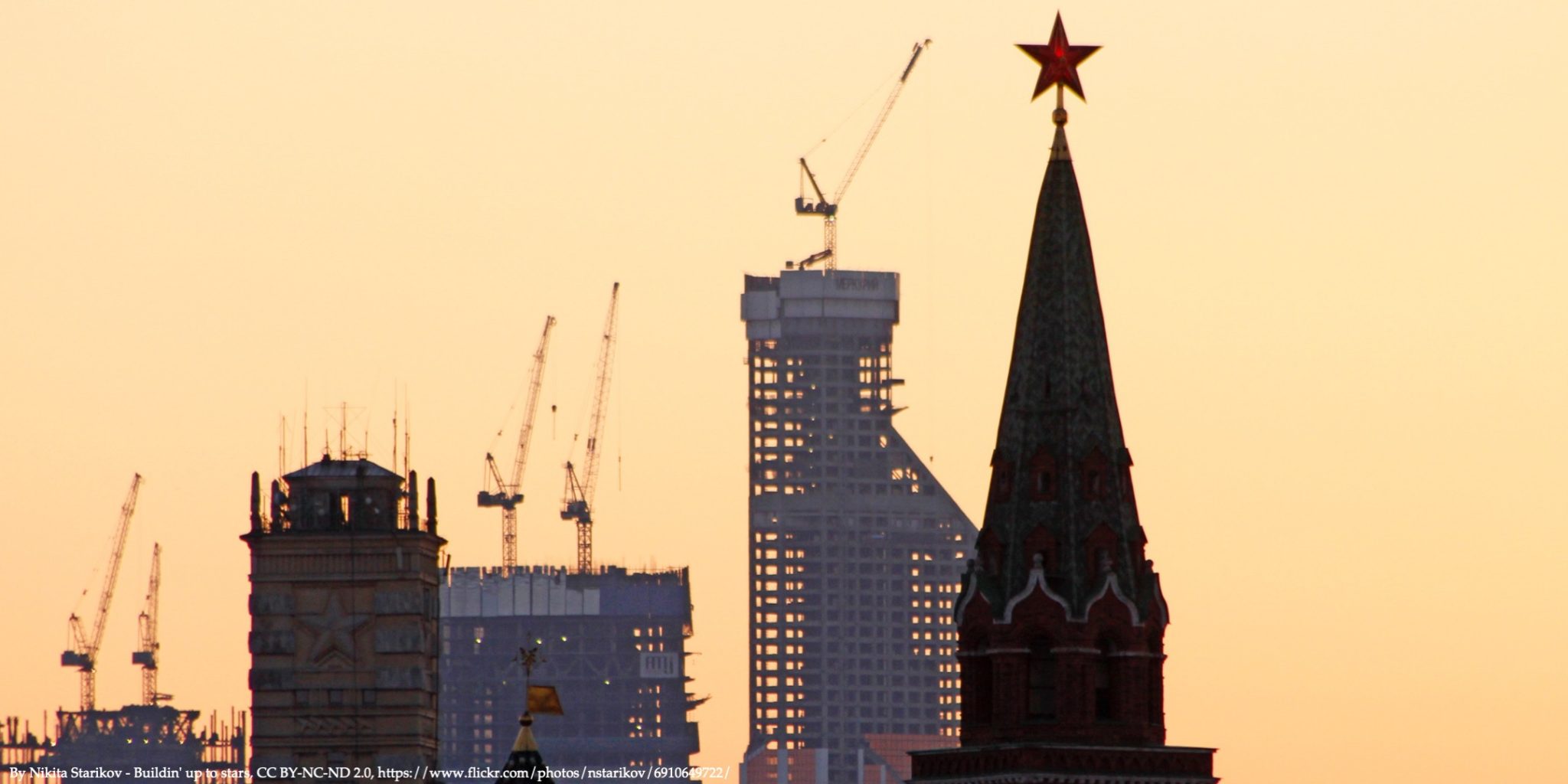
This brief discusses how the approval ratings of president Putin covaries with economic growth. In most years the relationship between approval ratings for Putin and growth looks like approval ratings for politicians in most countries so that when growth is higher, the president is more popular. Or to use an American expression “it’s the economy stupid”. The caveat in Russia is that external events at times overshadow the importance of growth to the extent that the president’s ratings stay high and can even go up despite a faltering economy. In a time of low Russian growth, this is not good news for geopolitics unless Putin can be convinced to focus on policies that generate high, sustainable growth instead of international turbulence. That said, it is clear that poor economic growth carries a political cost also in Russia. The only sustainable way of maintaining high approval ratings for the president is by fostering economic growth since in the contest between “the TV and the fridge”, the fridge will eventually win.
Russia is a complex country and culture. Instead of the simple American saying “it’s the economy stupid”, Russians talk about “the TV vs the fridge”. This translates into that concerns about the economic situation can be made irrelevant by propaganda so that voters turn their eyes away from the half-empty fridge to follow how Russia’s armed forces fight the enemy in foreign countries.
The propaganda messages have of course varied over the years, but it seems that external enemies that threaten the nation are at the heart of many of the messages. This theme in propaganda is of course not unique to Russia, but it seems to carry more weight in Russia than in other countries.
The observation that propaganda is used and that it seems to work to a relatively large extent at times can lead to the conclusion that “it’s not the economy stupid” when it comes to approval ratings of the Russian leadership.
This observation is tempting as another piece of evidence on how Russia is different and unique, but this brief will show that in most times, it is indeed “the economy stupid” also in Russia.
Putin’s ratings and growth
The idea that Russia is different in that growth would not be important for the president’s approval rating can be justified empirically when we look at the full series of approval ratings of Putin as measured by the Levada center and corresponding quarterly growth rates going back to 1999 (Figure 1).
Instead of showing a strong positive correlation as we would expect, the correlation is negative 0.3. However, a more careful look at the observations in the scatter plot suggests that there are a few clusters of observations that create this negative correlation. In the figure, three distinct clusters are marked; first there is the period when Russia rebounded strongly from the 1998 crisis in 1999-2000, with growth rates that have not been seen before or after that time in Russia. The growth was an artefact of the previous massive decline in income in combination with a large devaluation, and later followed by oil price increases. This happened in Putin’s initial time in the highest offices when he was prime minister, interim president and then elected president. Although Putin enjoyed high ratings as a consequence, it was not in line with the extreme growth rates that were the result of events preceding his tenure and can thus be regarded as outliers.
The second cluster is related to the global financial crisis in 2008/09 when Russian growth took a major hit as oil prices collapsed and the exchange rate was not allowed to appreciate correspondingly. However, this crisis was blamed (as in many other countries) on the US and the West and did not cost Putin in terms of approval ratings.
The final cluster is related to the annexation of Crimea and ongoing involvement in the conflict in Eastern Ukraine. This period also coincides with a sharp drop in oil prices that taken together led to negative growth that then remained low for a prolonged period. We should note that before the annexation of Crimea, growth rates in 2013 were very low at 1-2 percent with approval ratings going down to 63 percent, which was an all-time low since Putin’s first year in office.
Figure 1. Ratings and growth
Source: Becker (2019)
If we purge the data from the three exceptional episodes that we have identified above, we get Figure 2. Note that the scale has not been changed from Figure 1. Now there are no observations of negative growth rates, but the distribution of growth rates is still rather spread out, going from around 1 to 9 percent growth. The spread of the growth distribution is important since it allows us to identify the relationship between growth and approval ratings more clearly.
The relationship between approval ratings and growth in Figure 2 is strongly positive with a correlation coefficient of 0.7, and in line with what we would expect in other countries. This is a quite remarkable shift from the negative correlation in Figure 1. Note that if approval ratings in 2014 had been behaving as in “normal” years, the regression line would have put them around 60 percent instead of the actual approval rating that peaked at 86 percent after the annexation of Crimea. Such is the strength of the TV.
Figure 2. Ratings and growth
Source: Becker (2019)
This is very clear evidence that Russia is a “normal” country in “normal” times, but that there are also times when other forces overshadow this normalcy.
Policy conclusions
Are there any policy conclusions that can be drawn from the stark contrast between figures 1 and 2? The answer is a very clear “yes”, both for the Russian leadership but also for the rest of the world that has economic interests and security concerns with Russia.
For the Russian president, the message is that it pays in terms of high approval ratings to generate growth and “keeping the fridge well stocked”. It is also clear that the high popularity rating that was seen after the annexation of Crimea has been followed by several years of poor growth. A forthcoming brief discusses how the increased uncertainty created by this event has led to lower capital inflows, lower domestic investments and lower growth.
Not surprisingly, the sustained low growth has started to show in terms of falling approval ratings. The polls at the end of 2018 and early 2019 (for when there is not yet data on growth rates) indicate a significant decline in approval ratings, down to 64 percent from over 80 percent at the end of 2017. This is linked to protests over pension reforms, but they in turn are a result of lower government revenues in an economy that lacks growth.
In other words, if growth does not return before the propaganda loses its appeal, this will eventually result in falling approval ratings for the president, which is what we are seeing now.
There are potentially also some policy conclusions for Russia’s foreign investors, trading partners and neighbors. When growth in Russia is low and no credible reform programs are on the horizon, expect external actions that take the attention away from poor economic performance while increasing the level of uncertainty both in Russia and abroad.
For the more pro-active external actors, finding ways to support Russia’s return to growth through dialogue on real economic reforms could perhaps be both politically feasible and of mutual interest to Russia and the West. There are clearly some geopolitical issues that may interfere with this process, but it should still remain high on the wish list of regular people in Russia and elsewhere. Let the fridge rule!
References
- Becker, T., 2019. “Russia’s macroeconomy—a closer look at growth, investment, and uncertainty”, forthcoming SITE Working Paper.
Disclaimer: Opinions expressed in policy briefs and other publications are those of the authors; they do not necessarily reflect those of the FREE Network and its research institutes.
US-China Trade War of 2018 and Its Consequences
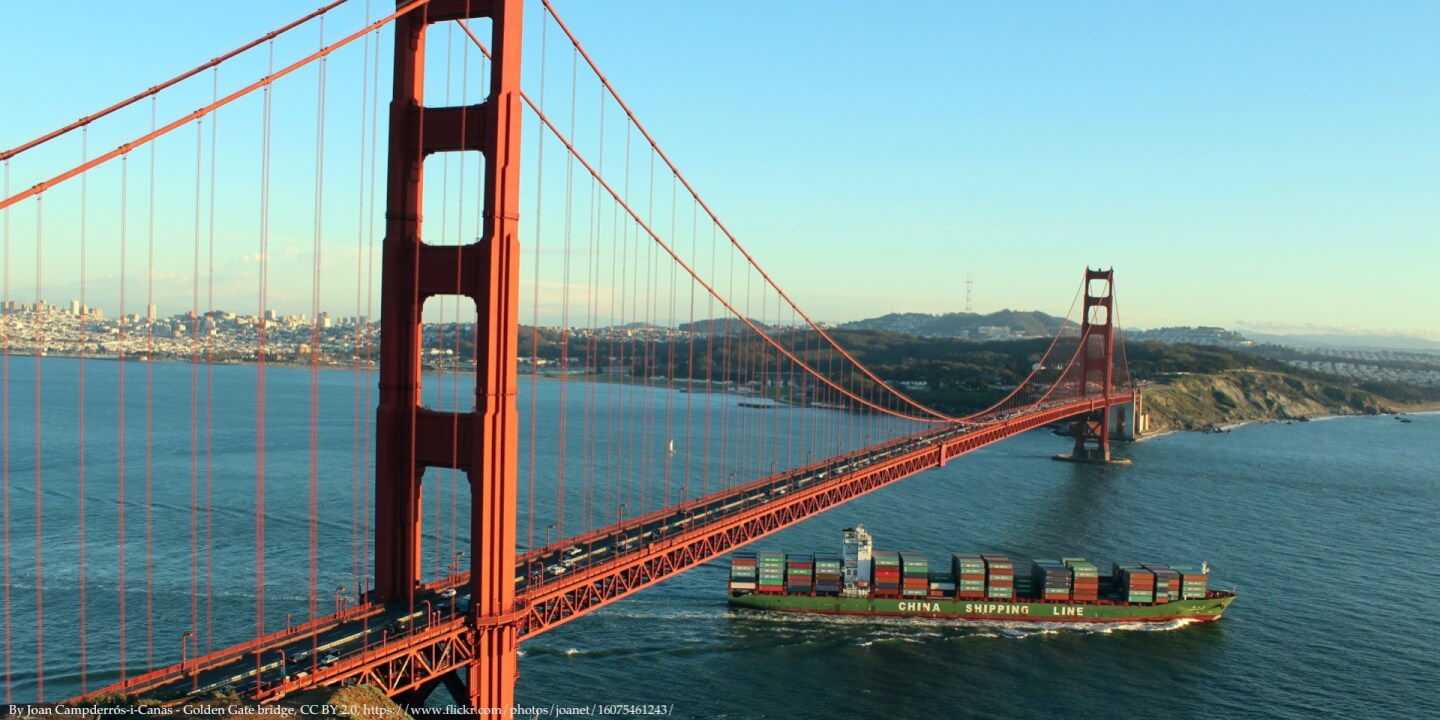
The trade war between the United States and China has become one of the main events in the global economy this year. What could be its consequences for the US and China, and how might it affect other countries – for example, Russia?
Chronology of the trade war
Donald Trump started the war, raising import tariffs on solar panels in January 2018, of which the main supplier is China. In response, on April 2nd, China raised import duties on 128 commodities originating from the United States. On July 6th, the US increased tariffs on Chinese goods by 25 pp., imports worth $34 billion. China responded symmetrically. In August, the United States increased the tariffs on another $16 billion of imported goods from China, to which a symmetrical response again followed. In September, the United States again applied higher tariffs for $200 billion of Chinese exports, and China for $60 billion of US exports. At each stage of the conflict escalation, China appealed to the WTO with complaints about the actions of the United States, pointing to the inconsistency of their actions with the obligations and principles of the WTO. There were several meetings of official representatives from the United States and China – without any significant results.
What are the main reasons for this unprecedented escalation?
Imbalance and intellectual property
The economies of the US and China today are by far the largest in the world, and the trade turnover between the two countries is one of the most important. A remarkable feature of these trade flows over last decades is their imbalance. In 2017, the United States imported $526 billion worth of goods from China, while China’s imports from the United States amounted to $154 billion. Part of this imbalance is offset by trade in services, but it is not enough to even it out: in the same the year the United States delivered $57 billion worth of services to China while importing services of $17 billion from China.
Experts have different views on this imbalance. On the one hand, there is a perception that it is a source of world economy vulnerability, a source of potential crisis. Therefore, it is necessary to reduce the trade deficit. Another point of view is that this imbalance merely reflects the fact that the US economy and its assets are very attractive to investors from all over the world, including Chinese – and that, in turn, requires that the surplus of capital flows biased to US side, was compensated by the corresponding deficit of trade in goods and services. One such investor is the Chinese state itself, which for many years has been pursuing a policy of exchange rate undervaluation in order to promote foreign trade. It led to an enormous accumulation of foreign exchange reserves and as of January 2018, China held $1.17 trillion of US bonds and was the largest creditor of US government.
US President Donald Trump referred to this trade imbalance as one of the reasons for the outbreak of this trade war against China. Trump aims at reducing the deficit by $100 billion from the current $375 billion. The unilateral increase in import tariffs applied to Chinese goods was the first action of the US administration in this direction.
The second, no less important, formal reason for the trade war is the inadequate protection of intellectual property rights in China. China’s production of counterfeit products, the lack of adequate practices and laws to protect foreign technologies from illegal dissemination in the country, is not news to anyone. And although the almost two decades since China’s WTO accession have meant a largely modernized legal framework in this regard, a number of important provisions are still inconsistent with international practices, and the implementation of existing intellectual property rights leaves much to be desired. Established in 2012, The Commission on the Theft of American Intellectual Property identifies China as the most malicious violator of US rights. The exact damage is not known, but the commission assessment of the losses to the American economy due to the forced transfer of technology to Chinese partners – which is an unspoken condition of foreign manufacturers access to the Chinese market – industrial espionage, contradictions in legislation, requirements for the storage of sensitive data in China are in the range from $225 to $600 billion per year (Office of US Trade Representative, 2018).
While both the trade deficit and the intellectual property rights issue were recognized for many years, it was in 2018 that Trump started acting on them. Therefore, in order to discuss the potential impact of the conflict between the world’s largest economies on themselves and other economies, such as Russia, it is important to understand what drives the actions undertaken by Trump’s administration.
Populism
Trump won the elections in 2016 with a minimum margin against the Democratic rival. To provide support for his decisions and to increase the chances of being reelected for the next term in 2020, it is crucial to maximize the pool of his supporters. Trade policy measures aimed at import substitution are very effective populist policies in any country. One of the first steps made by the US toward trade war was the increase in import tariffs on steel and aluminum – for all countries. Metallurgy and coal industries are among the most organized and strong lobbyists in any country. The European Union as an economic organization started with the European Coal and Steel Association. By aligning interests with these sectors much can be achieved in relation to trade liberalization, and vice versa – by increasing the level of protectionism, a significant popularity increase can be among voters whose incomes depend on the success of companies in these industries.
Deterrence
China works hard raising the technological level of its economy. In recent years the Chinese government and Communist party launched a number of ambitious programs aimed at achieving a technological breakthrough, lessening the dependence on imported technologies by substituting them with ones produced by domestic innovation centers. These programs specify the priority sectors, in which state subsidies are provided for the acquisition of foreign technologies by Chinese companies and their adaptation. One of the common arguments was that the United States believes that powerful state support for technology sectors in China, along with the existing problems in protecting intellectual property rights, increases the risks and potential losses of American companies.
However, while these concerns seem reasonable at first, they should not be taken at the face value.
China’s ability to push out American companies in the high-tech sector on the world market seems rather limited. So far, China has only succeeded in increasing its share in the middle and low technology segments. Instead, in recent years, China is rapidly increasing its defense spending, which in 2017, for the first time, reached a level of 1 trillion yuan (about $150 billion). China’s defense spending is the second highest in the world after the United States. Moreover, it’s growing very fast. While in 2005 the Chinese nominal defense expenses were only 10% of American expenses, in 2018 they are already around 40%. The dominance of state enterprises in the defense industry in China implies that the real purchasing value of these expenditures is quite comparable. New and existing Chinese industrial policy programs target military and dual-use industries among others. Therefore whilst addressing the intellectual property rights problem in China now, Trump’s administration also aims at preserving US leadership position in the military sector, which finds widespread support in Trump’s main voter groups among Republicans.
Obsolete weapon
Historically, trade wars implied tariff escalations to protect domestic industries from foreign competition. Today, the Trump administration behaves in a similar manner. However, the circumstances now are fundamentally different from those in the first half of 20th century and earlier. Firms not only trade in final goods, but more and more they trade in intermediate products and within firms themselves (Baldwin, 2012). The distribution of the production process to many companies across different countries of the world leads to two important effects, which were not observed in previous trade wars.
First, it is the effect of the escalation of tariff protection in the framework of the value chains. The import tariff is applied to the gross value of the product crossing the customs border. However, the exporting firm’s contribution to the gross value might be quite small. So the effective level of the tariff will be higher than the nominal level of the tariff, known as a so called amplification effect (World Bank, 2017, page 98). It means that the effective growth of the tariff by 25 percentage points in relation to Chinese imports will significantly exceed 25 % and in some cases can even become prohibitive. So, the tariff warfare will result in significantly greater losses for the sectors involved in the value chains, compared to the sectors less exposed to them. It means that foreign investors and multinational companies in China will suffer bigger losses compared to purely domestic Chinese companies. The Peterson Institute for International Economics made an assessment and confirmed these observations (Lovely and Yang, 2018).
Second, China’s participation in international multinational companies most often occurs in the assembly segments, while developed countries’ companies contribute at other stages, such as with innovation, design, financial and consulting services, marketing, and after-sales services. Then, the protectionist measures against goods produced in China by multinational companies will hit an American economy, generating losses in the service segments. A similar episode happened, for example, in 2006, when the European Union introduced anti-dumping duties on imported footwear from China and Vietnam, which in turn lead to a decline in the services sector in Europe – imported footwear contained a significant share of the value added created by European designers and distributors (World Bank, 2017). Obviously, we will observe the same consequences in the United States now, since the role of the American services sector in creating and promoting Chinese goods on the American market is significant and according to World Bank estimates in 2011, the contribution of value added generated by foreign services in China’s gross exports amounted to about 15% (World Bank, 2017).
Thus, not only the economy of China, but also the US economy itself will suffer from the growth of import tariffs in the USA. The USA is not an exception here – the governments of most countries continue to live in the paradigm of trade policy, which suits the structure of the world trade as at the beginning of the 20th century, while trade has gone far ahead and requires much more elaborate effective regulatory tools than tariffs on imported goods.
Consequences for Russia
The consequences of the US trade war with China for the Russian economy depend on what the main goals of the war are. If the motive is primarily electoral – to secure enough support in 2020, one can expect that the protective measures will be short-lived, and the geographical distribution of investment flows will remain almost intact and that China will remain an important location for global value chains transactions. The trade war will in this case lead to some economic slowdown in the short term. The main effects will be related to the redistribution of income within economies, where protected sectors will benefit on the expense of all other sectors. In these circumstances, Russia would suffer direct losses from the growth of tariffs on their exports to US (now it is predominantly steel and aluminum), but for the economy as a whole, the losses will not be significant, especially relative to the losses Russia bears because of sanctions.
However, if the main reason for the trade war has a long-term perspective, the investors will be forced to adjust the geography of their investment plans and China will face a significant outflow of foreign investments, which will significantly affect Chinese – and global – economic growth. In this case, both for Russia and for the whole world, the indirect effect of the US-Chinese trade conflict will be quite noticeable and it will take years to create new trade links and restore world trade and global value chains.
References
- Baldwin, Richard, 2012. “Global supply chains: why they emerged, why they matter, and where they are going”, CTEI Working papers 2012-13, The Graduate Institute, Geneve
- Lovely, Mary E., and Liang Yang, 2018. “Revised Tariffs Against China Hit Chinese Non-Supply Chains Even Harder.” PIIE Policy brief, Peterson Institute
- Office of the US Trade Representative. March 22, 2018. “Executive office of the President findings of the investigation into China’s acts, policies, and practices related to technology transfer, intellectual property, and innovation under section 301 of the trade act of 1974.” https://ustr.gov/sites/default/files/Section%20301%20FINAL.PDF
- World Bank, 2017. “Measuring and analyzing the impact of GVCs on economic development”. World Bank, Washington DC.
Note
A longer version of this brief has been published in Russian by Republic: https://republic.ru/posts/92217
Disclaimer: Opinions expressed in policy briefs and other publications are those of the authors; they do not necessarily reflect those of the FREE Network and its research institutes.
Energy Demand Management: Insights from Behavioral Economics
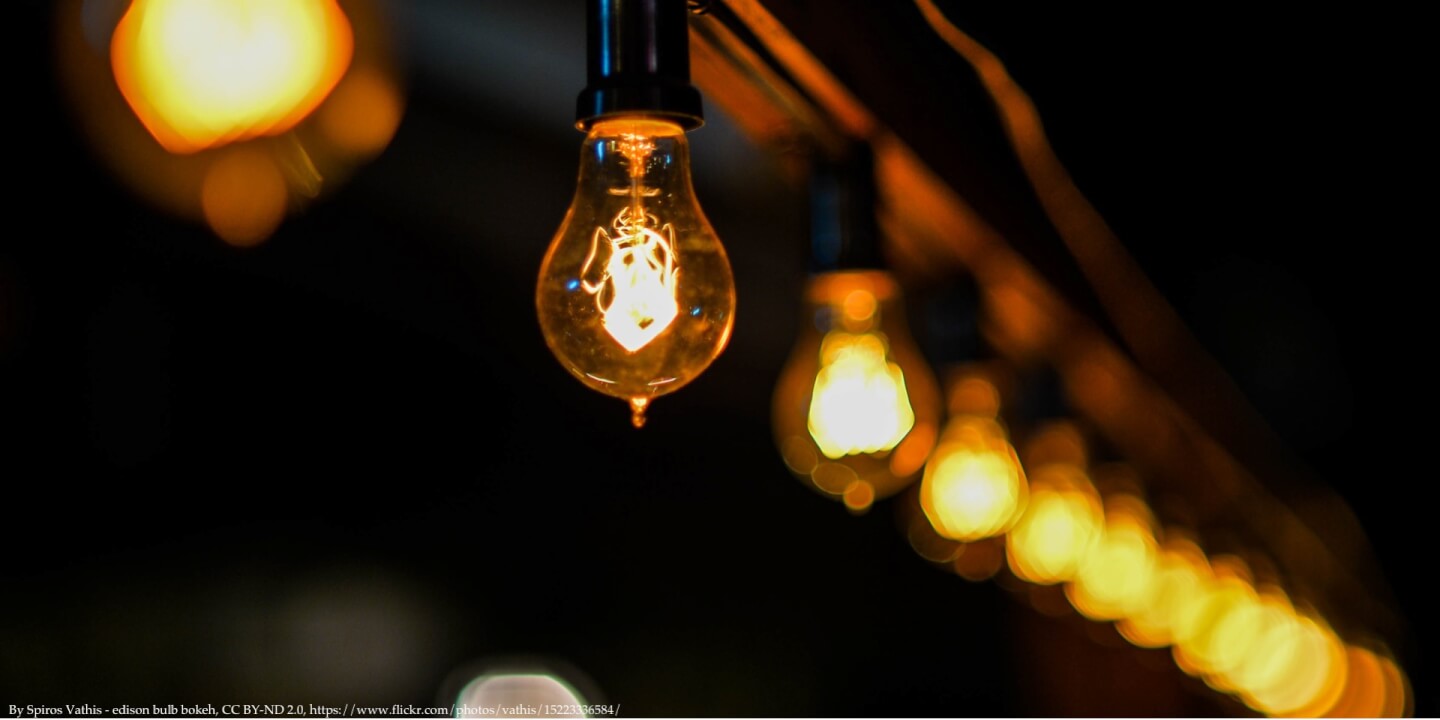
It has long been recognized that consumers fail to choose the cheapest and most efficient energy-consuming investments due to a range of market and non-market failures. This has become known as the ‘Energy Efficiency Gap’. However, there is currently a growing interest in terms of understanding on how consumers make decisions that involve an energy consumption component, and whether the efficiency of their decisions can be improved by changing the market incentives and governmental regulation. Meeting this interest, the most recent SITE Energy Talk was devoted to Demand Side Management. SITE invited Eleanor Denny, Associate Professor of Economics at Trinity College Dublin, and Natalya Volchkova, Assistant Professor at the New Economic School (NES) in Moscwo and Policy Director at the Center for Economic and Financial Research (CEFIR) to discuss the Demand Side Management process. The aim of this brief is to present the principles of Demand Side Management and discuss a few implemented programs in Europe, based on the discussions during this SITE Energy Talk.
For the last two decades, climate change policies have mostly been focused on the energy supply side, constantly encouraging new investments in renewables. But reducing energy demand may be as effective. Indeed, Denny and O’Malley (2010) found that investing 100MW in wind power is equivalent, in terms of emissions, to a decrease in demand of 50MW. Hence, there is a clear benefit of promoting energy saving. This has been the central point of different Demand Side Management (DSM) programs that may diversely focus on building management systems, demand response programs, dynamic pricing, energy storage systems, interruptible load programs and temporary use of renewable energy. The goal of these programs is to lower energy demand or, at least, smoothen the electricity demand over the day (i.e. remove peak-hour segments of demand to off-peak hours) as illustrated in Figure 1.
Figure 1 – Smoothing electricity demand during the day
A behavioral framework
DSM encompasses initiatives, technologies and installations that encourage energy users to optimize their consumption. However, the task does not seem easy, given the well-documented energy efficiency gap problem (e.g. Allcott & Greenstone, 2012 or Frederiks et al., 2015): consumers do not always choose the most energy efficient investments, despite potential monetary saving. One reason why might be that energy savings per se are not enough to trigger investment in energy efficient solutions or products. As Denny mentioned in her presentation, consumers will invest when the total private benefits are higher than the costs of investment. This trade-off can be summarized by the following equation:
This equation illustrates that any DSM design should take into account both non-monetary benefits and consumers’ time preferences. The non-monetary benefits, such as improved comfort, construction and installation time, but also warm glow (i.e. positive feeling of doing something good) or social comparison, may play a major role. Moreover, the consumers’ time preferences (reflected here by the discount rate ) are also crucial in the adoption of energy efficient products. In particular, if consumers have present biased preferences, they would rather choose a product with a lower cost today and greater future cost than the reverse (i.e. higher cost today with lower future cost). Since energy-efficient products often require higher upfront investment, consumers that are impatient for immediate gains, may never choose energy efficient products.
Ultimately, it is an empirical (and context specific) question when and why DSM programs can reduce the energy efficiency gap. We describe below some DSM programs that have been implemented and discuss their impact.
Smart meters, a powerful DSM tool
A common DSM program is the installation of smart meters, which measure consumption and can automatically regulate it. The adoption of smart meters allows real-time consumption measures, unlike traditional meters that only permitted load profiling (i.e. periodic information of the customer’s electricity use).
Figure 2 – Energy Intensity in Europe
As illustrated in Figure 2, many European countries have implemented smart meter deployment programs. Interestingly, most of those countries have a relatively high level of energy efficiency (proxied by the energy intensity indicator of final energy consumption). On the contrary, in the Balkans and non-EU Eastern Europe countries, which fare poorly on the energy intensity performance scale, no smart meter rollout programs seem to be implemented.
Following the European Commission (EC) directive of 2009 (Directive 2009/72/EC), twenty-two EU members will have smart meter deployment programs for electricity and gas by 2020 (see Figure 2). These programs are targeting end-users of energy, e.g. households that represent 29% of the current EU-28’s energy consumption, industries (36.9%) and services (29.8%) (EEA). With this rollout plan, a reduction of 9% in households’ annual energy consumption is expected.
The situation across the member states is however very different. Spain was one of the first EU countries to implement meters in 1988 for industries with demand over 5MW. All the meters will be changed at the end of 2018. 27 million euros for a 30-year investment in smart meter installations is forecasted (EC, 2013). Sweden started to implement smart meter rollout in 2003 and 5.2 million monthly-reading meters were installed by 2009. Vattenfall, one of the major utilities in Sweden, assessed their savings up to 12 euros per installed smart meter (Söderbom, 2012). Similarly in the United Kingdom, the Smart Metering Implementation Programme (SMIP) is estimated to bring an overall £7.2 billion (8.2 billion euros) net benefit over 20 years, mainly from energy saving (OFGEM, 2010). In general, smart metering has been effective, but its effectiveness may diminish over time (Carroll et al, 2014).
From smart-meter to real-time pricing
The idea of real-time pricing for electricity consumers is not new. Borenstein and Holland (2005) and Joskow and Tirole (2006) argue that this price scheme would lead to a more efficient allocation, with lower deadweight loss than under invariant pricing.
By providing detailed information about real-time consumption, smart meters enable energy producers to adopt dynamic pricing strategies. The increasing adoption of smart meters across Europe will likely increase the share of real-time-pricing consumers, as well as the efficiency gains. With the digitalization of the economy, it is likely that smart metering will grow. Indeed, Erdinc (2014) calculates that the economic impact of smart homes on in-home appliances could result in a 33% energy-bill reduction, due to differences in shift potential of appliances.
In 2004, the UK adopted a time-of-use programme called Economy 10, which provides lower tariffs during 10 hours of off-peak periods – split between night, afternoon and evening – for electrically charged and thermal storage heaters. The smart time-of-use tariffs involving daily variation in prices were only introduced in 2017.
Likewise, France’s main electricity provider EDF, implemented Tempo tariff for 350,000 residential customers and more than 100,000 small business customers. Based on a colour system to indicate whether or not the hour is a peak period, customers can automatically or manually monitor their consumption by controlling connection and disconnection of separate water and space-heating circuits. With this program, users reduced their electricity bills by 10% on average.
In Russia, the “consumptions threshold” program discussed by Natalya Volchkova, gave different prices for different consumption thresholds. But it seems that the consumers’ behaviour did not change. This might be due to the thresholds being too low, and an adjusted program should be launched in 2019.
Joskow and Tirole (2007), argue that an optimal electricity demand response program should include some rationing of price-insensitive consumers. Indeed, voluntary interruptible load programs have been launched, mainly targeting energy intensive industries that are consuming energy on a 24/7 basis. These programs consist of rewarding users financially to voluntarily be on standby. For instance, interruptible programmes in Italy apply a lump-sum compensation of 150,000 euros/MWh/year for 10 interruptions and 3000 euros/MW for each additional interruption (Torriti et al., 2010).
Nudging with energy labelling
Energy labelling has been also part of DSM. Since the EC Directives on Ecodesign and Energy Labelling (Directives 2009/125/EC and 2010/30/EU), energy-consuming products should be labelled according to their level of energy efficiency. For Ireland, Eleanor Denny has tested how labelling electrical in-home appliances may affect consumers’ decisions, like purchasing electrical appliances or buying a house. First, Denny and co-authors have nudged buyers of appliances, providing different information regarding future energy bills saving. They find that highly educated people, middle income and landlords are more likely to be concerned with energy-efficiency rates, rather than high-income people.
In another randomized control trial, Denny and co-authors manipulate information on the energy efficiency label for a housing purchase. In Ireland, landlords are charged for energy bills even when they rent out their property. The preliminary findings are that landlords informed about the annual energy cost of their houses are willing to pay 2,608 euros for a one step improvement in the letter rating – the EU label rating for buildings ranges from A to G – compared to the landlords that do not receive the information (see CONSEED project).
Similar to the European Directive, the 2009 Russian Energy efficiency law includes compulsory energy efficiency labels for some goods and improvements of the building standards (EBRD, 2011). Volchkova and co-authors run a randomized controlled experiment on the monetary incentives to buy energy efficient products. In 2016, people in the Moscow region received a voucher with randomly assigned discounts (-30%, -50% or -70%- for the purchase of LED bulbs. Vouchers were used very little, irrespective of the income. It seems that consumption habits and not so much monetary rewards were the main driver of LED bulb purchase.
How can DSM be improved?
Any demand response program requires some demand elasticity. For example, smart meters and dynamic pricing only improve electricity consumption efficiency if demand is price elastic. As Jessoe and Rapson (2014) show, one should provide detailed information (e.g. insights on non-price attributes, real-time feedback on in-home displays) to try to increase demand elasticity. Hence it seems that the low adoption of energy efficient goods is partly due to a lack of information or biased information received by the consumers. First, it is difficult for many to translate energy savings in kWh in monetary terms. Second, many consumers focus on the short-term purchase cost and discount heavily the long run energy saving. These information inefficiencies can, in principle, be diminished by private actors and/or governmental regulation. Denny mentioned the possibility of displaying monetary benefits on labels in consumers’ decision-making in order to improve energy cost salience. For instance, in the US or Japan, the usage cost information is also displayed in monetary terms. Moreover, lifetime usage cost (i.e. cost of ownership) should also be given to the customers since it has been shown that displaying lifetime energy consumption information has significantly higher effect than presenting annual information (Hutton & Wilkie 1980; Kaenzig 2010).
Summing up, DSM programs, including those with a behavioral framework, are an important tool for regulators, households and industries helping to meet emissions reduction targets, significantly decrease demand for energy and use energy more efficiently.
References
- Allcott, Hunt ; Greenstone, Michael. 2012. “Is There an Energy Efficiency Gap?”, Journal of Economic Perspectives, 26 (1): 3-28.
- Borenstein, Severin; Holland, Stephen. 2005. “On The Efficiency Of Competitive Electricity Markets With Time-Invariant Retail Prices”, Rand Journal of Economics, 36(3), 469-493.
- Carroll, James; Lyons, Seán; Denny, Eleanor. 2014. “Reducing household electricity demand through smart metering: The role of improved information about energy saving,” Energy Economics, 45(C), 234-243.
- Denny, Eleanor; O’Malley, Mark. 2010. “Base-load cycling on a system with significant wind penetration”, IEEE Transactions on Power Systems 2.25, 1088-1097.
- Erdinc, Ozan. 2014. “Economic impacts of small-scale own generating and storage units, and electric vehicles under different demand response strategies for smart households”, Applied Energy, 126(C), 142-150.
- European Bank for Reconstruction and Development. “The low carbon transition”. Chapter 3 Effective policies to induce mitigation (2011).
- European Commission. Electricity Directive 2009/92. Annex I.
- European Commission. Ecodesign and Energy Labelling Framework directives 2009/125/EC and 2010/30/EU.
- European Commission. “From Smart Meters to Smart Consumers”, Promoting best practices in innovative smart metering services to the European regions (2013).
- European Commission. “Benchmarking smart metering deployment in the EU-27 with a focus on electricity” (2014).
- European Environment Agency. Data on Final energy consumption of electricity by sector and Energy intensity.
- Frederiks, Elisha R.; Stenner, Karen; Hobman, Elizabeth V. 2015. “Household energy use: Applying behavioural economics to understand consumer decision-making and behaviour”, Renewable and Sustainable Energy Reviews, 41(C), 1385-1394.
- Hutton, Bruce R.; Wilkie, William L. 1980. “Life Cycle Cost: A New Form of Consumer Information.” Journal of Consumer Research, 6(4), 349-60.
- Jessoe, Katrina; Rapson, David. 2014. “Knowledge is (less) power: experimental evidence from residential energy use”, American Economic Review, 104(4), 1417-1438.
- Joskow, Paul; Tirole, Jean. 2006. “Retail Electricity Competition“, Rand Journal of Economics, 37(4), 799-815.
- Joskow, Paul; Tirole, Jean. 2007. “Reliability and Competitive Electricity Markets”, Rand Journal of Economics, 38(1), 60-84.
- Kaenzig, Josef; Wüstenhagen, Rolf. 2010. “The Effect of Life Cycle Cost Information on Consumer Investment Decisions Regarding Eco‐Innovation”, Journal of Industrial Ecology, 14(1), 121-136.
- OFGEM. “Smart Metering Implementation Programme” (2010).
- Söderbom, J. “Smart Meter roll out experiences”, Vattenfall (2012).
- Torriti, Jacopo; Hassan, Mohamed G.; Leach, Matthew. 2010. “Demand response experience in Europe: Policies, programmes and implementation”, Energy, 35(4), 1575-1583.
Project links
Eleanor Denny and co-authors’ European research projects:
- CONSEED (Consumer Energy Efficiency Decision making) https://www.conseedproject.eu/
- NEEPD (Nudging Energy efficient Purchasing Decisions) https://www.neepd.com/
Disclaimer: Opinions expressed in policy briefs and other publications are those of the authors; they do not necessarily reflect those of the FREE Network and its research institutes.
Losers and Winners of Russian Countersanctions: A welfare analysis
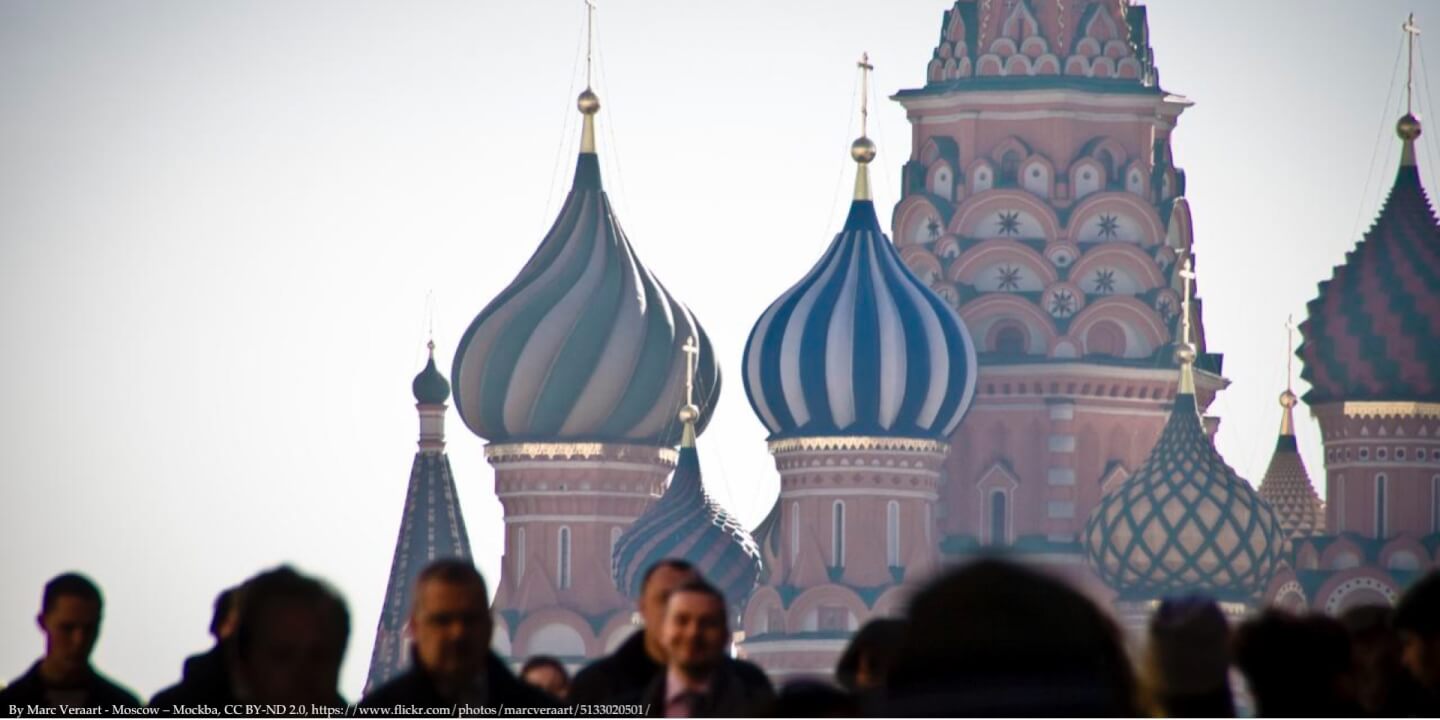
In this brief we provide a quantitative assessment of the consequences of countersanctions introduced by the Russian government in 2014 in response to sectoral restrictive measures initiated by a number of developed countries. Commodity groups that fell under countersanctions included meat, fish, dairy products, fruit and vegetables. By applying a basic partial equilibrium analysis to data from several sources, including Rosstat, Euromonitor, UN Comtrade, industry reviews etc., we obtain that total consumers’ loss due to countersanctions amounts to 288 bn Rub or 2000 rubles per year for each Russian citizen. Producers capture 63% of this amount, importers 26%, while deadweight loss amounts to 10%. 30% of the transfer from Russian consumers toward importers was acquired by Belarus. The gain of Belarusian importers of cheese is especially impressive – 83% of total importer’s gains on the cheese market.
In August 2014, in response to sectoral sanctions initiated by some countries against Russia, the national government issued resolution No. 778, which prohibited import of processed and raw agricultural products from the United States, the EU, Ukraine and a number of other countries (Norway, Canada, Australia, etc.).
Russian countersanctions were, in particular, imposed on meat, fish, dairy products, fruit and vegetables. Later the list of counter sanctioned goods was edited: inputs for the production of baby food and medicines have been deleted from the ban list, while new items were added. Salt was added to the list in November 2016 and animal fats in October 2017.
The popular idea behind the countersanctions was to limit market access for countries, which supported sectoral sanctions. The other rhetoric of the countersanctions was to support domestic producers via trade restrictions, or by other words – import substitution.
We apply a basic partial equilibrium analysis in order to evaluate the effect of countersanctions on the welfare of main stakeholders – consumers, producers and importers. The overall results are in line with general microeconomic consequences of trade restrictions in a small open economy, that is, we observe a decline in consumer surplus, increase in producer surplus and redistribution across importers. Perhaps, even more interestingly, we are able to provide a numerical assessment of redistribution effects between Russian consumers and producers, on the one hand, and among importers from different countries, on the other.
Partial equilibrium welfare analysis
We apply a framework of the classical analysis of import tariff increases to Russian countersanctions. Countersanctions resulted in increased domestic prices, declining consumption and increased domestic production. Given the increase in prices and declined volumes of consumption, we evaluate the losses by consumers as a decline in consumer surplus. Respectively, given the increase in prices and increase in domestic output we identify the producers gains as an increase in producer surplus. The only difference with a classical analysis is the lack of increase in government revenues. In this case increases in domestic prices were driven by restrictions on trade with historical partners which were substituted by more costly producers. Given the changes in the composition of importers after sanctions, we identify countries which lost and gained access to the Russian market. We use changes in volumes of trade as a measure of respective gains and losses. Figure 1 presents all relevant concepts.
In order to measure all relevant welfare changes, we rely on consumption, production and price data from Rosstat and Euromonitor, trade data from the UN Comtrade database. We use data for 2013 as a benchmark before countersanctions and compare it to 2016. The measures of own price elasticities of Russian demand and supply were taken from the literature. We use real price (in terms of 2013 prices) and volume information for consumption and supply in 2016 as the resulting points on the supply (point C) and demand (point A) curves as shown on Figure 1. Then we restore the consumption and production points on these curves (points F and B) as they would have been in 2013 given the own price elasticities of demand and supply and price level as of 2013.
Figure 1. Visualization of deadweight losses, consumer and producer surplus changes
Welfare analysis
Data
We consider 12 commodity groups that were included in 2014 in the countersanctions list: pork, cheese, poultry, apples, beef, tomatoes, processed meat, fromage frais, butter, oranges, condensed milk, grapes, cream, sour milk products, milk, and bananas.
Prices and volumes information are taken from Rosstat official statistics, which in a few cases were adjusted by data from Euromonitor. Import values were obtained from the UN Comtrade database. The summary of the original data and results of welfare analyses are reported in table 1. Below we discuss in details the situation in three markets – beef, apples and cheese.
Table 1. Summary table of the welfare effects of countersanctions
Group | Price (RUR per kg, 2013) | Production (thous. tons) | Consumption (thous. tons) | Elasticity | Consumer losses, RUR mn | Producer surplus, RUR mn | Deadweight loss, RUR mn | Importer gains, RUR mn | ||||
2016 | 2013 | 2016 | 2013 | 2016 | 2013 | demand | supply | |||||
Beef | 376 | 357 | 238 | 240 | 600 | 897 | -0.78 | 0.1 | 11311 | 4388 | 234 | 6690 |
Poultry | 109 | 108 | 4468 | 3610 | 4577 | 4084 | -0.78 | 0.45 | 3263 | 3173 | 13 | 77 |
Pork | 286 | 289 | 2042 | 1299 | 2282 | 1919 | -0.78 | 0.2 | -7167 | -6447 | 38 | -757 |
Milk | 55 | 47 | 5540 | 5386 | 5704 | 5595 | -0.93 | 0.3 | 48234 | 42507 | 4443 | 1284 |
Butter | 343 | 271 | 251 | 225 | 340 | 340 | -0.93 | 0.18 | 27468 | 17680 | 3370 | 6419 |
Cheese | 358 | 283 | 605 | 435 | 748 | 764 | -0.93 | 0.28 | 63493 | 44259 | 8437 | 10797 |
Fromage frais | 233 | 190 | 407 | 371 | 456 | 457 | -0.93 | 0.3 | 21803 | 17104 | 2600 | 2099 |
Apples | 84 | 70 | 324 | 313 | 986 | 1665 | -0.85 | 0.1 | 15225 | 4562 | 1238 | 9425 |
Bananas | 61 | 47 | 0 | 0 | 1141 | 1165 | -0.9 | 0.1 | 18967 | 0 | 2315 | 16652 |
Oranges | 65 | 59 | 0 | 0 | 932 | 1059 | -0.9 | 0.1 | 6054 | 0 | 272 | 5782 |
Grapes | 175 | 131 | 174 | 101 | 366 | 459 | -0.85 | 0.1 | 18312 | 7527 | 2351 | 8435 |
Tomatoes | 82 | 65 | 1130 | 863 | 1583 | 1718 | -0.97 | 0.1 | 28824 | 18177 | 3290 | 7357 |
Data sources: Rosstat, Euromonitor, UN COMTRADE
Bold figures were used to mark the commodity groups with a noticeable consumption growth in 2013-2016, italic figures – for those with consumption decrease, and underlined – for groups where consumption changed insignificantly during the period.
Beef
The Russian beef market experienced a drastic decrease in consumption during two years under countersanctions. In 2013 constant prices, the average real of 1 kg of beef increased by 5.3% from 357 Rub/kg in 2013 up to 376 Rub/kg in 2016. Domestic output decreased by 0.8% and to 238 thousand tons in 2016 from 240 in 2013. Domestic consumption decreased by 33.1% to 600 thousand tons in 2016 from 897 in 2013. Our estimations indicate that consumer losses amount to 11.3 bn Rub or 3.5% of beef consumption in 2013; producers’ gains are 4.4 bn Rub or 1.4%; deadweight losses are estimated at 0.2 bn Rub or 0.07%; and importers’ gains equal 6.7 bn Rub or 2.1%.
Out of total 6.7 bn Rub of importers’ gains, importers from Belarus acquire the major share (88%) – 5.9 bn Rub. Importers of beef from India and Colombia gained 0.4 bn Rub (6% of total) and 0.3 bn Rub (5%) respectively. Beef importers from Mongolia gained 0.03 bn Rub, from Kazakhstan – 0.01 bn Rub. Importers of beef from Brazil, Paraguay, Australia, Uruguay, Ukraine, Lithuania, Poland, and Argentina lost market shares in over the period 2013-2016.
Cheese
Average real price for 1 kg of cheese increased by 26.5% up to 358 Rub/kg in 2016 from 283 Rub/kg in 2013, both in constant 2013 prices. Domestic output increased by 39.1% to 605 thousand tons in 2016 from 435 thous. tons in 2013. Domestic consumption decreased by 2.1% to 748 thous. tons in 2016 from 764 thous. tons in 2013. Our results indicate the following effects of countersanctions on cheese market: consumers’ losses amounted to 63.5 bn Rub or 29.4% of cheese consumption in 2013; producer’s gain is 44.3 bn Rub or 20.5%; deadweight loss is estimated at 8.4 bn Rub or 3.9%; importers’ gains equal 10.8 bn Rub or 5.0%.
Out of a total 10.8 bn Rub of importer’s gains on the cheese market, importers of cheese from Belarus acquired the major share (82.9%) – 9.0 bln Rub, importers of cheese from Argentina gained 0.5 bn Rub (4.8% of total importers’ gain), importers from Uruguay gained 0.4 bn Rub (3.9%), Swiss cheese importers gained 0.2 bn Rub, importers from Armenia – 0.2 bn Rub (1.8%). While importers of cheese from Ukraine, the Netherlands, Germany, Finland, Poland, Lithuania, France, Denmark, Italy, and Estonia lost market access over 2013-2016.
Apples
In 2013 constant prices, average real price for 1 kg of apples increased by 20.0% up to 84 Rub/kg in 2016 from 70 Rub/kg in 2013. Domestic output increased by 3.5% to 324 thous. tons in 2016 from 313 thous. tons in 2013. Domestic consumption decreased by 40.8% to 986 thous. tons in 2016 from 1665 thous. tons in 2013. According to our analysis, the effects of countersanctions on the apple market are the following: consumers’ losses amounted to 15.2 bn Rub or 13.1 of apple consumption in 2013; producer’s gain is 4.6 bn Rub or 3.0%; deadweight loss is estimated at 1.2 bn Rub or 1.1%; importers’ gains equal 9.4 bln Rub or 8.1%.
Out of a total 9.4 bn Rub of importer’s gains, importers from Serbia acquired the major share (49.7%) – 4,7 bn Rub, importers of apples from China gained 1.6 bn Rub (16.7% of total importers’ gains), those importing from Macedonia gained 0.8 bn Rub (8.4%), from Azerbaijan 0.6 bn Rub (6.0%), and from South Africa 0.4 bn Rub (4.5% of total importers’ gains). While importers of apples from Poland, Italy, Belgium, and France lost market access.
Overall effects for 12 commodity groups
We calculated the welfare effects for 12 commodity groups: beef, poultry, milk, cheese, cottage cheese, ton butter, dairy products, apples, bananas, oranges, grapes and tomatoes.
Total consumers’ loss due to countersanctions amounts to 288 bn Rub, producers gain 63% out of this amount (182 bn Rub), 26% of total consumers’ loss is redistributed to importers (75 bn Rub), deadweight losses amount to 10% (31 bn Rub).
Distribution of importers’ gains
Belarus is the major beneficiary of Russians countersanctions: its exporters gain 29.4 bn Rub (38%), Ecuador’s exporters are in the second place with 16.4 bln Rub (21). Exporters from Serbia gained 5.1 bn Rub (7%).
Conclusion
There is no doubt that countersanctions were paid out of the pockets of Russian consumers: our estimation of total consumer losses amounts to 288 billion rubles, i.e. each Russian citizen paid 2000 rubles per year. Out of this sum, Russian producers received 144 billion rubles, i.e. transfer from Russian consumers to producers equals 1260 rubles per person per year. Among Russian sectors, major gains and associated increases in production happened in pork industries (50%), poultry (20%), dairy products (10-30%), fruit and vegetables (10-50%).
The transfer from Russian consumers toward importers from non-sanctioned countries equals 75 billion rubles a year (520 rubles per person per year), out of which 30% was acquired by Belarusian importers. Countersanctions lead to deadweight losses in the efficiency of Russian economy equal to 31 billion rubles or 215 rubles per person per year.
Disclaimer: Opinions expressed in policy briefs and other publications are those of the authors; they do not necessarily reflect those of the FREE Network and its research institutes.